Handling Imbalanced Heart Disease Data and Explaining the Factors
Sandip Das1 , Gairik Sajjan2 , Arkajyoti Poddar3 , Tamojit Dasgupta4 , Sayani Patty5 , Debmitra Ghosh6
Section:Research Paper, Product Type: Journal Paper
Volume-11 ,
Issue-01 , Page no. 62-65, Nov-2023
Online published on Nov 30, 2023
Copyright © Sandip Das, Gairik Sajjan, Arkajyoti Poddar, Tamojit Dasgupta, Sayani Patty, Debmitra Ghosh . This is an open access article distributed under the Creative Commons Attribution License, which permits unrestricted use, distribution, and reproduction in any medium, provided the original work is properly cited.
View this paper at Google Scholar | DPI Digital Library
How to Cite this Paper
- IEEE Citation
- MLA Citation
- APA Citation
- BibTex Citation
- RIS Citation
IEEE Style Citation: Sandip Das, Gairik Sajjan, Arkajyoti Poddar, Tamojit Dasgupta, Sayani Patty, Debmitra Ghosh, “Handling Imbalanced Heart Disease Data and Explaining the Factors,” International Journal of Computer Sciences and Engineering, Vol.11, Issue.01, pp.62-65, 2023.
MLA Style Citation: Sandip Das, Gairik Sajjan, Arkajyoti Poddar, Tamojit Dasgupta, Sayani Patty, Debmitra Ghosh "Handling Imbalanced Heart Disease Data and Explaining the Factors." International Journal of Computer Sciences and Engineering 11.01 (2023): 62-65.
APA Style Citation: Sandip Das, Gairik Sajjan, Arkajyoti Poddar, Tamojit Dasgupta, Sayani Patty, Debmitra Ghosh, (2023). Handling Imbalanced Heart Disease Data and Explaining the Factors. International Journal of Computer Sciences and Engineering, 11(01), 62-65.
BibTex Style Citation:
@article{Das_2023,
author = {Sandip Das, Gairik Sajjan, Arkajyoti Poddar, Tamojit Dasgupta, Sayani Patty, Debmitra Ghosh},
title = {Handling Imbalanced Heart Disease Data and Explaining the Factors},
journal = {International Journal of Computer Sciences and Engineering},
issue_date = {11 2023},
volume = {11},
Issue = {01},
month = {11},
year = {2023},
issn = {2347-2693},
pages = {62-65},
url = {https://www.ijcseonline.org/full_spl_paper_view.php?paper_id=1413},
publisher = {IJCSE, Indore, INDIA},
}
RIS Style Citation:
TY - JOUR
UR - https://www.ijcseonline.org/full_spl_paper_view.php?paper_id=1413
TI - Handling Imbalanced Heart Disease Data and Explaining the Factors
T2 - International Journal of Computer Sciences and Engineering
AU - Sandip Das, Gairik Sajjan, Arkajyoti Poddar, Tamojit Dasgupta, Sayani Patty, Debmitra Ghosh
PY - 2023
DA - 2023/11/30
PB - IJCSE, Indore, INDIA
SP - 62-65
IS - 01
VL - 11
SN - 2347-2693
ER -
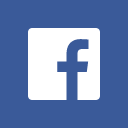
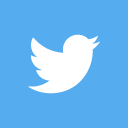
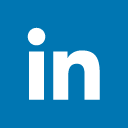
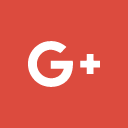
Abstract
Heart disease is one of the most serious and life threatening problems. If predicted beforehand, many lives can be saved. But, the problem is that medical datasets are highly imbalanced, which leads machine learning algorithms to perform poorly on the minority class. Which in terms leads to wrong predictions. In healthcare it is highly risky to predict something wrongly, because, people’s lives are on stake. The ratio of minority and majority class data should be 1:1, or near about equal, in order to get a good result. Synthetic Minority Oversampling TEchnique(SMOTE) is one such oversampling technique that makes it come true, which is used in this work. In addition we have used eXplainable AI(XAI) to better visualise the predictions. We have used LIME (Local Interpretable Model-agnostic Explanation) and SHAP (Shapely Additive Explanations) algorithms to understand the contributions of features towards the predictions.
Key-Words / Index Term
Heart Disease, SMOTE, Machine Learning, Explainable AI, LIME, SHAP
References
[1] Deldar, K., Mahdavi, M., & Mohammadzadeh, N. (2020). Handling imbalanced healthcare data with supervised and unsupervised methods: A systematic literature review. Journal of biomedical informatics, 109, 103516.
[2] Alshammari, R., & Bahsoon, R. (2019). Handling imbalanced data in healthcare: A systematic review. ACM Computing Surveys (CSUR), Vol.52, Issue.5, pp.1-38, 2019.
[3] Wang, S., Yao, J., Hu, Y., Zhao, L., & Zhang, Y. (2020). Addressing imbalanced datasets in medical image analysis. IEEE Transactions on Medical Imaging, Vol.39, Issue.7, pp.2408-2418, 2020.
[4] Al-Bahrani, R., Huang, W., & El-Sheimy, N. (2019). imbalanced healthcare data using ensemble methods and data sampling techniques. Applied Sciences, Vol.9, Issue.13, 2721, 2019.
[5] https://www.cdc.gov/heartdisease/facts.htm [DATASET]
[6] Wang, H., Yang, X., & Zhang, Q. (2019). A deep learning framework for handling imbalanced medical data. IEEE Access, 7, 89154-89162.
[7] Yao, J., Wang, S., Li, W., & Zhang, Y. (2020). Handling imbalanced electronic health record data using convolutional neural networks with auxiliary training. Journal of biomedical informatics, 110, 103530.
[8] L.H. Yang, J. Liu, Y.M.Wang, L. Martínez, A micro-extended belief rule-based system for big data multiclass classification problems, IEEE Trans. Syst. Man Cybern. Syst. pp.1–21, 2018.
[9] P.V. Ngoc, C.V.T. Ngoc, T.V.T. Ngoc, D.N. Duy. A C4. 5 algorithm for english emotional classification, Evolving Syst. 10, pp.425–451, 2019.
[10] Datta, Shounak, and Swagatam Das.Near-Bayesian Support Vector Machines forImbalanced Data Classi?cation with Equal or Unequal Misclassi?cation Costs. NeuralNetworks 70: pp.39–52, 2015.
[11] ahajournals.org/doi/full/10.1161/CIRCULATIONAHA.114.008729