An Enhanced Intrusion Detection System Using Edge Centric Approach
Bhavya Lahari Vaddempudi1 , Amrutha Tulabandu2 , S.N.B. Tanuja Reddy3 , Deepika Leela Pudi4 , Venkata Narayana Yerininti5
- Dept. of Information Technology, Vasireddy Venkatadri Institute of Technology, Guntur, India.
- Dept. of Information Technology, Vasireddy Venkatadri Institute of Technology, Guntur, India.
- Dept. of Information Technology, Vasireddy Venkatadri Institute of Technology, Guntur, India.
- Dept. of Information Technology, Vasireddy Venkatadri Institute of Technology, Guntur, India.
- Dept. of Information Technology, Vasireddy Venkatadri Institute of Technology, Guntur, India.
Section:Research Paper, Product Type: Journal Paper
Volume-12 ,
Issue-4 , Page no. 12-16, Apr-2024
CrossRef-DOI: https://doi.org/10.26438/ijcse/v12i4.1216
Online published on Apr 30, 2024
Copyright © Bhavya Lahari Vaddempudi, Amrutha Tulabandu, S.N.B. Tanuja Reddy, Deepika Leela Pudi, Venkata Narayana Yerininti . This is an open access article distributed under the Creative Commons Attribution License, which permits unrestricted use, distribution, and reproduction in any medium, provided the original work is properly cited.
View this paper at Google Scholar | DPI Digital Library
How to Cite this Paper
- IEEE Citation
- MLA Citation
- APA Citation
- BibTex Citation
- RIS Citation
IEEE Style Citation: Bhavya Lahari Vaddempudi, Amrutha Tulabandu, S.N.B. Tanuja Reddy, Deepika Leela Pudi, Venkata Narayana Yerininti, “An Enhanced Intrusion Detection System Using Edge Centric Approach,” International Journal of Computer Sciences and Engineering, Vol.12, Issue.4, pp.12-16, 2024.
MLA Style Citation: Bhavya Lahari Vaddempudi, Amrutha Tulabandu, S.N.B. Tanuja Reddy, Deepika Leela Pudi, Venkata Narayana Yerininti "An Enhanced Intrusion Detection System Using Edge Centric Approach." International Journal of Computer Sciences and Engineering 12.4 (2024): 12-16.
APA Style Citation: Bhavya Lahari Vaddempudi, Amrutha Tulabandu, S.N.B. Tanuja Reddy, Deepika Leela Pudi, Venkata Narayana Yerininti, (2024). An Enhanced Intrusion Detection System Using Edge Centric Approach. International Journal of Computer Sciences and Engineering, 12(4), 12-16.
BibTex Style Citation:
@article{Vaddempudi_2024,
author = {Bhavya Lahari Vaddempudi, Amrutha Tulabandu, S.N.B. Tanuja Reddy, Deepika Leela Pudi, Venkata Narayana Yerininti},
title = {An Enhanced Intrusion Detection System Using Edge Centric Approach},
journal = {International Journal of Computer Sciences and Engineering},
issue_date = {4 2024},
volume = {12},
Issue = {4},
month = {4},
year = {2024},
issn = {2347-2693},
pages = {12-16},
url = {https://www.ijcseonline.org/full_paper_view.php?paper_id=5673},
doi = {https://doi.org/10.26438/ijcse/v12i4.1216}
publisher = {IJCSE, Indore, INDIA},
}
RIS Style Citation:
TY - JOUR
DO = {https://doi.org/10.26438/ijcse/v12i4.1216}
UR - https://www.ijcseonline.org/full_paper_view.php?paper_id=5673
TI - An Enhanced Intrusion Detection System Using Edge Centric Approach
T2 - International Journal of Computer Sciences and Engineering
AU - Bhavya Lahari Vaddempudi, Amrutha Tulabandu, S.N.B. Tanuja Reddy, Deepika Leela Pudi, Venkata Narayana Yerininti
PY - 2024
DA - 2024/04/30
PB - IJCSE, Indore, INDIA
SP - 12-16
IS - 4
VL - 12
SN - 2347-2693
ER -
![]() |
![]() |
![]() |
11 | 31 downloads | 7 downloads |
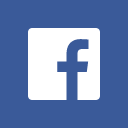
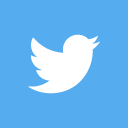
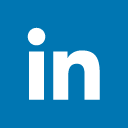
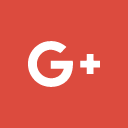
Abstract
In the ever-evolving landscape of Cybersecurity, the detection and mitigation of network intrusions and anomalous activities remain formidable challenges. Conventional methods for identifying threats often encounter difficulties in scaling up and adapting swiftly, as they heavily rely on labeled network data. Furthermore, a narrow focus on individual data points may inadvertently overlook critical details at the packet level, thus exposing vulnerabilities that malicious actors can exploit. To confront these ongoing challenges head-on, Graph Neural Networks (GNNs) emerge as a promising solution. Their innate ability to comprehend complex network structures equips them with the capability to provide deeper insights into the dynamics of network traffic. By harnessing the power of GNN, it autonomously detects and comprehends intrusions and anomalies, surpassing the limitations of conventional techniques. Through experimentation and evaluation on real-world datasets, the proposed system demonstrates promising results in accurately identifying and classifying network intrusions.
Key-Words / Index Term
Cyber Security, Network intrusions, Graph Neural Networks (GNN), Packet level analysis, Performance metrics, Effectiveness Evaluation, Bot-Iot.
References
[1] A. R. Mathew, A. Al Hajj and K. Al Ruqeishi, "Cyber crimes: Threats and protection," 2010 International Conference on Networking and Information Technology, Manila, Philippines, pp.16-18, 2010. doi: 10.1109/ICNIT.2010.5508568.
[2] N. Aggarwal, M. Sehgal and A. Arya, "An empirical analysis of Cyber Crimes, their prevention measures, and laws in India," 2022 Seventh International Conference on Parallel, Distributed and Grid Computing (PDGC), Solan, Himachal Pradesh, India, pp.570-575, 2022. doi: 10.1109/PDGC56933.2022.10053354.
[3] Anupreet Kaur Mokha. A Study on Awareness of Cyber Crime and Security. Research J. Humanities and Social Sciences. October -December, Vol.8, Issue.4, pp.459-464, 2017. doi: 10.5958/2321-5828.2017.00067.5
[4] S. Angra and S. Ahuja, "Machine learning and its applications: A review," 2017 International Conference on Big Data Analytics and Computational Intelligence (ICBDAC), Chirala, Andhra Pradesh, India, pp.57-60, 2017. doi: 10.1109/ICBDACI.2017.8070809.
[5] D. Kataria et al., "Artificial Intelligence And Machine Learning," 2022 IEEE Future Networks World Forum (FNWF), Montreal, QC, Canada, pp.1-70, 2022. doi: 10.1109/FNWF55208.2022.00133.
[6] M. Gorricho-Segura, X. Echeberria-Barrio and L. Segurola-Gil, "Edge-based Analysis for Network Intrusion Detection using a GNN Approach," 2023 JNIC Cybersecurity Conference (JNIC), Vigo, Spain, pp.1-7, 2023. doi: 10.23919/JNIC58574.2023.10205384.
[7] L. Zeng, C. Yang, P. Huang, Z. Zhou, S. Yu and X. Chen, "GNN at the Edge: Cost-Efficient Graph Neural Network Processing Over Distributed Edge Servers," in IEEE Journal on Selected Areas in Communications, March, Vol.41, No.3, pp.720-739, 2023. doi: 10.1109/JSAC.2022.3229422.
[8] F. Scarselli, M. Gori, A. C. Tsoi, M. Hagenbuchner and G. Monfardini, "The Graph Neural Network Model," in IEEE Transactions on Neural Networks, Jan., Vol.20, No.1, pp.61-80, 2009. doi: 10.1109/TNN.2008.2005605.
[9] M. Schneider, J. Weißenbach and U. Schmid, "Substrate Temperature and Bias Voltage Dependent Properties of Sputtered AlN Thin Films for BAW Applications," 2018 IEEE 18th International Conference on Nanotechnology (IEEE-NANO), Cork, Ireland, pp.420-425, 2018. doi: 10.1109/NANO.2018.8626332.
[10] Ankita Sharma, “Review on Major Cyber security Issues in Educational Sector,” International Journal of Computer Sciences and Engineering, Vol.9, Issue.12, pp.26-29, 2021.