Enhanced GMD Technique for Segmentation of Yarn Images based on Parametric Value and Intensity Gradient Analysis
R. Sudha Muthusamy1 , V. Chitraa2
Section:Research Paper, Product Type: Journal Paper
Volume-06 ,
Issue-08 , Page no. 56-63, Oct-2018
CrossRef-DOI: https://doi.org/10.26438/ijcse/v6si8.5663
Online published on Oct 31, 2018
Copyright © R. Sudha Muthusamy, V. Chitraa . This is an open access article distributed under the Creative Commons Attribution License, which permits unrestricted use, distribution, and reproduction in any medium, provided the original work is properly cited.
View this paper at Google Scholar | DPI Digital Library
How to Cite this Paper
- IEEE Citation
- MLA Citation
- APA Citation
- BibTex Citation
- RIS Citation
IEEE Style Citation: R. Sudha Muthusamy, V. Chitraa, “Enhanced GMD Technique for Segmentation of Yarn Images based on Parametric Value and Intensity Gradient Analysis,” International Journal of Computer Sciences and Engineering, Vol.06, Issue.08, pp.56-63, 2018.
MLA Style Citation: R. Sudha Muthusamy, V. Chitraa "Enhanced GMD Technique for Segmentation of Yarn Images based on Parametric Value and Intensity Gradient Analysis." International Journal of Computer Sciences and Engineering 06.08 (2018): 56-63.
APA Style Citation: R. Sudha Muthusamy, V. Chitraa, (2018). Enhanced GMD Technique for Segmentation of Yarn Images based on Parametric Value and Intensity Gradient Analysis. International Journal of Computer Sciences and Engineering, 06(08), 56-63.
BibTex Style Citation:
@article{Muthusamy_2018,
author = {R. Sudha Muthusamy, V. Chitraa},
title = {Enhanced GMD Technique for Segmentation of Yarn Images based on Parametric Value and Intensity Gradient Analysis},
journal = {International Journal of Computer Sciences and Engineering},
issue_date = {10 2018},
volume = {06},
Issue = {08},
month = {10},
year = {2018},
issn = {2347-2693},
pages = {56-63},
url = {https://www.ijcseonline.org/full_spl_paper_view.php?paper_id=475},
doi = {https://doi.org/10.26438/ijcse/v6i8.5663}
publisher = {IJCSE, Indore, INDIA},
}
RIS Style Citation:
TY - JOUR
DO = {https://doi.org/10.26438/ijcse/v6i8.5663}
UR - https://www.ijcseonline.org/full_spl_paper_view.php?paper_id=475
TI - Enhanced GMD Technique for Segmentation of Yarn Images based on Parametric Value and Intensity Gradient Analysis
T2 - International Journal of Computer Sciences and Engineering
AU - R. Sudha Muthusamy, V. Chitraa
PY - 2018
DA - 2018/10/31
PB - IJCSE, Indore, INDIA
SP - 56-63
IS - 08
VL - 06
SN - 2347-2693
ER -
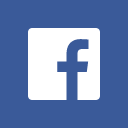
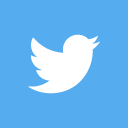
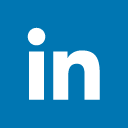
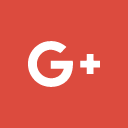
Abstract
Because of the approach of computer based innovation image processing strategies have turned out to be progressively vital in a wide assortment of utilizations. Segmentation is an exemplary subject in the field of image processing. Many works are existing in the area of segmentation and these systems regularly must be joined with area of learning new innovations techniques to achieve the end goal to adequately tackle the segmentation issue. This paper discuss the issue of segmenting yarn image with fuzzy and coherence techniques. The point of segmentation in the considered application is to remove yarn core from the yarn. The technique is guided and compelled by Coherence Enhancing Diffusion (CED) and FCM (Fuzzy C-Means) channel and furthermore the main problem of the yarn image segmentation is considered. For the process of segmentation Gaussian mixture model in enhanced with coherence and fuzzy to acquire a division limit esteem. The Results of segmentation by the CED, FCM and proposed strategy GMD are compared. The correlation demonstrates that the proposed method gives best outcomes. The yarn core and the hairiness segmentation from the proposed algorithm are adequate for assurance of yarn properties performed in the accompanying strides of the estimations of yarn hairiness measurements.
Key-Words / Index Term
Coherence Enhancing Diffusion, Fuzzy C-Means,GMD, Yarn Segmentation, Yarn Hairiness
References
[1]. Kim YK, Langley KD, Avsar F. Quantita¬tive grading of spun yarns for appear¬ ance. Journal of Textile Engineering 2006; 52:13-14.
[2]. Zhong P, Zhang K, Han S, Hu R, Pang JY, Zhang XY, Huang FX. Evaluation method for yarn diameter uneven¬ness based on image sequence pro¬cessing. Textile Research Journal 2014; Advance online publication. doi: 10.1177/0040517514547211.
[3]. Li ZJ, Pan RR, Gao WD. Formation of digital yarn black board using sequence images. Textile Research Journal 2015; Advance online publication. doi: 10.1177/0040517514563725.
[4]. Eldessouki M, Ibrahim S, Militky J. A dynamic and robust image processing based method for measuring the yarn di¬ameter and its variation. Textile Research Journal 2014; Advance online publica¬tion. doi: 10.1177/0040517514530032.
[5] USTER ®, http://www.uster.com/UI/textile-TESTER-5-2-311.aspx, accessed on May 2010.
[6] V. Carvalho, P. Cardoso, M. Belsley, R. Vasconcelos, and F. Soares:“Development of a Yarn Evenness Measurement and Hairiness Analysis System”, IECON`06, The 32nd Annual Conference of the IEEEIndustrial Society, Paris, France, pp. 3621-3626, 2006.
[7]. M. Kuzański: “Measurement Methods for Yarn Hairiness Analysis - TheIdea and Construction of Research Standing”, International Conference MEMSTECH’2006 - Perspective Technologies and Methods in Mems Design, Lviv-Polyana, Ukraine, pp. 87-90, 2006.
[8] Y. A. Ozkaya, M. Acar, and M. R. Jackson: “Computer Vision for Yarn Hairiness and Irregularity Assessment”, ESDA’2002, 6th Biennial Conference on Engineering Systems Design and Analysis Istanbul,Turkey, pp.-, 2002.
[9] S. Pateria: “Design of a New Yarn Hairiness Tester”, M.Sc. Thesis,Department of Mechanical Engineering ,Indian Institute of Technology, Bombay, 2005.
[10] J. Weickert: “Anisotropic Diffusion in Image Processing”, ECMI Series,Teubner-Verlag, Germany, 1998, http://www.mia.uni-saarland.de/ weickert/book.html, accessed on January 2010.
[11] J. Weickert: “Coherence-Enhancing Diffusion Filtering”, International Journal of Computer Vision, vol. 31, pp. 111-127, 1999.
[12] N. Otsu: “A threshold selection method from gray-level histograms”. IEEE Trans. Systems, Man, and Cybernetics, vol. 9, no. 1, pp. 62-66.1979.
[13] P. K. Sahoo, S. Soltani, K. C. Wong, Y. C. Chen: “A Survey of Thresholding Techniques”, Computer Vision, Graphics, and Image Processing, vol. 41, pp. 233-260, 1988.
[14] Zhongjian Li,Ruru Pan, Jing’an Wang,Ziyu Wang, Bianbian Li,Weidong Gao” Real-time Segmentation of Yarn Images Based on an FCM Algorithm and Intensity Gradient Analysis” School of Textiles and Clothing, Jiangnan University, Wuxi 214122, China
[15] Benferhat S, Dubois D, Prade H (1997) Some syntactic approaches to the handling of inconsistent knowledge bases: a comparative study,part 1 :the flat case. Studia Logica 58: 17–45MATHCrossRefMathSciNetGoogle Scholar
[16] Bovens L, Hartmann S (2003) Bayesian epistemology. Oxford University Press, OxfordMATHGoogle Scholar
[17] Bovens L, Olsson E (2000) Coherence, reliability and Bayesian networks. Mind 109: 685–719CrossRefMathSciNetGoogle Scholar
[18] Chen S, Yeh M, Hsiao P (1995) A comparison of similarity measures of fuzzy values. Fuzzy Sets Syst 72: 79–89CrossRefMathSciNetGoogle Scholar
[19] Dubois D, Prade H (1980) Fuzzy set and systems. Academic Press, New YorkGoogle Scholar
[20] Fitelson B (2003) A probabilistic theory of coherence. Analysis 63: 194–199MATHCrossRefMathSciNetGoogle Scholar
[21] Gärdenfors P (1988) Knowledge in flux: modeling the dynamics of epistemic states. MIT Press, CambridgeGoogle Scholar
[22] Glass DH (2002) Coherence, explanation and Bayesian networks. In: Proceedings of the 13th Irish conference on AI and cognitive science. LNAI, vol 2464, pp 177–182Google Scholar
[23] Glass DH (2005) Problems with priors in probabilistic measures of coherence. Erkenntnis 63: 375–385MATHCrossRefMathSciNetGoogle Scholar
[24] Hunter A (2000) Reasoning with inconsistency using quasi-classical logic. J Logic Comput 10: 677–703MATHCrossRefMathSciNetGoogle Scholar
[25] Hunter A (2002) Measuring inconsistency in knowledge via quasi-classical methods. In: Proceedings of the 18th American national conference on AI (AAAI’02), pp 68–73Google Scholar
[26] Hunter A (2004) Making argumentation more believable. In: Proceedings of the 19th American national conference on AI (AAAI’04), pp 269–274Google Scholar
[27] Hunter A, Konieczny S (2004) Approaches to measuring inconsistent information. In: Inconsistency tolerance. LNCS, vol 3300, pp 189–234Google Scholar
[28] Kemeny J, Oppenheim P (1952) Degrees of factual support. Philos Sci 19: 307–324CrossRefGoogle Scholar
[29] Knight KM (2001) Measuring inconsistency. J Philos Logic 31: 77–98CrossRefMathSciNetGoogle Scholar
[30] Knight KM (2003) Two information measures for inconsistent sets. J Logic Lang Inf 12: 227–248MATHCrossRefMathSciNetGoogle Scholar
[31] Konieczny S, Lang J, Marquis P (2003) Quantifying information and contradiction in propositional logic through test actions. In: Proceedings of the 18th international joint conference on AI (IJCAI’03), pp 106–111Google Scholar
[32] Lozinskii E (1994) Information and evidence in logic systems. J Exp Theor Artif Intell 6: 163–193MATHCrossRefGoogle Scholar
[33] Moretti L, Akiba K (2007) Probabilistic measures of coherence and the problem of belief individuation. Synthese 154(1): 73–95MATHCrossRefMathSciNetGoogle Scholar
[34] Olsson EJ (2002) What is the problem of coherence and truth?. J Philos 99: 246–272MathSciNetGoogle Scholar
[35] Olsson EJ (2005) Against coherence. Oxford University Press, OxfordGoogle Scholar
[36] Priest G (2002) Paraconsistent logic. In: Gabbay DM, Guenther F (eds) Handbook of philosophical logic, vol 6. SpringerGoogle Scholar
[37] Qi G, Liu W, Bell DA (2005) Measuring conflict and agreement between two prioritized belief bases. In: Proceedings of the 18th international joint conference on AI (IJCAI’05), pp 552–558Google Scholar
[38] Sancho-Royo A, Verdegay JL (2000) Coherence measures on finite fuzzy sets. Int J Uncertain Fuzziness Knowl Based Syst 8: 641–663MATHMathSciNetGoogle Scholar
[39] Sancho-Royo A, Verdegay JL (2005) Fuzzy coherence measures. Int J Intell Syst 20: 1–11MATHCrossRefGoogle Scholar
[40] Shogenji T (1999) Is coherence truth-conducive?. Analysis 59: 338–345CrossRefGoogle Scholar
[41] Shogenji T (2001) Reply to Akiba on the probabilistic measure of coherence. Analysis 61: 144–150CrossRefMathSciNetGoogle Scholar
[42] Wang X, Baets BD, Kerre E (1995) A comparative study of similarity measures. Fuzzy Sets Syst 73: 259–268MATHCrossRefGoogle Scholar