A Study on Scalable and automated Causal Relationship Discovery Strategies In Data Analytics
S. Sajida1 , M. Padmavathamma2
Section:Research Paper, Product Type: Journal Paper
Volume-07 ,
Issue-06 , Page no. 9-15, Mar-2019
CrossRef-DOI: https://doi.org/10.26438/ijcse/v7si6.915
Online published on Mar 20, 2019
Copyright © S. Sajida, M. Padmavathamma . This is an open access article distributed under the Creative Commons Attribution License, which permits unrestricted use, distribution, and reproduction in any medium, provided the original work is properly cited.
View this paper at Google Scholar | DPI Digital Library
How to Cite this Paper
- IEEE Citation
- MLA Citation
- APA Citation
- BibTex Citation
- RIS Citation
IEEE Style Citation: S. Sajida, M. Padmavathamma, “A Study on Scalable and automated Causal Relationship Discovery Strategies In Data Analytics,” International Journal of Computer Sciences and Engineering, Vol.07, Issue.06, pp.9-15, 2019.
MLA Style Citation: S. Sajida, M. Padmavathamma "A Study on Scalable and automated Causal Relationship Discovery Strategies In Data Analytics." International Journal of Computer Sciences and Engineering 07.06 (2019): 9-15.
APA Style Citation: S. Sajida, M. Padmavathamma, (2019). A Study on Scalable and automated Causal Relationship Discovery Strategies In Data Analytics. International Journal of Computer Sciences and Engineering, 07(06), 9-15.
BibTex Style Citation:
@article{Sajida_2019,
author = {S. Sajida, M. Padmavathamma},
title = {A Study on Scalable and automated Causal Relationship Discovery Strategies In Data Analytics},
journal = {International Journal of Computer Sciences and Engineering},
issue_date = {3 2019},
volume = {07},
Issue = {06},
month = {3},
year = {2019},
issn = {2347-2693},
pages = {9-15},
url = {https://www.ijcseonline.org/full_spl_paper_view.php?paper_id=858},
doi = {https://doi.org/10.26438/ijcse/v7i6.915}
publisher = {IJCSE, Indore, INDIA},
}
RIS Style Citation:
TY - JOUR
DO = {https://doi.org/10.26438/ijcse/v7i6.915}
UR - https://www.ijcseonline.org/full_spl_paper_view.php?paper_id=858
TI - A Study on Scalable and automated Causal Relationship Discovery Strategies In Data Analytics
T2 - International Journal of Computer Sciences and Engineering
AU - S. Sajida, M. Padmavathamma
PY - 2019
DA - 2019/03/20
PB - IJCSE, Indore, INDIA
SP - 9-15
IS - 06
VL - 07
SN - 2347-2693
ER -
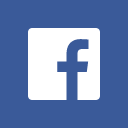
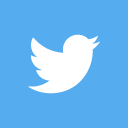
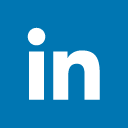
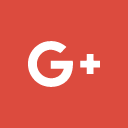
Abstract
Causation is one of the crucial relationships among the related variables that provide good stuff for data analytics. A causal relationship among a set of events exists when one or group of event/s is the result of the occurrence of the other event or a set of events. The primary objective of any research in data analytics or scientific analysis is to identify the level to which a relation exists among the subjective variables. Causal research can facilitate business environment to quantify the effect of present business practices on future production levels to aid in the business planning process. The process of discovering causal relationships among variables have multitude application areas like critical care services in medicine, advertising, bioinformatics, road safety ,share markets, and too more to be included. The present work targeted to study the existing methods of causal relationship discovery. The study also tried to propose the automated and straight forward causal relationship discovery methods which are scalable.
Key-Words / Index Term
Decision tree, causal relationship,bayesian netorks,Structural Equation models,CDT
References
[1]. Alfieri’s C. F., et al. [C. F. Aliferis, A. Statnikov, I. Tsamardinos, S. Mani, and X. D.Koutsoukos, “Local causal and Markov blanket induction for causal discovery and feature selection for classification part I: Algorithms and empirical evaluation”, Journal of Machine Learning. Res., vol. 11, pp. 171–234, 2010.
[2]. Birch M.W.” The Detection of Partial Association”, Journal of Royal Statistical Society Aeries B (Methodological), Vol. 26. No. 2 (1964), pp. 313-324.
[3]. Bollen K.A., Pearl J. ”Eight Myths About Causality and Structural Equation Models. In: Morgan S.” (eds), Handbook of Causal Analysis for Social Research. Springer, Dordrecht (2013)
[4]. Christopher D. Ittner, “Strengthening causal inferences in positivist field studies”, Accounting, Organizations and Society 39 (2014) 545–549.
[5]. Constantin F. Aliferis, Alexander Statnikov, Ioannis Tsamardinos, Subramani Mani, Xenofon D. Koutsoukos, “Local Causal and Markov Blanket Induction for Causal Discovery and Feature Selection for Classification”, Journal of Machine Learning Research 11 (2010) 171-234.
[6]. Donald B. Rubin [Donald B. Rubin, “Estimating Causal Effects from Large Data Sets Using Propensity Scores” 15 October 1997 | Volume 127 Issue 8 Part 2 | Pages 757-763, Annals of Internal Medicine, American College of Physicians]
[7]. Frey L., D. Fisher, I. Tsamardinos, C. Aliferis, and A. Statnikov, “Identifying Markov blankets with decision tree induction,” in Proc. 3rd IEEE Int. Conf. Data Mining, Nov. 2003, pp. 59–66.]
[8]. Jin Z., J. Li, L. Liu, T. D. Le, B. Sun, and R. Wang, “Discovery of causal rules using partial association,” in Proc. IEEE 12th Int. Conf. Data Mining, Dec. 2012, pp. 309–318]
[9]. Jiuyong Li, et al [Jiuyong Li, Thuc Duy Le, Lin Liu, Jixue Liu, Zhou Jin, Bingyu Sun, and Saisai Ma”, From Observational Studies to Causal Rule Mining”. ACM Trans. Intell. Syst. Technol.2015
[10]. Jiuyong Li, Saisai Ma, Thuc Duy Le, Lin Liu and Jixue Liu “Causal Decision Trees,” arXiv: 1508.03812v1 [cs.AI] 16 Aug 2015]
[11]. Jiuyong Li, Lin Liu, Thuc Duy Le. Jiuyong Li • Lin Liu • Thuc Duy Le, “Practical Approaches to Causal Relationship Exploration” Jiuyong Li School of Information Technology and Mathematical Sciences University.2015]
[12]. Li. j, Liu. L, Le. T., “Practical approaches to causal relationship exploration”2015. X. 80 p. 55 illu., softcover, ISBN:978-3-319-14432-0, http://www.springer.com/978-3-319-14432-0]
[13]. Magliacane Sara, Tom Claassen, Joris M. Mooij, “Joint Causal Inference from Observational and Experimental Datasets”, Journal of Machine Learning Research, March 2017.
[14]. S. L. Morgan and D. J. Harding, “Matching estimators of causal effects: Prospects and pitfalls in theory and practice,” Sociological Methods Res., vol. 35, pp. 3–60, 2006.
[15]. Sander Greenland and Babette Brumback, “An overview of relations among causal modelling methods”,International Journal of Epidemiology, Volume 31, Issue 5, 1 October 2002, Pages 1030–1037.
[16]. Spirtes P., C. C. Glymour, and R. Scheines, Causation, Predication, and Search, 2nd ed. Cambridge, MA, USA: MIT Press, 2000.
[17]. Spirtes Peter. “Introduction to Causal Inference, ” Journal of Machine Learning Research 11 (2010) 1643-1662.
[18]. Stephen L. Morgan, David J. Harding, “Matching Estimators of Causal Effects”, Sociological Methods& Research Volume 35 Number 1August 2006 3-60 _ 2006 Sage Publications
[19]. Swati Hira and P. S. Deshpande ,“Mining precise cause and effect rules in large time series data of socio‑economic indicators,” SpringerPlus (2016) 5:1625.
[20]. Yeying Zhu, Donna L. Coffman and Debashis Ghosh, “A Boosting Algorithm for Estimating Generalized Propensity Scores with Continuous Treatments”, J. Causal Infer. 2015; 3(1): 25–40.