Neuro-degenerative disease Identification using MRI 3D-Convolution Method
Suprava Saha1 , Deepika Das2 , Aditya Kumar Singh3 , Sabbir Reza Tarafdar4 , Tushnik Sarkar5
Section:Research Paper, Product Type: Journal Paper
Volume-11 ,
Issue-01 , Page no. 190-196, Nov-2023
Online published on Nov 30, 2023
Copyright © Suprava Saha, Deepika Das, Aditya Kumar Singh, Sabbir Reza Tarafdar, Tushnik Sarkar . This is an open access article distributed under the Creative Commons Attribution License, which permits unrestricted use, distribution, and reproduction in any medium, provided the original work is properly cited.
View this paper at Google Scholar | DPI Digital Library
How to Cite this Paper
- IEEE Citation
- MLA Citation
- APA Citation
- BibTex Citation
- RIS Citation
IEEE Style Citation: Suprava Saha, Deepika Das, Aditya Kumar Singh, Sabbir Reza Tarafdar, Tushnik Sarkar, “Neuro-degenerative disease Identification using MRI 3D-Convolution Method,” International Journal of Computer Sciences and Engineering, Vol.11, Issue.01, pp.190-196, 2023.
MLA Style Citation: Suprava Saha, Deepika Das, Aditya Kumar Singh, Sabbir Reza Tarafdar, Tushnik Sarkar "Neuro-degenerative disease Identification using MRI 3D-Convolution Method." International Journal of Computer Sciences and Engineering 11.01 (2023): 190-196.
APA Style Citation: Suprava Saha, Deepika Das, Aditya Kumar Singh, Sabbir Reza Tarafdar, Tushnik Sarkar, (2023). Neuro-degenerative disease Identification using MRI 3D-Convolution Method. International Journal of Computer Sciences and Engineering, 11(01), 190-196.
BibTex Style Citation:
@article{Saha_2023,
author = {Suprava Saha, Deepika Das, Aditya Kumar Singh, Sabbir Reza Tarafdar, Tushnik Sarkar},
title = {Neuro-degenerative disease Identification using MRI 3D-Convolution Method},
journal = {International Journal of Computer Sciences and Engineering},
issue_date = {11 2023},
volume = {11},
Issue = {01},
month = {11},
year = {2023},
issn = {2347-2693},
pages = {190-196},
url = {https://www.ijcseonline.org/full_spl_paper_view.php?paper_id=1432},
publisher = {IJCSE, Indore, INDIA},
}
RIS Style Citation:
TY - JOUR
UR - https://www.ijcseonline.org/full_spl_paper_view.php?paper_id=1432
TI - Neuro-degenerative disease Identification using MRI 3D-Convolution Method
T2 - International Journal of Computer Sciences and Engineering
AU - Suprava Saha, Deepika Das, Aditya Kumar Singh, Sabbir Reza Tarafdar, Tushnik Sarkar
PY - 2023
DA - 2023/11/30
PB - IJCSE, Indore, INDIA
SP - 190-196
IS - 01
VL - 11
SN - 2347-2693
ER -
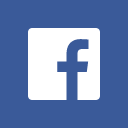
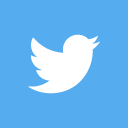
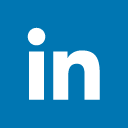
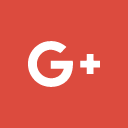
Abstract
Alzheimer`s disease (AD) is a perpetual neurological disorder primarily affecting the brain leading to cognitive deterioration, behavioral problems, and memory loss. Alzheimer’s disease which poses a significant danger to individuals worldwide in accordance with the World Health Organization (WHO). According to a recent study, by the year 2060, 70% of the population would have this condition. With a prevalence rate of 60 to 80 percent among dementia cases globally, Alzheimer`s disease emerges as the leading etiology. Researchers are diligently working on the development of advanced machine learning models to improve the accuracy of skull stripping, specifically for separating neural tissues from non-neural tissue in magnetic resonance imaging (MRI) scans and identifying affected patients. In this paper, we unveil a fresh perspective of modified 3D-UNet architecture for precise brain segmentation and 3D-CNN architecture for classification. We argue for a volumetric analysis of the whole brain instead of localization and context information-based approaches for disease classification. As the dataset possesses the time-series like nature, utilization of the long short-term memory-based LSTM architecture has been utilized for medical analysis using MRI data from multiple regular patients. It enhances disease diagnosis & treatment effectiveness. The proposed approach demonstrates segmentation accuracy of 97% and classification accuracy of 95%. These findings enlighten the potential of LSTM-based analysis for neuro-degenerative diseases like-AD.
Key-Words / Index Term
Brain Segmentation, 3D U-Net, Disease Classification, 3D CNN, Alzheimer’s disease, MRI, LSTM
References
[1] Chiyu Feng, Ahmed Elazab, Peng Yang, Tianfu Wang, Feng Zhou, Huoyou Hu, Xiaohua Xiao, and Baiying Lei. Deep learning framework for alzheimer’s disease diagnosis via 3d-cnn and fsbi-lstm. IEEE Access, 7: pp.63605– 63618, 2019.
[2] Zahra Asefy, Sirus Hoseinnejhad, and Zaker Ceferov. Nanoparticles approaches in neurodegenerative diseases diagnosis and treatment. Neurological Sciences, 42: pp.2653–2660, 2021.
[3] Zahraa Sh Aaraji and Hawraa H Abbas. Automatic classification of alzheimer’s disease using brain mri data and deep convolutional neural networks. arXiv preprint arXiv:2204.00068, 2022.
[4] Philip Scheltens, Kaj Blennow, Monique MB Breteler, Bart De Strooper, Giovanni B Frisoni, Stephen Salloway, and Wiesje Maria Van der Flier. Alzheimer’s disease. The Lancet, 388(10043): pp.505–517, 2016.
[5] Marcus E Raichle. Functional brain imaging and human brain function. Journal of Neuroscience, 23(10): pp.3959– 3962, 2003.
[6] Renaud Jardri, V´eronique Houfflin-Debarge, Pierre Delion, Jean-Pierre Pruvo, Pierre Thomas, and Delphine Pins. Assessing fetal response to maternal speech using a noninvasive functional brain imaging technique. International Journal of Developmental Neuroscience, 30(2): pp.159–161, 2012.
[7] Srinivasan Aruchamy, Amrita Haridasan, Ankit Verma, Partha Bhattacharjee, Sambhu Nath Nandy, and Siva Ram Krishna Vadali. Alzheimer’s disease detection using machine learning techniques in 3d mr images. In 2020 National Conference on Emerging Trends on Sustainable Technology and Engineering Applications (NCETSTEA), pages 1–4. IEEE, 2020.
[8] William W Orrison, Jeffrey Lewine, John Sanders, and Michael F Hartshorne. Functional brain imaging. Elsevier Health Sciences, 2017.
[9] Shaswati Roy and Pradipta Maji. A simple skull stripping algorithm for brain mri. In 2015 Eighth International Conference on Advances in Pattern Recognition (ICAPR), pages 1–6. IEEE, 2015.
[10] Farahnaz Hosseini, Hossein Ebrahimpourkomleh, and Mehrnaz KhodamHazrati. Quantitative evaluation of skull stripping techniques on magnetic resonance images. In World Congress on Electrical Engineering and Computer Systems and Science (EECSS 2015), 2015.
[11] Simon F Eskildsen, Pierrick Coup´e, Vladimir Fonov, Jos´e V Manj´on, Kelvin K Leung, Nicolas Guizard, Shafik N Wassef, Lasse Riis Ostergaard, D Louis Collins, Alzheimer’s Disease Neuroimaging Initiative, et al. Beast: brain extraction based on nonlocal segmentation technique. NeuroImage, 59(3): pp.2362–2373, 2012.
[12] MC Metzger, G Bittermann, L Dannenberg, Rainer Schmelzeisen, N-C Gellrich, Bettina Hohlweg-Majert, and C Scheifele. Design and development of a virtual anatomic atlas of the human skull for automatic segmentation in computer-assisted surgery, preoperative planning, and navigation. International journal of computer assisted radiology and surgery, 8(5): pp.691–702, 2013.
[13] Konstantin Levinski, Alexei Sourin, and Vitali Zagorodnov. Interactive surface-guided segmentation of brain mri data. Computers in Biology and Medicine, 39(12): pp.1153– 1160, 2009.
[14] Hiroyuki Sugimori and Masashi Kawakami. Automatic detection of a standard line for brain magnetic resonance imaging using deep learning. Applied Sciences, 9(18): pp.38-49, 2019.
[15] Liang Zou, Jiannan Zheng, Chunyan Miao, Martin J
Mckeown, and Z Jane Wang. 3d cnn based automatic
diagnosis of attention deficit hyperactivity disorder using functional and structural mri. Ieee Access, 5: pp.23626–
23636, 2017.
[16] Daniel Maturana and Sebastian Scherer. Voxnet: A 3d convolutional neural network for real-time object recognition. In 2015 IEEE/RSJ international conference on intelligent robots and systems (IROS), pages 922–928. IEEE, 2015.
[17] Linmin Pei, Murat Ak, Nourel Hoda M Tahon, Serafettin Zenkin, Safa Alkarawi, Abdallah Kamal, Mahir Yilmaz, Lingling Chen, Mehmet Er, Nursima Ak, et al. A general skull stripping of multiparametric brain mris using 3d convolutional neural network. Scientific Reports, 12(1):10826, 2022.
[18] Carlos Paredes-Orta, Jorge Domingo Mendiola- Santiba˜nez, Danjela Ibrahimi, Juvenal Rodr´?guez- Res´endiz, Germ´an D´?az-Florez, and Carlos Alberto Olvera-Olvera. Hyperconnected openings codified in a max tree structure: An application for skull-stripping in brain mri t1. Sensors, 22(4):1378, 2022.
[19] Gabriele Valvano, Nicola Martini, Andrea Leo, Gianmarco Santini, Daniele Della Latta, Emiliano Ricciardi, and Dante Chiappino. Training of a skull-stripping neural network with efficient data augmentation. arXiv preprint arXiv:1810.10853, 2018.
[20] Zijian Wang, Yaoru Sun, Qianzi Shen, and Lei Cao. Dilated 3d convolutional neural networks for brain mri data classification. Ieee Access, 7: pp.134388–134398, 2019.
[21] Xingang Liu, Yukun Duan, and Bin Liu. Nanoparticles as contrast agents for photoacoustic brain imaging. Aggregate, 2(1): pp.4–19, 2021.
[22] Luke Clark and Barbara J Sahakian. Cognitive neuroscience and brain imaging in bipolar disorder. Dialogues in clinical neuroscience, 2022.
[23] P Kalavathi and VB Surya Prasath. Methods on skull stripping of mri head scan images—a review. Journal of digital imaging, 29: pp.365–379, 2016.
[24] Martin Hofmann-Apitius, Gordon Ball, Stephan Gebel, Shweta Bagewadi, Bernard De Bono, Reinhard Schneider, Matt Page, Alpha Tom Kodamullil, Erfan Younesi, Christian Ebeling, et al. Bioinformatics mining and modelling methods for the identification of disease mechanisms in neurodegenerative disorders. International journal of molecular sciences, 16(12): pp.29179–29206, 2015.
[25] Qihua Li, Hongmin Bai, Yinsheng Chen, Qiuchang Sun, Lei Liu, Sijie Zhou, Guoliang Wang, Chaofeng Liang, and Zhi-Cheng Li. A fully-automatic multiparametric radiomics model: towards reproducible and prognostic imaging signature for prediction of overall survival in glioblastoma multiforme. Scientific reports, 7(1):14331, 2017.
[26] Polina Druzhinina and Ekaterina Kondrateva. The effect of skull-stripping on transfer learning for 3d mri models: Adni data. In Medical Imaging with Deep Learning, 2022.
[27] Benoit Dufumier, Pietro Gori, Julie Victor, Antoine Grigis, Michele Wessa, Paolo Brambilla, Pauline Favre, Mircea Polosan, Colm Mcdonald, Camille Marie Piguet, et al. Contrastive learning with continuous proxy meta-data for 3d mri classification. In Medical Image Computing and Computer Assisted Intervention– MICCAI 2021: 24th International Conference, Strasbourg, France, September 27–October 1, 2021, Proceedings, Part II 24, pages 58–68. Springer, 2021.
[28] Sreevani Katabathula, Qinyong Wang, and Rong Xu. Predict alzheimer’s disease using hippocampus mri data: a lightweight 3d deep convolutional network model with visual and global shape representations. Alzheimer’s Research & Therapy, 13(1):1–9, 2021.
[29] Yechong Huang, Jiahang Xu, Yuncheng Zhou, Tong Tong, Xiahai Zhuang, and Alzheimer’s Disease Neuroimaging Initiative (ADNI). Diagnosis of alzheimer’s disease via multi-modality 3d convolutional neural network. Frontiers in neuroscience, 13:509, 2019.
[30] Kamran Kazemi and Negar Noorizadeh. Quantitative comparison of spm, fsl, and brainsuite for brain mr image segmentation. Journal of Biomedical Physics and Engineering, 4(1), 2014.
[31] Hyunho Hwang, Hafiz Zia Ur Rehman, and Sungon Lee. 3d u-net for skull stripping in brain mri. Applied Sciences, 9(3):569, 2019.
[32] Yue Liu, Yuankai Huo, Blake Dewey, Ying Wei, Ilwoo Lyu, and Bennett A Landman. Generalizing deep learning brain segmentation for skull removal and intracranial measurements. Magnetic resonance imaging, 88:44–52, 2022.
[33] Modupe Odusami, Rytis Maskeli?unas, Robertas Dama?sevi?cius, and Tomas Krilavi?cius. Analysis of features of alzheimer’s disease: detection of early stage from functional brain changes in magnetic resonance images using a finetuned resnet18 network. Diagnostics, 11(6):1071, 2021.
[34] SUNITA M Kulkarni and G Sundari. Comparative analysis of performance of deep cnn based framework for brain mri classification using transfer learning. Journal of Engineering Science and Technology, 16(4):2901–2917, 2021.
[35] Nitika Goenka and Shamik Tiwari. Alzvnet: A volumetric convolutional neural network for multiclass classification of alzheimer’s disease through multiple neuroimaging computational approaches. Biomedical Signal Processing and Control, 74:103500, 2022.
[36] Andrew Hoopes, Jocelyn S Mora, Adrian V Dalca, Bruce Fischl, and Malte Hoffmann. Synthstrip: skull-stripping for any brain image. NeuroImage, 260:119474, 2022.
[37] Riccardo De Feo, Artem Shatillo, Alejandra Sierra, Juan Miguel Valverde, Olli Gr¨ohn, Federico Giove, and Jussi Tohka. Automated joint skull-stripping and segmentation with multi-task u-net in large mouse brain mri databases. NeuroImage, 229:117734, 2021.
[38] C? a?gatay Berke Erdas?, Emre S¨umer, and Seda Kibaro?glu. Neurodegenerative disease detection and severity prediction using deep learning approaches. Biomedical Signal Processing and Control, 70:103069, 2021.
[39] Maria Agnese Pirozzi, Mario Tranfa, Mario Tortora, Roberta Lanzillo, Vincenzo Brescia Morra, Arturo Brunetti, Bruno Alfano, and Mario Quarantelli. A polynomial regression-based approach to estimate relaxation rate maps suitable for multiparametric segmentation of clinical brain mri studies in multiple sclerosis. Computer Methods and Programs in Biomedicine, 223:106957, 2022.
[40] Rajni Maurya and Sulochana Wadhwani. Morphology based brain tumor identification and segmentation in mr images. In 2021 IEEE Bombay Section Signature Conference (IBSSC), pages 1–6. IEEE, 2021.
[41] Erico Tjoa and Cuntai Guan. A survey on explainable artificial intelligence (xai): Toward medical xai. IEEE transactions on neural networks and learning systems, 32(11): pp.4793–4813, 2020.
[42] Gopal S Tandel, Antonella Balestrieri, Tanay Jujaray, Narender N Khanna, Luca Saba, and Jasjit S Suri. Multiclass magnetic resonance imaging brain tumor classification using artificial intelligence paradigm. Computers in Biology and Medicine, 122:103804, 2020.
[43] Clifford R Jack Jr, Matt A Bernstein, Nick C Fox, Paul Thompson, Gene Alexander, Danielle Harvey, Bret Borowski, Paula J Britson, Jennifer L. Whitwell, Chadwick Ward, et al. The alzheimer’s disease neuroimaging initiative (adni): Mri methods. Journal of Magnetic Resonance Imaging: An Official Journal of the International Society for Magnetic Resonance in Medicine, 27(4): pp.685– 691, 2008.
[44] Jaeyong Kang, Zahid Ullah, and Jeonghwan Gwak. Mri-based brain tumor classification using ensemble of deep features and machine learning classifiers. Sensors, 21(6):2222, 2021.
[45] Alperen Derin, Ahmet Furkan Bayram, Caglar Gurkan, Abdulkadir Budak, and Hakan KARATAS?. Automatic skull stripping and brain segmentation with u-net in mri database. Avrupa Bilim ve Teknoloji Dergisi, (40): pp.75–81, 2022.
[46] Florent S´egonne, Anders M Dale, Evelina Busa, Maureen Glessner, David Salat, Horst Karl Hahn, and Bruce Fischl. A hybrid approach to the skull stripping problem in mri. Neuroimage, 22(3):pp.1060–1075, 2004.
[47] Hafiz Zia Ur Rehman, Hyunho Hwang, and Sungon Lee. Conventional and deep learning methods for skull stripping in brain mri. Applied Sciences, 10(5):1773, 2020.