An Analysis on the Performance of Rapid Miner and R Programming Language as Data Pre-processing Tools for Unsupervised Form of Insurance Claim Dataset
Surya Susan Thomas1 , Ananthi Sheshasaayee2
Section:Research Paper, Product Type: Journal Paper
Volume-07 ,
Issue-05 , Page no. 1-4, Mar-2019
CrossRef-DOI: https://doi.org/10.26438/ijcse/v7si5.14
Online published on Mar 10, 2019
Copyright © Surya Susan Thomas, Ananthi Sheshasaayee . This is an open access article distributed under the Creative Commons Attribution License, which permits unrestricted use, distribution, and reproduction in any medium, provided the original work is properly cited.
View this paper at Google Scholar | DPI Digital Library
How to Cite this Paper
- IEEE Citation
- MLA Citation
- APA Citation
- BibTex Citation
- RIS Citation
IEEE Style Citation: Surya Susan Thomas, Ananthi Sheshasaayee, “An Analysis on the Performance of Rapid Miner and R Programming Language as Data Pre-processing Tools for Unsupervised Form of Insurance Claim Dataset,” International Journal of Computer Sciences and Engineering, Vol.07, Issue.05, pp.1-4, 2019.
MLA Style Citation: Surya Susan Thomas, Ananthi Sheshasaayee "An Analysis on the Performance of Rapid Miner and R Programming Language as Data Pre-processing Tools for Unsupervised Form of Insurance Claim Dataset." International Journal of Computer Sciences and Engineering 07.05 (2019): 1-4.
APA Style Citation: Surya Susan Thomas, Ananthi Sheshasaayee, (2019). An Analysis on the Performance of Rapid Miner and R Programming Language as Data Pre-processing Tools for Unsupervised Form of Insurance Claim Dataset. International Journal of Computer Sciences and Engineering, 07(05), 1-4.
BibTex Style Citation:
@article{Thomas_2019,
author = {Surya Susan Thomas, Ananthi Sheshasaayee},
title = {An Analysis on the Performance of Rapid Miner and R Programming Language as Data Pre-processing Tools for Unsupervised Form of Insurance Claim Dataset},
journal = {International Journal of Computer Sciences and Engineering},
issue_date = {3 2019},
volume = {07},
Issue = {05},
month = {3},
year = {2019},
issn = {2347-2693},
pages = {1-4},
url = {https://www.ijcseonline.org/full_spl_paper_view.php?paper_id=794},
doi = {https://doi.org/10.26438/ijcse/v7i5.14}
publisher = {IJCSE, Indore, INDIA},
}
RIS Style Citation:
TY - JOUR
DO = {https://doi.org/10.26438/ijcse/v7i5.14}
UR - https://www.ijcseonline.org/full_spl_paper_view.php?paper_id=794
TI - An Analysis on the Performance of Rapid Miner and R Programming Language as Data Pre-processing Tools for Unsupervised Form of Insurance Claim Dataset
T2 - International Journal of Computer Sciences and Engineering
AU - Surya Susan Thomas, Ananthi Sheshasaayee
PY - 2019
DA - 2019/03/10
PB - IJCSE, Indore, INDIA
SP - 1-4
IS - 05
VL - 07
SN - 2347-2693
ER -
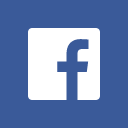
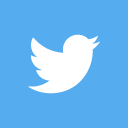
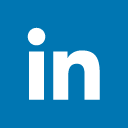
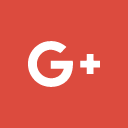
Abstract
Data Science has emerged as a super science in almost all the sectors of analytics. Data Mining is the key runner and the pillar stone of data analytics. The analysis and study of any form of data has become so relevant in todays’ scenario and the output from these studies give great societal contributions and hence are of great value. Data analytics involves many steps and one of the primary and the most important one is data pre-processing stage. Raw data has to be cleaned, stabilized and processed to a new form to make the analysis easier and correct. Many pre-processing tools are available but this paper specifically deals with the comparative study of two tools such as Rapid Miner and R programming language which are predominantly used by data analysts. The output of the paper gives an insight into the weightage of the particular tool which can be recommended for better data pre-processing.
Key-Words / Index Term
Data analytics, data pre-processing, noise removal, clean data, Rapid Miner, R programming
References
[1] D. Thornton, G. Van Capelleveen, M. Poel, J. Van Hillegersberg, and R. M. Mueller, “Outlier-based Health Insurance Fraud Detection for U.S. Medicaid Data,” Proc. 16th Int. Conf. Enterp. Inf. Syst., pp. 684–694, 2014.
[2] S. B. Kotsiantis, D. Kanellopoulos, and P. E. Pintelas, “Data preprocessing for supervised leaning,” Int. J. Comput. Sci., vol. 1, no. 1, pp. 111–117, 2006.
[3] D. P. Methods, “Data_Preprocessing.”
[4] T. H. Zolt´an Prekopcs´ak, G´abor Makrai and C. G´asp´ar-Papanek∗, “Radoop: Analyzing Big Data with RapidMiner and Hadoop.” http://www.iasri.res.in/ebook/win_school_aa/notes/Data_Preprocessing.pdf, “No Title.” .
[6] A. FAMILI, W. SHEN, R. WEBER, and E. SIMOUDIS, “Data preprocessing and intelligent data analysis,” Intell. Data Anal., vol. 1, no. 1–4, pp. 3–23, 1997.
[7] C. M. Teng, “Correcting noisy data,” Proc 16th Int. Conf Mach. Learn., pp. 239–248, 1999.
[8] K. Rangra and K. L. Bansal, “Comparative Study of Data Mining Tools,” Int. J. Adv. Res. Comput. Sci. Softw. Eng., vol. 4, no. 6, pp. 2277–128, 2014.
[9] https://en.wikipedia.org/wiki/RapidMiner, “No Title.” .
[10] Y. Ramamohan, K. Vasantharao, C. K. Chakravarti, and A. S. K. Ratnam, “A Study of Data Mining Tools in Knowledge Discovery Process,” Int. J. Soft Comput. Eng., 2012.
[11] Https://www.r-project.org/about.html, “No Title.” .
[12] A. Jović, K. Brkić, and N. Bogunović, “An overview of free software tools for general data mining,” 2014 37th Int. Conv. Inf. Commun. Technol. Electron. Microelectron. MIPRO 2014 - Proc., no. May, pp. 1112–1117, 2014.