Survey of Technologies for Evaluation of Student Dropout Using Educational Data
Sumit Gupta1 , Amit Ranjan2
Section:Survey Paper, Product Type: Journal Paper
Volume-07 ,
Issue-10 , Page no. 167-171, May-2019
CrossRef-DOI: https://doi.org/10.26438/ijcse/v7si10.167171
Online published on May 05, 2019
Copyright © Sumit Gupta, Amit Ranjan . This is an open access article distributed under the Creative Commons Attribution License, which permits unrestricted use, distribution, and reproduction in any medium, provided the original work is properly cited.
View this paper at Google Scholar | DPI Digital Library
How to Cite this Paper
- IEEE Citation
- MLA Citation
- APA Citation
- BibTex Citation
- RIS Citation
IEEE Style Citation: Sumit Gupta, Amit Ranjan, “Survey of Technologies for Evaluation of Student Dropout Using Educational Data,” International Journal of Computer Sciences and Engineering, Vol.07, Issue.10, pp.167-171, 2019.
MLA Style Citation: Sumit Gupta, Amit Ranjan "Survey of Technologies for Evaluation of Student Dropout Using Educational Data." International Journal of Computer Sciences and Engineering 07.10 (2019): 167-171.
APA Style Citation: Sumit Gupta, Amit Ranjan, (2019). Survey of Technologies for Evaluation of Student Dropout Using Educational Data. International Journal of Computer Sciences and Engineering, 07(10), 167-171.
BibTex Style Citation:
@article{Gupta_2019,
author = {Sumit Gupta, Amit Ranjan},
title = {Survey of Technologies for Evaluation of Student Dropout Using Educational Data},
journal = {International Journal of Computer Sciences and Engineering},
issue_date = {5 2019},
volume = {07},
Issue = {10},
month = {5},
year = {2019},
issn = {2347-2693},
pages = {167-171},
url = {https://www.ijcseonline.org/full_spl_paper_view.php?paper_id=996},
doi = {https://doi.org/10.26438/ijcse/v7i10.167171}
publisher = {IJCSE, Indore, INDIA},
}
RIS Style Citation:
TY - JOUR
DO = {https://doi.org/10.26438/ijcse/v7i10.167171}
UR - https://www.ijcseonline.org/full_spl_paper_view.php?paper_id=996
TI - Survey of Technologies for Evaluation of Student Dropout Using Educational Data
T2 - International Journal of Computer Sciences and Engineering
AU - Sumit Gupta, Amit Ranjan
PY - 2019
DA - 2019/05/05
PB - IJCSE, Indore, INDIA
SP - 167-171
IS - 10
VL - 07
SN - 2347-2693
ER -
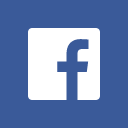
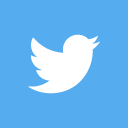
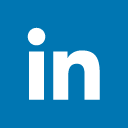
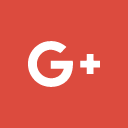
Abstract
Interpretation of the dropout students and the reason behind is the most important for the universities. Due to many different reasons such as pressure, low performance, high expectations from family, faculties and individuals it is being tough to sustain for the students. Most important source of knowing the expressions of the students in these instances is their social media interactions with other students. They express their major problems on it. But this is a challenge to process such huge data and evaluating expressions from it. Data mining techniques have given a boost in such processing and application of machine learning has become boon for it. It is found that there are many such techniques available but newest techniques which are best fit in processing of expressional data is machine learning. Surveys of such techniques have become a great source of expression evaluation.
Key-Words / Index Term
Cloud Computing, Fault Tolerance, Virtual Machines Migration, Resource Management
References
[1] V. Hegde and P. P. Prageeth, "Higher education student dropout prediction and analysis through educational data mining," 2018 2nd International Conference on Inventive Systems and Control (ICISC), Coimbatore, 2018, pp. 694-699. doi: 10.1109/ICISC.2018.8398887
[2] M. C. Nicoletti, M. Marques and M. P. Guimaraes, "A data mining approach for forecasting students` performance," 2018 13th Iberian Conference on Information Systems and Technologies (CISTI), Caceres, 2018, pp. 1-7. doi: 10.23919/CISTI.2018.8399389
[3] W. Zhang and S. Qin, "A brief analysis of the key technologies and applications of educational data mining on online learning platform," 2018 IEEE 3rd International Conference on Big Data Analysis (ICBDA), Shanghai, 2018, pp. 83-86. doi: 10.1109/ICBDA.2018.8367655
[4] S. R. Guruvayur and R. Suchithra, "A detailed study on machine learning techniques for data mining," 2017 International Conference on Trends in Electronics and Informatics (ICEI), Tirunelveli, 2017, pp. 1187-1192. doi: 10.1109/ICOEI.2017.8300900
[5] A. F. Meghji, N. Ahmed Mahoto, M. A. Unar and M. Akram Shaikh, "Analysis of Student Performance using EDM Methods," 2018 5th International Multi-Topic ICT Conference (IMTIC), Jamshoro, 2018, pp. 1-7. doi: 10.1109/IMTIC.2018.8467226
[6] C. Romero, S. Ventura, “Educational data mining : a review of the state of the art”, IEEE Transactions on Systems, Man, and Cybernetics, (Applications and reviews), Vol. 40, Issue 6, pp 601- 618, 2010.
[7] R.S. Baker, A.T. Corbett,K.R. Koedinger, “Detecting Student Misuse of Intelligent Tutoring Systems”. Proceedings of the 7th International Conference on Intelligent Tutoring Systems, pp 531-540, 2004.
[8] T. Tang, G. McCalla,” Smart recommendation for an evolving elearning system: architecture and experiment”, International Journal on E-Learning, vol. 4, issue 1, pp 105-129, 2005.
[9] M. de Raadt, M. Hamilton, R.F. Lister, J. Tutty, B. Baker,I. Box, & M. Petre. “Approaches to learning in computer programming students and their effect on success”. Research and Development in Higher Education Series, Vol. 28, pp 407-414, 2005.
[10] Saurabh Pal, “Data Mining: A Prediction For Performance Improvement Using Classification”, International Journal of Computer Science and Information Security, Vol. 9, Isuue 4, pp 136- 140, 2011. Proceedings of the International Conference on Inventive Computing and Informatics (ICICI 2017) IEEE Xplore Compliant - Part Number: CFP17L34-ART, ISBN: 978-1-5386-4031-9
[11] Romero, C., & Ventura, S. (2010). Educational Data Mining: A Review of the State of the Art. IEEE Transactions on Systems, Man, and Cybernetics, Part C (Applications and Reviews), 40(6), 601–618. doi:10.1109/tsmcc.2010.2053532