An Exhaustive Review of the Privacy Preservation and Security Mechanisms in Big Data Life Cycle
Manjula GS1 , T. Meyyappan2
Section:Review Paper, Product Type: Journal Paper
Volume-06 ,
Issue-11 , Page no. 76-83, Dec-2018
Online published on Dec 31, 2018
Copyright © Manjula GS, T. Meyyappan . This is an open access article distributed under the Creative Commons Attribution License, which permits unrestricted use, distribution, and reproduction in any medium, provided the original work is properly cited.
View this paper at Google Scholar | DPI Digital Library
How to Cite this Paper
- IEEE Citation
- MLA Citation
- APA Citation
- BibTex Citation
- RIS Citation
IEEE Style Citation: Manjula GS, T. Meyyappan, “An Exhaustive Review of the Privacy Preservation and Security Mechanisms in Big Data Life Cycle,” International Journal of Computer Sciences and Engineering, Vol.06, Issue.11, pp.76-83, 2018.
MLA Style Citation: Manjula GS, T. Meyyappan "An Exhaustive Review of the Privacy Preservation and Security Mechanisms in Big Data Life Cycle." International Journal of Computer Sciences and Engineering 06.11 (2018): 76-83.
APA Style Citation: Manjula GS, T. Meyyappan, (2018). An Exhaustive Review of the Privacy Preservation and Security Mechanisms in Big Data Life Cycle. International Journal of Computer Sciences and Engineering, 06(11), 76-83.
BibTex Style Citation:
@article{GS_2018,
author = {Manjula GS, T. Meyyappan},
title = {An Exhaustive Review of the Privacy Preservation and Security Mechanisms in Big Data Life Cycle},
journal = {International Journal of Computer Sciences and Engineering},
issue_date = {12 2018},
volume = {06},
Issue = {11},
month = {12},
year = {2018},
issn = {2347-2693},
pages = {76-83},
url = {https://www.ijcseonline.org/full_spl_paper_view.php?paper_id=544},
publisher = {IJCSE, Indore, INDIA},
}
RIS Style Citation:
TY - JOUR
UR - https://www.ijcseonline.org/full_spl_paper_view.php?paper_id=544
TI - An Exhaustive Review of the Privacy Preservation and Security Mechanisms in Big Data Life Cycle
T2 - International Journal of Computer Sciences and Engineering
AU - Manjula GS, T. Meyyappan
PY - 2018
DA - 2018/12/31
PB - IJCSE, Indore, INDIA
SP - 76-83
IS - 11
VL - 06
SN - 2347-2693
ER -
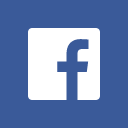
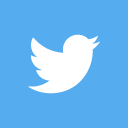
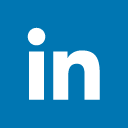
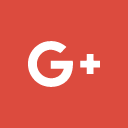
Abstract
As there is an exponential growth of data in every field of life, the assessment and extraction of data from the massive data sets has derived as a dreadful challenge in this golden era of big data. Conventional security methods cannot be adapted to big data due to its massive volume, and range. Undoubtedly, mining fruitful information from this massive data has been a universal interest for the organizations having large dataset. Big data life cycle includes three phases such as data generation, data storage, and data processing. In big data process, distributed systems are adapted since it needs large storage and high computational power. As many parties are engaged in these systems, the possibility of the violation in security concerns increases. In addition, since big data contains individual’s personal information, privacy is the foremost security concern. The main objective of this paper is to present an exhaustive overview of the privacy preservation mechanisms in big data life cycle. The modern privacy-preserving methods such as the generalization are capable of effectively managing the privacy assaults on a sole data set, whereas the protection of privacy for multiple data sets continues to be hard. Therefore, with intention of conserving the secrecy of multiple data sets, it is desirable to initially anonymize whole data sets and thereafter encrypt them before amassing or exchanging them in cloud. The challenges in existing mechanisms and eventual research discussions relevant to privacy preservation in big data are mentioned. The security techniques to protect the data set from being accessed by illegal users are also discussed.
Key-Words / Index Term
Big Data, Conventional, Data Privacy, Mining, Security
References
[1] NasrinIrshadHussain, BharadwajChoudhury, SandipRakshit, ―A Novel Method for Preserving Privacy in Big-Data Mining, International Journal of Computer Applications (0975 – 8887), Vol. 103, No. 16, pp. 22-25, October 2014.
[2] J. Manyika, M. Chui, B. Brown, J. Bughin, R. Dobbs, C. Roxburgh, and A. Byers, “Big data: The next frontier for innovation, competition, and productivity,” Mickensy Global Institute , pp. 1–137, Jun. 2011.
[3] B. Matturdi, X. Zhou, S, Li, and F. Lin, “Big data security and privacy: A review,” China
Communications, vol. 11, no. 14, pp. 135–145, Apr. 2014.
[4] P.Samarati, “Protecting respondent`s privacy in micro data release,” In IEEE Transaction on knowledge and Data Engineering, pp.010-027, 2001
[5] L. Xu, C. Jiang, J. Wang, J. Yuan, and Y. Ren, “Information security in big data: Privacy and data mining,” in IEEE Access, vol. 2, pp. 1149–1176, Oct. 2014.
[6] Chen, M.; Mao, S.; Liu, Y. Big data: A survey. Mob. Netw. Appl, Vol.19, pp. 171–209, 2014.
[7] Wenliang Du and Zhijun Zhan, “Using Randomized Response Tech-niques for Privacy-Preserving Data
Mining,” SIGKDD ’03, August 24-27, 2003, Washington, DC, USA.
[8] ArisGkoulalas-Divanis, &GrigoriosLoukides, “Revisiting Sequential Pattern Hiding to Enhance Utility”,
ACM, August 2011.
[9] Yehuda Lindell, Benny Pinkas, ―Secure Multiparty Computation for Privacy-Preserving Data Mining, The Journal of Privacy and Confiden-tiality, Vol. 1, no. 1,, pp. 1-39, 2009.
[10] B. Pinkas, ―Cryptographic techniques for privacy-preserving data mining, SIGKDD Explore, Vol. 4, no. 2, pp. 12-19, 2002.
[11] Pingshui WANG, Survey on Privacy Preserving Data Mining, International Journal of Digital Content
[12] 20 essential Hadoop tools for crunching Big Data [Online] availa-ble:http://bigdata-madesimple.com/20-essential-hadoop-tools-for-crunching-big-data/
[13] Akhil Mittal, “Trustworthiness of Big Data,” International Journal of Computer Applications (0975 –
8887), Vol. 80, no.9, October, 2013.
[14] Boris Glavic,“Big Data Provenance: Challenges and Implications for Benchmarking”, Specifying Big Data
Benchmarks,Vol. 8163, Springer Berlin Heidelberg, 2014.
[15] MogreNeha V., &PatilSulbha,“Slicing: An Approach for Privacy Preservation in High-Dimensional Data Using Anonymization Technique,”IRAJ International Conference, Pune 2013
[16] K.Anbazhagan, Dr.R.Sugumar, M.Mahendran, R.Natarajan, “An Efficient Approach for Statistical Anonymization Techniques for Privacy Preserving Data Mining,”International Journal of Advanced Re-search in Computer and Communication Engineering, Vol. 1, no. 7, pp. 482-485, September 2012.
[17] Y.H.Wu. C.Chiang and A.L.P.Chen. “Hiding Sensitive Association Rules with Limited Side Effects”,
IEEE Transaction on Knowledge and Data Engineering, Vol.19, no.1, pp 29-42, 2007
[18] GarimaSehgal, Dr. KanwalGarg “Comparison of Various Clustering Algorithms” (IJCSIT) International
Journal of Computer Science and Information Technologies, Vol. 5, no. 3, pp. 3074-3076, 2014.
[19] M.-Y. Lin, P.-Y. Lee, and S.-C. “Hsueh. Apriori-based frequent itemset mining algorithms on
MapReduce,”In Proc. ICUIMC, pages 26–30.ACM, 2012.
[20] Singh, Pravesh Kumar, and MohdShahid Husain. "Books Reviews using Naıve Bayes and Clustering lassifier." Second International Conference on Emerging Research in Computing, Information, Communication and Applications “ (ERCICA-2014), pp. 886-891, 2014.
[21] J. Domingo-Ferrer, D. Sanchez, and J. Soria-Comas. “Database anonymization: privacy models, data tility, and microaggregation- based inter-model connections,”. Morgan & Claypool, 2016
[22] L. Sweeney, "k-anonymity: A model for protecting privacy," International Journal on Uncertainty, Fuzziness and Knowledge-Based Systems, pp. 557–570, 2002.
[23] Khaled El Emam,&Fida Kamal Dankar, Protecting Privacy Using k-Anonymity, J Am Med Inform Assoc. Vol. 15, no. 5, pp. 627–637, 2008.
[24] N. Li, T. Li, S. Venkatasubramanian, "t-Closeness: Privacy Beyond k-Anonymity and l-Diversity, " IEEE 23rd International Conference on Data Engineering, 2007, pp. 106 - 115.
[25] Meraj Fatima , Dr. T.K.ShaikShavali , G. Kumar, “Strict Privacy with Enhanced Utility Preservation by T-Closeness Through Microaggregation,”Interrnational Journal of Advanced Technology and Innovative Research, Vol.08, no.15, pp. 3026-3035, October-2016.
[26] F. H. Cate, V. M. Schönberger, "Notice and Consent in a World of Big Data," Microsoft Global Privacy Summit Summary Report and Outcomes, Nov 2012.
[27] [27]Friedman A, Schuster A. Data mining with differential privacy. In: Proceedings of the 16th ACM SIGKDD international conference on Knowledge discovery and data mining, Washington, DC, USA, pp.25–28, July 2010.