ECG Signal Feature Extraction and Classification: Survey
Bhagyashri Bhirud1 , Vinod Pachghare2
Section:Survey Paper, Product Type: Journal Paper
Volume-07 ,
Issue-07 , Page no. 1-6, Mar-2019
Online published on Mar 30, 2019
Copyright © Bhagyashri Bhirud, Vinod Pachghare . This is an open access article distributed under the Creative Commons Attribution License, which permits unrestricted use, distribution, and reproduction in any medium, provided the original work is properly cited.
View this paper at Google Scholar | DPI Digital Library
How to Cite this Paper
- IEEE Citation
- MLA Citation
- APA Citation
- BibTex Citation
- RIS Citation
IEEE Style Citation: Bhagyashri Bhirud, Vinod Pachghare, “ECG Signal Feature Extraction and Classification: Survey,” International Journal of Computer Sciences and Engineering, Vol.07, Issue.07, pp.1-6, 2019.
MLA Style Citation: Bhagyashri Bhirud, Vinod Pachghare "ECG Signal Feature Extraction and Classification: Survey." International Journal of Computer Sciences and Engineering 07.07 (2019): 1-6.
APA Style Citation: Bhagyashri Bhirud, Vinod Pachghare, (2019). ECG Signal Feature Extraction and Classification: Survey. International Journal of Computer Sciences and Engineering, 07(07), 1-6.
BibTex Style Citation:
@article{Bhirud_2019,
author = {Bhagyashri Bhirud, Vinod Pachghare},
title = {ECG Signal Feature Extraction and Classification: Survey},
journal = {International Journal of Computer Sciences and Engineering},
issue_date = {3 2019},
volume = {07},
Issue = {07},
month = {3},
year = {2019},
issn = {2347-2693},
pages = {1-6},
url = {https://www.ijcseonline.org/full_spl_paper_view.php?paper_id=894},
publisher = {IJCSE, Indore, INDIA},
}
RIS Style Citation:
TY - JOUR
UR - https://www.ijcseonline.org/full_spl_paper_view.php?paper_id=894
TI - ECG Signal Feature Extraction and Classification: Survey
T2 - International Journal of Computer Sciences and Engineering
AU - Bhagyashri Bhirud, Vinod Pachghare
PY - 2019
DA - 2019/03/30
PB - IJCSE, Indore, INDIA
SP - 1-6
IS - 07
VL - 07
SN - 2347-2693
ER -
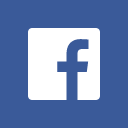
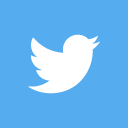
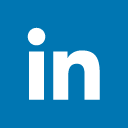
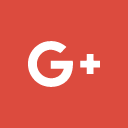
Abstract
Nowadays due to busy and hectic lifestyle, many people cannot pay enough attention to their health. Stress, junk food, obesity, smoking and lack of exercise leads to heart diseases. It is one of major cause leading rise to death rate. ECG (Electrocardiogram) is the best and easiest way to record and analyze the electrical and muscular activities of heart. Due to nonlinearity and complexity of ECG signals, it requires significant amount of training to analyze and study the ECG waveform. For preventive measures and predictive analysis, it is necessary to analyze these waveforms in faster, efficient way and in real time too. Number of methods and techniques have been developed in recent time. Different techniques and methodologies are discussed in this literature review.
Key-Words / Index Term
ECG Signal, Arrhythmia, Feature Extraction, Feature Selection
References
[1] Venkatesan, C., P. Karthigaikumar, Anand Paul, S. Satheeskumaran, and R. Kumar. ”ECG Signal Preprocessing and SVM Classifier-Based Abnormality Detection in Remote Healthcare Applications.” IEEE Access 6 (2018): 9767-9773
[2] Li, Qiao, Cadathur Rajagopalan, and Gari D. Clifford. ”Ventricular fibrillation and tachycardia classification using a machine learning approach.” IEEE Transactions on Biomedical Engineering 61, no. 6 (2014): 1607-1613
[3] Hammad, Mohamed, Asmaa Maher, Kuanquan Wang, Feng Jiang, and Moussa Amrani. ”Detection of abnormal heart conditions based on characteristics of ECG signals.” Measurement 125 (2018): 634-644
[4] Alonso-Atienza, Felipe, Eduardo Morgado, Lorena Fernandez-Martinez, Arcadi Garc´ıa-Alberola, and Jos´e Luis Rojo-Alvarez. ”Detection of life-threatening arrhythmias using feature selection and support vector machines.” IEEE Trans. Biomed. Eng 61, no. 3 (2014): 832-840
[5] Xia, Yufa, Huailing Zhang, Lin Xu, Zhifan Gao, Heye Zhang, Huafeng Liu, and Shuo Li. ”An automatic cardiac arrhythmia classification system with wearable electrocardiogram.” IEEE Access 6 (2018): 16529-16538
[6] http://www.ni.com/data-acquisition/what-is/
[7] Chouhan, V. S., and Sarabjeet Singh Mehta. ”Total removal of baseline drift from ECG signal.” In Computing: Theory and Applications, 2007. ICCTA’07. International Conference on, pp. 512-515. IEEE, 2007.
[8] https://www.physionet.org/physiotools/wag/ecgpuw-1.htm
[9] Zhang, Xu-Sheng, Yi-Sheng Zhu, Nitish V. Thakor, and Zhi-Zhong Wang. ”Detecting ventricular tachycardia and fibrillation by complexity measure.” IEEE Transactions on biomedical engineering 46, no. 5 (1999): 548-555
[10] Kuo, S. ”Computer detection of ventricular fibrillation.” Proc. of Computers in Cardiology, IEEE Comupter Society (1978): 347-349
[11] Barro, S., R. Ruiz, D. Cabello, and J. Mira. ”Algorithmic sequential decision-making in the frequency domain for life threatening ventricular arrhythmias and imitative artefacts: a diagnostic system.” Journal of biomedical engineering 11, no. 4 (1989): 320-328
[12] Amann, Anton, Robert Tratnig, and Karl Unterkofler. ”Detecting ventricular fibrillation by time-delay methods.” IEEE Transactions on Biomedical Engineering 54, no. 1 (2007): 174-177
[13] Jekova, Irena, and Vessela Krasteva. ”Real time detection of ventricular fibrillation and tachycardia.” Physiological measurement 25, no. 5 (2004): 1167
[14] Jekova, Irena. ”Shock advisory tool: Detection of life-threatening cardiac arrhythmias and shock success prediction by means of a common parameter set.” Biomedical Signal Processing and Control 2, no. 1 (2007): 25-33
[15] Li, Qiao, Roger G. Mark, and Gari D. Clifford. ”Robust heart rate estimation from multiple asynchronous noisy sources using signal quality indices and a Kalman filter.” Physiological measurement 29, no. 1 (2007): 15
[16] Thakor, Nitish V., Y-S. Zhu, and K-Y. Pan. ”Ventricular tachycardia and fibrillation detection by a sequential hypothesis testing algorithm.” IEEE Transactions on Biomedical Engineering 37, no. 9 (1990): 837-843
[17] Arafat, Muhammad Abdullah, Abdul Wadud Chowdhury, and Md Kamrul Hasan. ”A simple time domain algorithm for the detection of ventricular fibrillation in electrocardiogram.” Signal, Image and Video Processing 5, no. 1 (2011): 1-10
[18] Amann, Anton, Robert Tratnig, and Karl Unterkofler. ”Reliability of old and new ventricular fibrillation detection algorithms for automated external defibrillators.” Biomedical engineering online 4, no. 1 (2005): 60
[19] Anas, Emran M. Abu, Soo Y. Lee, and Md K. Hasan. ”Sequential algorithm for life threatening cardiac pathologies detection based on mean signal strength and EMD functions.” Biomedical engineering online 9, no. 1 (2010): 43
[20] Dzwonczyk, Roger, Charles G. Brown, and Howard A. Werman. ”The median frequency of the ECG during ventricular fibrillation: its use in an algorithm for estimating the duration of cardiac arrest.” IEEE Transactions on Biomedical Engineering 37, no. 6 (1990): 640-646
[21] Zhang, Xu-Sheng, Yi-Sheng Zhu, Nitish V. Thakor, and Zhi-Zhong Wang. ”Detecting ventricular tachycardia and fibrillation by complexity measure.” IEEE Transactions on biomedical engineering 46, no. 5 (1999): 548-555
[22] Amann, Anton, Robert Tratnig, and Karl Unterkofler. ”A new ventricular fibrillation detection algorithm for automated external defibrillators.” database 1, no. 2 (2005): 3
[23] Li, Haiyan, Wenguang Han, Chao Hu, and Max Q-H. Meng. ”Detecting ventricular fibrillation by fast algorithm of dynamic sample entropy.” In Robotics and Biomimetics (ROBIO), 2009 IEEE International Conference on, pp. 1105-1110. IEEE, 2009