OBL-GA based FCM with level sets for automatic GBM tumor segmentation in MR Images
B.S. Rao1 , E.S. Reddy2
Section:Research Paper, Product Type: Journal Paper
Volume-5 ,
Issue-1 , Page no. 85-20, Jan-2017
Online published on Jan 31, 2017
Copyright © B.S. Rao, E.S. Reddy . This is an open access article distributed under the Creative Commons Attribution License, which permits unrestricted use, distribution, and reproduction in any medium, provided the original work is properly cited.
View this paper at Google Scholar | DPI Digital Library
How to Cite this Paper
- IEEE Citation
- MLA Citation
- APA Citation
- BibTex Citation
- RIS Citation
IEEE Style Citation: B.S. Rao, E.S. Reddy, “OBL-GA based FCM with level sets for automatic GBM tumor segmentation in MR Images,” International Journal of Computer Sciences and Engineering, Vol.5, Issue.1, pp.85-20, 2017.
MLA Style Citation: B.S. Rao, E.S. Reddy "OBL-GA based FCM with level sets for automatic GBM tumor segmentation in MR Images." International Journal of Computer Sciences and Engineering 5.1 (2017): 85-20.
APA Style Citation: B.S. Rao, E.S. Reddy, (2017). OBL-GA based FCM with level sets for automatic GBM tumor segmentation in MR Images. International Journal of Computer Sciences and Engineering, 5(1), 85-20.
BibTex Style Citation:
@article{Rao_2017,
author = {B.S. Rao, E.S. Reddy},
title = {OBL-GA based FCM with level sets for automatic GBM tumor segmentation in MR Images},
journal = {International Journal of Computer Sciences and Engineering},
issue_date = {1 2017},
volume = {5},
Issue = {1},
month = {1},
year = {2017},
issn = {2347-2693},
pages = {85-20},
url = {https://www.ijcseonline.org/full_paper_view.php?paper_id=1163},
publisher = {IJCSE, Indore, INDIA},
}
RIS Style Citation:
TY - JOUR
UR - https://www.ijcseonline.org/full_paper_view.php?paper_id=1163
TI - OBL-GA based FCM with level sets for automatic GBM tumor segmentation in MR Images
T2 - International Journal of Computer Sciences and Engineering
AU - B.S. Rao, E.S. Reddy
PY - 2017
DA - 2017/01/31
PB - IJCSE, Indore, INDIA
SP - 85-20
IS - 1
VL - 5
SN - 2347-2693
ER -
![]() |
![]() |
![]() |
1001 | 486 downloads | 541 downloads |
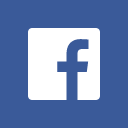
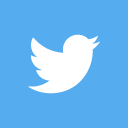
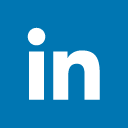
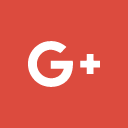
Abstract
This paper presents an automatic method for the segmentation of Glioblastoma multiforme(GBM) tumors from MRI images. The global search ability of Genetic Algorithm (GA) to optimize the Fuzzy C-means (FCM) clustering algorithm to obtain better clustering center. But the prematurity problem of GA itself has bad effects on the whole clustering. Therefore, in order to optimize the traditional GA-FCM algorithm�s clustering effect, in this work, we introduce the Opposition-based learning mechanism into GA, to construct an OBL-Genetic Algorithm (OBL-GA). The improved algorithm forms the next generation of evolutionary population by selecting the superior individuals in the collection of the sub generation and reverse sub generation, to increase the population diversity, and final to overcome the prematurity problem of GA. Then applying the improved algorithm to FCM, which gives better results and then resultant image, is applied with level sets, to exact delineation of GBM tumor. The validation is performed on a labeled BRATS data set. Our segmentation results are highly accurate, and compare favorably to the state of the art.
Key-Words / Index Term
Fuzzy-c means,Glioblastoma multiforme,Segmentation,Genetic Algorithm,Opposition based learning,MRI
References
[1] A. Rovira, J. Swanton, M. Tintor, E. Huerga, F. Barkhof, M. Filippi, J.L. Frederiksen, A. Langkilde, K. Miszkiel, C. Polman, M. Rovaris, J. Sastre-Garriga, D. Miller, X. Montalban, A single, early magnetic resonance imaging study in the diagnosis of multiple sclerosis. Arch. Neurol. vol. 66, no. 5, pp. 587-592, 2009.
[2] Y. Ge, Multiple sclerosis: the role of MR imaging. Am. J. Neuroradiol. vol. 27, no. 6, pp. 1165-1176, 2006.
[3] D. Hill, Neuroimaging to assess safety and efficacy of AD therapies. Expert Opin. Investig. Drugs. vol. 19, no. 1, pp. 23-26, 2010.
[4] R.E. Jung, J.M. Segall, H.J. Bockholt, R.A. Flores, S.M. Smith, R.S. Chavez, R.J. Haier, Neuroanatomy of creativity.Hum.Brain Mapp. vol. 31, no. 3, pp. 398-409n 2010.
[5] Verma R, Zacharaki EI, Ou Y, Cai H, Chawla S, Lee SK, et al. Multiparametric tissue characterization of brain neoplasms and their recurrence using pattern classification of MR images. Academic Radiology. 2008; 15: 966�977.
[6] Ruan S, Zhang N, Liao Q, Zhu Y. Image fusion for following-up brain tumor evolution. IEEE International Symposium on Biomedical Imaging: From Nano to Macro. 2011; 1: 281�284.
[7] Knopp EA, Cha S, Johnson G, et al. Glial neoplasms: dynamic contrast-enhanced T2*-weighted MR imaging. Radiology 1999;211: 791�798.
[8] Lev MH, Ozsunar Y, Henson JW, et al. Glial tumor grading and outcome prediction using dynamic spin-echo MR susceptibility mapping compared with conventional contrast-enhanced MR: confounding effect of elevated rCBV of oligodendrogliomas. AJNR Am J Neuroradiol 2004;25:214�221.
[9]. Vannier MW, Butterfield RL, Jordan D, Murphy WA, Levitt RG,Gado M. Multispectral analysis of magnetic resonance images.Radiology 1985;154:221�224.
[10].Kvinnsland Y, Brekke N, Taxt TM, Gru�ner R. Multispectral analysisof multimodal images. Acta Oncol 2009; 48:277�284.
[11]. Dickson S, Thomas BT, Goddard P. Using neural networks to automatically detect brain tumours in MR images. Int J Neural Syst 1997;8:91�99.
[12]. Abdolmaleki P, Mihara F, Masuda K, Buadu LD. Neural networks analysis of astrocytic gliomas from MRI appearances. Cancer Lett 1997;118:69�78.
[13]. Zhou J, Chan K, Chong H, Krishnan S. Extraction of brain tumor from MR images using one-class support vector machine. Conf Proc IEEE Eng Med Biol Soc 2005;6:6411�6414.
[14]. V.Vani, M. Kalaiselvi Geetha , "Automatic Tumor Classification of Brain MRI Images", International Journal of Computer Sciences and Engineering, Volume-04, Issue-10, Page No (144-151), Oct -2016.
[15]. Clark MC, Hall LO, Goldgof DB, Velthuizen R, Murtagh FR, Silbiger MS. Automatic tumor segmentation using knowledge-based techniques. IEEE Trans Med Imaging 1998;17:187�201.
[16]. Bezdek JC, Hall LO, Clark MC, Goldgof DB, Clarke LP. Medical image analysis with fuzzy models. Stat Methods Med Res 1997;6: 191�214.
[17] Azzeddine Riahi, "Image Segmentation Techniques Based on Fuzzy C-Means and Otsu, Applied to the Brain MRI in Tumor Detection", International Journal of Computer Sciences and Engineering, Volume-03, Issue-12, Page No (89-101), Dec -2015.
18] J.C. Bezdek, Pattern Recognization with Fuzzy Objective Function Algorithms, Advanced Applications in Pattern Recognization, Springer, Boston, Mass, USA, 1981.
[19] BackT, Evolutionary algorithms in theory and practice: evolution strategies evolutionary programming genetic algorithms. NewYork: Oxford University Press, 1996.
[20] Sabau, A. S, �Variable Density Based Genetic Clustering,� Symbolic and Numeric Algorithms for Scientific Computing.Timisoara, Romania, pp.200-206, September 2012.
[21] Wen-jie Ma, Wen-xia Yun, �Research progress of genetically algorithm,� Application Research of Computers, vol.29, no.4, pp.1201-1206, April 2012.
[22] Quan Liu, Xiao-yan Wang, and Qi-ming Fu, �Double Elite Coevolutionary Genetic Algorithm,� Journal of Software, vol.23, no.4, pp.765-775, April 2012.
[23] T. Chan and L. Vese, "Active contours without edges� in IEEE transactions on image processing 10(2), 2001, pp. 266-277.
[24] Vishnumurthy T D, Mohana H S Vaibhav A Meshram and Pramod Kammar, "Suppression of Herringbone Artifact in MR Images of Brain Using Combined Wavelet and FFT Based Filtering Technique", International Journal of Computer Sciences and Engineering, Volume-04, Issue-02, Page No (66-71), Feb -2016.
[25] B. Menze, A. Jakab, S. Bauer, J. Kalpathy-Cramer, K. Farahani, and et al. on Medical Imaging, 2014.
[26] Bandhyopadhyay SK, Paul TU. Automatic segmentation of brain tumour from multiple images of brain MRI. Int J Appl Innovat Eng Manage (IJAIEM) 2013;2(1):240�8
[27] LJun-Hao Zhang, Ming Hu HA , Jing Wu,� Implementation of Rough Fuzzy K-means Clustering Algorithm in Matlab�, Proceedings of Ninth International Conference on Machine Learning and Cybernetics�, July 2010.
[28] Biju.V.G, Mythili.P, �A Genetic Algorithm based Fuzzy C Mean Clustering Model for Segmenting Microarray Images� IJCA , Volume 52� No.11, 2012
[29] Vipin Y. Borole, Seema S. Kawathekar, "Study of various DIP Techniques used for Brain Tumor detection and tumor area calculation using MRI images", International Journal of Computer Sciences and Engineering, Volume-04, Issue-07, Page No (39-43), Jul -2016.