Feature Dimension Reduction Using Euclidean Distance Oriented Similarity Based Rough Set Model
A.C. Mondal1 , S. Kolay2
Section:Research Paper, Product Type: Journal Paper
Volume-07 ,
Issue-01 , Page no. 33-36, Jan-2019
Online published on Jan 20, 2019
Copyright © A.C. Mondal, S. Kolay . This is an open access article distributed under the Creative Commons Attribution License, which permits unrestricted use, distribution, and reproduction in any medium, provided the original work is properly cited.
View this paper at Google Scholar | DPI Digital Library
How to Cite this Paper
- IEEE Citation
- MLA Citation
- APA Citation
- BibTex Citation
- RIS Citation
IEEE Style Citation: A.C. Mondal, S. Kolay, “Feature Dimension Reduction Using Euclidean Distance Oriented Similarity Based Rough Set Model,” International Journal of Computer Sciences and Engineering, Vol.07, Issue.01, pp.33-36, 2019.
MLA Style Citation: A.C. Mondal, S. Kolay "Feature Dimension Reduction Using Euclidean Distance Oriented Similarity Based Rough Set Model." International Journal of Computer Sciences and Engineering 07.01 (2019): 33-36.
APA Style Citation: A.C. Mondal, S. Kolay, (2019). Feature Dimension Reduction Using Euclidean Distance Oriented Similarity Based Rough Set Model. International Journal of Computer Sciences and Engineering, 07(01), 33-36.
BibTex Style Citation:
@article{Mondal_2019,
author = {A.C. Mondal, S. Kolay},
title = {Feature Dimension Reduction Using Euclidean Distance Oriented Similarity Based Rough Set Model},
journal = {International Journal of Computer Sciences and Engineering},
issue_date = {1 2019},
volume = {07},
Issue = {01},
month = {1},
year = {2019},
issn = {2347-2693},
pages = {33-36},
url = {https://www.ijcseonline.org/full_spl_paper_view.php?paper_id=588},
publisher = {IJCSE, Indore, INDIA},
}
RIS Style Citation:
TY - JOUR
UR - https://www.ijcseonline.org/full_spl_paper_view.php?paper_id=588
TI - Feature Dimension Reduction Using Euclidean Distance Oriented Similarity Based Rough Set Model
T2 - International Journal of Computer Sciences and Engineering
AU - A.C. Mondal, S. Kolay
PY - 2019
DA - 2019/01/20
PB - IJCSE, Indore, INDIA
SP - 33-36
IS - 01
VL - 07
SN - 2347-2693
ER -
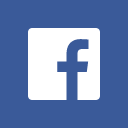
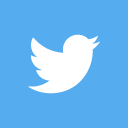
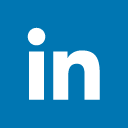
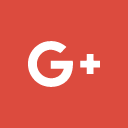
Abstract
In machine learning, a very high dimensional data reduces the performance of a classifier. To overcome this, a relevant feature dimension reduction algorithm can be applied before applying any classification algorithm. Rough set theory [1] is a very good tool to reduce the feature dimension of an information system or decision system. However, if a decision system contains real-valued data, we cannot apply directly the rough set theory. Various extensions to rough set can be used to handle this kind of data. Among them, Fuzzy-Rough set theory [2], similarity based rough set model [3] are interesting. We propose an algorithm for dimension reduction using Euclidean distance oriented similarity based rough set model. To show the effectiveness of the algorithm, we take Grammatical Facial Expression Dataset from UCI Machine Learning Repository, created by Freitas et al. [4] and applied KNN classifier before and after feature dimension reduction.
Key-Words / Index Term
Rough Set, Feature Dimension Reduction, Similarity Relation, KNN, Grammatical Facial Expression Recognition
References
[1] Z. Pawlak, “Rough Sets. Theoretical Aspects of Reasoning about Data”, Kluwer Academic Publishers, 1991.
[2] R. Jensen, Q. Shen, “Semantics-preserving dimensionality reduction: rough and fuzzy-rough-based approaches”, IEEE Transactions on Knowledge and Data Engineering, Vol.16, Issue.12, pp.1457-1471, 2004.
[3] J. Stepaniuk, “Similarity Based Rough Sets and Learning”, Proceedings of the Fourth International Workshop on Rough Sets, Fuzzy Sets, and Machine Discovery, Tokyo, Japan, pp. 18-22, 1996.
[4] F. A. Freitas, S. M.. Peres, C. A. M. Lima, F. V. Barbosa, “Grammatical Facial Expressions Recognition with Machine Learning”, 27th Florida Artificial Intelligence Research Society Conference (FLAIRS), Palo Alto, pp. 180-185, 2014.
[5] K. S. Ray, S. Kolay “Application of Approximate Equality for Reduction of Feature Vector Dimension”, Journal of Pattern Recognition Research, Vol.11, Issue. 1, pp.26-40, 2016