Extensive Review on Computational Predictions of Genomic Regulatory Sequences
asikala S1 , Ratha Jeyalakshmi T2
Section:Review Paper, Product Type: Journal Paper
Volume-07 ,
Issue-08 , Page no. 91-94, Apr-2019
CrossRef-DOI: https://doi.org/10.26438/ijcse/v7si8.9194
Online published on Apr 10, 2019
Copyright © Sasikala S, Ratha Jeyalakshmi T . This is an open access article distributed under the Creative Commons Attribution License, which permits unrestricted use, distribution, and reproduction in any medium, provided the original work is properly cited.
View this paper at Google Scholar | DPI Digital Library
How to Cite this Paper
- IEEE Citation
- MLA Citation
- APA Citation
- BibTex Citation
- RIS Citation
IEEE Style Citation: Sasikala S, Ratha Jeyalakshmi T, “Extensive Review on Computational Predictions of Genomic Regulatory Sequences,” International Journal of Computer Sciences and Engineering, Vol.07, Issue.08, pp.91-94, 2019.
MLA Style Citation: Sasikala S, Ratha Jeyalakshmi T "Extensive Review on Computational Predictions of Genomic Regulatory Sequences." International Journal of Computer Sciences and Engineering 07.08 (2019): 91-94.
APA Style Citation: Sasikala S, Ratha Jeyalakshmi T, (2019). Extensive Review on Computational Predictions of Genomic Regulatory Sequences. International Journal of Computer Sciences and Engineering, 07(08), 91-94.
BibTex Style Citation:
@article{S_2019,
author = {Sasikala S, Ratha Jeyalakshmi T},
title = {Extensive Review on Computational Predictions of Genomic Regulatory Sequences},
journal = {International Journal of Computer Sciences and Engineering},
issue_date = {4 2019},
volume = {07},
Issue = {08},
month = {4},
year = {2019},
issn = {2347-2693},
pages = {91-94},
url = {https://www.ijcseonline.org/full_spl_paper_view.php?paper_id=924},
doi = {https://doi.org/10.26438/ijcse/v7i8.9194}
publisher = {IJCSE, Indore, INDIA},
}
RIS Style Citation:
TY - JOUR
DO = {https://doi.org/10.26438/ijcse/v7i8.9194}
UR - https://www.ijcseonline.org/full_spl_paper_view.php?paper_id=924
TI - Extensive Review on Computational Predictions of Genomic Regulatory Sequences
T2 - International Journal of Computer Sciences and Engineering
AU - Sasikala S, Ratha Jeyalakshmi T
PY - 2019
DA - 2019/04/10
PB - IJCSE, Indore, INDIA
SP - 91-94
IS - 08
VL - 07
SN - 2347-2693
ER -
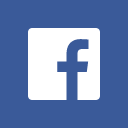
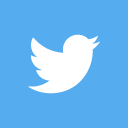
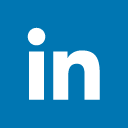
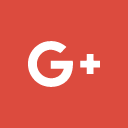
Abstract
This paper focuses an extensive study of the existing computational work related to prediction of gene regulatory sequences and the relevant factors based on different properties. A hybrid approach is studied which combines position correlation score function and increment of diversity to elucidate signal features and composition features of sequences to improve the accuracy of promoter classifiers. It is found that Markov Model of order K is used to extract features from k-mer frequency of the sequence. Also a Support Vector Machine (SVM) is applied with the transcription signals such as Gc box, TATA box, CAAT box, NIT box and CpG islands and modified Mahalanob discriminant to predict Eukaryotic and Prokaryotic promoters. It is studied that a new approach is implemented using Artificial Neural Network (ANN) with the properties namely curvature, stacking energy and Stress Induced duplex Destabilization (SIDD). To analyze sequence characteristics of prokaryotic and eukaryotic promoters, Convolutional Neural Networks (CNN) is found to be contributing a significant role. This paper analyses the use of a tool bTSSfinder for promoter predicting models. It is identified that an algorithm exist for promoter prediction based on evolutionarily conserved sequences by concentrating AT-rich elements and G-quadruplex sequences using various statistical measures such as recall, precision, specificity accuracy and F1- scores . Algorithms using machine learning based approach are studied to discover promoters in nucleotide sequence using entropy based feature. Some of the remarkable DNA structural features such as DNA bending stiffness, duplex free energy, duplex disrupt energy, stacking energy, DNA denaturation, protein deformation, nucleosome position, propeller twist are studied. Multifarious promoter prediction models which are found to be predicting promoters associated with PoI II sequence, sigma factors such as σ70, σ66, σ54 and transcription factor binding sites. This paper studied that bidirectional genes are co expressed and tends to be involved in the same biological functions with stronger expression correlation. Also studied the intergenic regions enriched of regulatory elements are essential for the transcription initiation. Though various models are found to be effective, still they need to uncover various characteristics. Because of the dynamicity of gene regulatory process, promoter prediction models still require improvement .Indeed this field has a wider exposure of detailed research work.
Key-Words / Index Term
Eukaryotic and Prokaryotic Promoters, Sigma Factor, TSS
References
[1] Humberto Barrios, Brenda Valderrama & Enrique Morett , “Compilation and analysis of σ54 dependent promoter sequences” , Nucleic Acids Research 1999 , Vol. 27 , No.22 4305-4313
[2]Qian-Zhong Li, Hao Lin , “The recognition and prediction of sigma70 promoters in Escherichia coli K-12 “ , Journal of theoretical Biology 242 (2006) 135 - 141
[3]Ronna R Mallios, David M Ojcius and David H Ardel , “An iterative strategy combining biophysical criteria and duration hidden Markov models for structural predictions of Chlamydia trachomatis σ66 promoters” , BMC Bioinformatics 2009 , 10:27
[4] Wang Q1, Wan L, Li D, Zhu L, Qian M, Deng M , “Searching for bidirectional promoters in Arabidopsis thaliana” , BMC Bioinformatics. 2009 Jan 30;10 Suppl 1:S29
[5] Guofeng Meng Axel Mosig and Martin Vingron, “A computational evaluation of over-representation of regulatory motifs in the promoter regions of differentially expressed genes”, BMC Bioinformatics 2010 11:267
[6] Charles Bland, Abigail S Newsome, Aleksandra A Markovets , “Promoter prediction in E. coli based on SIDD profiles and Artificial Neural Networks” 7th Annual MCBIOS Conference Bioinformatics: Systems, Biology, Informatics and Computation Jonesboro, AR, USA. 19-20 February 2010 https://doi.org/10.1186/1471-2105-11-S6-S17
[7]Ravi Gupta, Priyankara Wikramasinghe, Anirban Bhattacharyya, Francisco A Perez, Sharmistha Pal and Ramana V Davuluri, “Annotation of gene promoters by integrative data-mining of ChIP-seq Pol-II enrichment data” , BMC Bioinformatics 2010, 11(Suppl 1):S65
[8] C. Premalatha, Chandrabose Aravindan, Kamarajan Kannan , “ Promoter prediction in eukaryotes using soft computing techniques” , 2011 IEEE Conference - Recent Advances in Intelligent Computational Systems
[9]Hao Lin Qian-Zhong Li , “Eukaryotic and prokaryotic promoter prediction using hybrid approach”, Theory Biosci. (2011) 130: 91–100
[10] Yanglan Gan, Jihong Guan and Shuigeng Zhou , “A comparison study on feature selection of DNA structural properties for promoter prediction”, BMC Bioinformatics 2012, 13:4
[11] Zaw ZawHtikea , Shoon LeiWin , “ Recognition of Promoters in DNA Sequences Using Weightily Averaged One-dependence Estimators” , Procedia Computer Science, 2013 , Vol 23, Pages 60-67
[12] Smitha C S , Saritha R , “Computational Transcription Factor Binding Prediction Using Random Forests” , International Conference on Control, Instrumentation, Communication and Computational Technologies (ICCICCT) 2014
[13] ]Lin H, Deng EZ, Ding H, Chen W, Chou KC , “ iPro54-PseKNC: a sequence-based predictor for identifying sigma-54 promoters in prokaryote with pseudo k-tuple nucleotide composition” , Nucleic Acids Res. 2014 Dec 1;42(21):12961-72.
[14] Gusmao EG, Dieterich C, Zenke M, Costa IG , “.Detection of active transcription factor binding sites with the combination of DNase hypersensitivity and histone modifications “, Bioinformatics. 2014 Nov 15;30 (22): 3143-51
[15] Tianyin Zhoua, Ning Shenb,c, Lin Yanga, Namiko Abed, John Hortonc,e, Richard S. Mannd,f, Harmen J. Bussemakerf,g, Raluca Gordânc,e and Remo Rohsa , “ Quantitative modeling of transcription factor binding specificities using DNA shape” , Proceedings of National Academy of Sciences , 2015 , 112(15), 4654–4659.
[16] Ramzan Kh. Umarov and Victor V. Solovyev , “Recognition of prokaryotic and eukaryotic promoters using convolutional deep learning neural networks” , PLoS One . 2017; 12(2): e0171410.
[17] Ilham Ayub Shahmuradov , Rozaimi Mohamad Razali, Salim Bougouffa, Aleksandar Radovanovic and Vladimir B. Bajic , “bTSSfinder: a novel tool for the prediction of promoters in cyanobacteria and Escherichia coli “ , Bioinformatics, 33(3), 2017, 334–340 doi: 10.1093/bioinformatics/btw629
[18] Wenying He , Cangzhi Jia, Yucong Duan and Quan Zou , “70ProPred: a predictor for discovering σ70 promoters based on combining multiple features “ , 11th International Conference on Systems Biology (ISB 2017) Shenzhen, China. 18-21 August 2017
[19] Marco Di Salvo, Eva Pinatel, Adelfia Tala, Marco Fondi , Clelia Peano and Pietro Alifano , “G4PromFinder: an algorithm for predicting transcription promoters in GC-rich bacterial genomes based on AT-rich elements and G-quadruplex motifs” BMC Bioinformatics (2018) 19:36