Melanoma Skin Cancer Detection Using Improved RBF Classifier
K. Thenmozhi1
Section:Research Paper, Product Type: Journal Paper
Volume-06 ,
Issue-08 , Page no. 125-132, Oct-2018
CrossRef-DOI: https://doi.org/10.26438/ijcse/v6si8.125132
Online published on Oct 31, 2018
Copyright © K. Thenmozhi . This is an open access article distributed under the Creative Commons Attribution License, which permits unrestricted use, distribution, and reproduction in any medium, provided the original work is properly cited.
View this paper at Google Scholar | DPI Digital Library
How to Cite this Paper
- IEEE Citation
- MLA Citation
- APA Citation
- BibTex Citation
- RIS Citation
IEEE Style Citation: K. Thenmozhi, “Melanoma Skin Cancer Detection Using Improved RBF Classifier,” International Journal of Computer Sciences and Engineering, Vol.06, Issue.08, pp.125-132, 2018.
MLA Style Citation: K. Thenmozhi "Melanoma Skin Cancer Detection Using Improved RBF Classifier." International Journal of Computer Sciences and Engineering 06.08 (2018): 125-132.
APA Style Citation: K. Thenmozhi, (2018). Melanoma Skin Cancer Detection Using Improved RBF Classifier. International Journal of Computer Sciences and Engineering, 06(08), 125-132.
BibTex Style Citation:
@article{Thenmozhi_2018,
author = {K. Thenmozhi},
title = {Melanoma Skin Cancer Detection Using Improved RBF Classifier},
journal = {International Journal of Computer Sciences and Engineering},
issue_date = {10 2018},
volume = {06},
Issue = {08},
month = {10},
year = {2018},
issn = {2347-2693},
pages = {125-132},
url = {https://www.ijcseonline.org/full_spl_paper_view.php?paper_id=487},
doi = {https://doi.org/10.26438/ijcse/v6i8.125132}
publisher = {IJCSE, Indore, INDIA},
}
RIS Style Citation:
TY - JOUR
DO = {https://doi.org/10.26438/ijcse/v6i8.125132}
UR - https://www.ijcseonline.org/full_spl_paper_view.php?paper_id=487
TI - Melanoma Skin Cancer Detection Using Improved RBF Classifier
T2 - International Journal of Computer Sciences and Engineering
AU - K. Thenmozhi
PY - 2018
DA - 2018/10/31
PB - IJCSE, Indore, INDIA
SP - 125-132
IS - 08
VL - 06
SN - 2347-2693
ER -
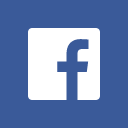
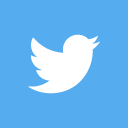
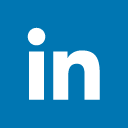
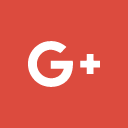
Abstract
Melanoma is a category of cancer that develops from the pigment-containing cells recognized as melanocytes. Melanomas usually ensue in the fur but may arise in the jaws, guts or ogle. This paper tends to two distinct frameworks for identification of skin growth in dermoscopy pictures. The primary framework utilizes worldwide strategies and the second framework utilizes neighborhood highlights and the classifier. Henceforth, melanoma is effortlessly to distinguish utilizing with help of worldwide strategies and neighborhood highlights. Skin Disease prediction has become important in a variety of applications such as health insurance, tailored health communication and public health. Due to the costs for dermatologists to monitor every patient, there is a need for an computerized system to evaluate a patient’s risk of melanoma using images of their skin lesions captured using a standard digital camera. The traditional diagnosis technique aims at improving the quality of existing diagnostic systems by proposing advanced feature selection and classification methods.RBF neural network derives classification. For this classification (RBF neural network)this paper proposed new learning method using K means clustering. This paper focuses on the detection of skin lesion as a literature survey.
Key-Words / Index Term
Dermoscopy, Melanoma , Neural network, Clustering, Classification
References
[1]. TaisiaHuckle, and Karl Parker, Long-Term Impact on Alcohol-Involved Crashes of Lowering the Minimum Purchase Age in New Zealand, American Journal of Public Health, Vol. 104, pp.1087-1091,June 2014.
[2]. Messadi M, Ammar M, Cherifi H, Chikh MA and Bessaid A,” Interpretable Aide Diagnosis System for Melanoma Recognition”, 2014, Bioengineering & Biomedical Science.
[3]. Eduardo López-Caneda, Socorro Rodríguez Holguín, Montserrat Corral, Sonia Doallo, Fernando Cadaveira, Evolution of the binge drinking pattern in college students: Neurophysiological correlates, Alcohol (Elsevier) Vol. 48 , 2014.
[4]. Angelina Pilatti , Juan Carlos Godoy , SilvinaBrussino , Ricardo Marcos Pautassi,” Underage drinking: Prevalence and risk factors associated with drinking experiences among Argentinean children”, Alcohol( Elsevier), Vol. 47, pp. 323- 331, 2013.
[5]. Nadia Smaoui, SouhirBessassi,” A developed system for melanoma diagnosis”,2013, International Journal of Computer Vision and Signal Processing, 3(1), 10-17(2013).
[6]. Teresa Mendonca, Pedro M. Ferreira,Jorge S. Marques, Andre R. S. Marcal, Jorge Rozeira,” PH2 - A dermoscopic image database for research and benchmarking”,2013, 35th Annual International Conference of the IEEE EMBS Osaka, Japan, 3 - 7 July, 2013.
[7]. Angel Alfonso Cruz-Roa, John Edison ArevaloOvalle, AnantMadabhushi, and Fabio Augusto Gonz´alez Osorio,” A Deep Learning Architecture for Image Representation, Visual Interpretability and Automated Basal-Cell Carcinoma Cancer Detection”,2013.
[8]. Mahmoud Elgamal ,” AUTOMATIC SKIN CANCER IMAGES CLASSIFICATION”, 2013, (IJACSA) International Journal of Advanced Computer Science and Applications, Vol. 4, No. 3, 2013.
[9]. G. Ravi Kumar, Dr. G. A. Ramachandra, K.Nagamani,” An Efficient Prediction of Breast Cancer Data using Data Mining Techniques”, International Journal of Innovations in Engineering and Technology (IJIET),Vol. 2 ,No. 4, pp139- 144, August 2013.
[10]. Kawsar Ahmed, Abdullah Al Emran, TasnubaJesmin, Roushney Fatima Mukti,MdZamilurRahman, Farzana Ahmed,” Early Detection of Lung Cancer Risk Using Data Mining”, Asian Pacific Journal of Cancer Prevention, Vol. 14,pp. 595-.2014