Energy Prediction Using Data Analytics in Smart Grid
Panchami Anil1 , Anas P V2 , Naseef Kuruvakkottil3 , Anusha K V4 , Balagopal N5
Section:Research Paper, Product Type: Journal Paper
Volume-06 ,
Issue-06 , Page no. 110-115, Jul-2018
CrossRef-DOI: https://doi.org/10.26438/ijcse/v6si6.110115
Online published on Jul 31, 2018
Copyright © Panchami Anil, Anas P V, Naseef Kuruvakkottil, Anusha K V, Balagopal N . This is an open access article distributed under the Creative Commons Attribution License, which permits unrestricted use, distribution, and reproduction in any medium, provided the original work is properly cited.
View this paper at Google Scholar | DPI Digital Library
How to Cite this Paper
- IEEE Citation
- MLA Citation
- APA Citation
- BibTex Citation
- RIS Citation
IEEE Style Citation: Panchami Anil, Anas P V, Naseef Kuruvakkottil, Anusha K V, Balagopal N, “Energy Prediction Using Data Analytics in Smart Grid,” International Journal of Computer Sciences and Engineering, Vol.06, Issue.06, pp.110-115, 2018.
MLA Style Citation: Panchami Anil, Anas P V, Naseef Kuruvakkottil, Anusha K V, Balagopal N "Energy Prediction Using Data Analytics in Smart Grid." International Journal of Computer Sciences and Engineering 06.06 (2018): 110-115.
APA Style Citation: Panchami Anil, Anas P V, Naseef Kuruvakkottil, Anusha K V, Balagopal N, (2018). Energy Prediction Using Data Analytics in Smart Grid. International Journal of Computer Sciences and Engineering, 06(06), 110-115.
BibTex Style Citation:
@article{Anil_2018,
author = {Panchami Anil, Anas P V, Naseef Kuruvakkottil, Anusha K V, Balagopal N},
title = {Energy Prediction Using Data Analytics in Smart Grid},
journal = {International Journal of Computer Sciences and Engineering},
issue_date = {7 2018},
volume = {06},
Issue = {06},
month = {7},
year = {2018},
issn = {2347-2693},
pages = {110-115},
url = {https://www.ijcseonline.org/full_spl_paper_view.php?paper_id=456},
doi = {https://doi.org/10.26438/ijcse/v6i6.110115}
publisher = {IJCSE, Indore, INDIA},
}
RIS Style Citation:
TY - JOUR
DO = {https://doi.org/10.26438/ijcse/v6i6.110115}
UR - https://www.ijcseonline.org/full_spl_paper_view.php?paper_id=456
TI - Energy Prediction Using Data Analytics in Smart Grid
T2 - International Journal of Computer Sciences and Engineering
AU - Panchami Anil, Anas P V, Naseef Kuruvakkottil, Anusha K V, Balagopal N
PY - 2018
DA - 2018/07/31
PB - IJCSE, Indore, INDIA
SP - 110-115
IS - 06
VL - 06
SN - 2347-2693
ER -
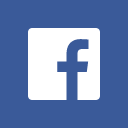
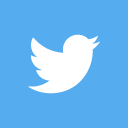
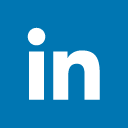
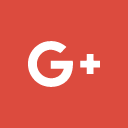
Abstract
A fully automated systemwhere embedded large pools of sensors in the existing electricity grid systems for monitoring and controlling it by making use of modern information technology is what is known as Smart Grid. By deriving and processing new information from these data in real time it can be made more applicable. Energy consumption prediction, which is a significant part of smart grid, may be difficult to handle with huge energy usage data in the grid. This is because the redundancy from feature selection cannot be avoided. Our aim is to predict the commercial energy consumption by a building based on its previous consumption history. First, we apply a correlation based feature selection method in order to filter out the most relevant attributes. Out of the resulting dataset so formed, for the purpose of dimensionality reduction we use a Kernel Principle Component Analysis methodology. What we obtain will be a set of principal components which will be our new dataset. To predict the energy usage, we use a Support Vector Regression method that uses kernel technique that determines a suitable point as the predicted value. Finally, we evaluate the performance of the predictor based on different evaluators to understand the efficiency of the technique.
Key-Words / Index Term
Smart Grid, Energy Consumption, Correlation Based Feature Selection, Kernel Principal Component Analysis, Support Vector Regression, Prediction
References
[1] Nagi, Jea, K. S. Yap, S. K. Tiong, S. K. Ahmed, and A. M. Mohammad. "Detection of abnormalities and electricity theft using genetic support vector machines." TENCON 2008-2008 IEEE Region 10 Conference, pp. 1-6. IEEE, 2008
[2] Angelos, Eduardo Werley S., Osvaldo R. Saavedra, Omar A. Carmona Cortés, and André Nunes de Souza. "Detection and identification of abnormalities in customer consumptions in power distribution systems." IEEE Transactions on Power Delivery 26, no. 4 (2011): 2436-2442.
[3] Costa, Breno C., Bruno LA Alberto, André M. Portela, W. Maduro, and Esdras O. Eler. "Fraud detection in electric power distribution networks using an ANN-based knowledge-discovery process." International Journal of Artificial Intelligence & Applications 4, no. 6 (2013): 17.
[4] Bu, Li, Dongbin Zhao, Yu Liu, and Qiang Guan. "A hierarchical classification algorithm for evaluating energy consumption behaviors." Neural Networks (IJCNN), 2014 International Joint Conference on, pp. 1461-1466. IEEE, 2014.
[5] Mayhew, Michael, Michael Atighetchi, Aaron Adler, and Rachel Greenstadt. "Use of machine learning in big data analytics for insider threat detection." Military Communications Conference, MILCOM 2015-2015 IEEE, pp. 915-922. IEEE, 2015.
[6] Jindal, Anish, Amit Dua, Kuljeet Kaur, Mukesh Singh, Neeraj Kumar, and S. Mishra. "Decision tree and SVM-based data analytics for theft detection in smart grid." IEEE Transactions on Industrial Informatics 12, no. 3 (2016): 1005-1016.
[7] Haben, Stephen, Colin Singleton, and Peter Grindrod. "Analysis and clustering of residential customers energy behavioral demand using smart meter data." IEEE transactions on smart grid 7, no. 1 (2016): 136-144.
[8] MCA, Mrs J. Sukanya. "Applications of Big Data Analytics and Machine Learning Techniques in Health Care Sectors." International Journal Of Engineering And Computer Science 6, no. 7 (2017).
[9] Wang, Kun, Chenhan Xu, Yan Zhang, Song Guo, and Albert Zomaya. "Robust big data analytics for electricity price forecasting in the smart grid." IEEE Transactions on Big Data(2017).
[10] Wahid, Fazli, RozaidaGhazali, Abdul Salam Shah, and Muhammad Fayaz. "Prediction of energy consumption in the buildings using multi-layer perceptron and random forest." IJAST 101 (2017): 13-22.
[11] Xuemei, Li, Ding Lixing, LvJinhu, Xu Gang, and Li Jibin. "A novel hybrid approach of kpca and svm for building cooling load prediction." Knowledge Discovery and Data Mining, 2010. WKDD`10. Third International Conference on, pp. 522-526. IEEE, 2010.
[12] Dubey, Vimal Kumar, and Amit Kumar Saxena. "Hybrid classification model of correlation-based feature selection and support vector machine." Current Trends in Advanced Computing (ICCTAC), IEEE International Conference on, pp. 1-6. IEEE, 2016.
[13] Ince, Huseyin, and Theodore B. Trafalis. "Kernel principal component analysis and support vector machines for stock price prediction." IIE Transactions 39, no. 6 (2007): 629-637.
[14] Michalak, Krzysztof, and Halina Kwasnicka. "Correlation-based feature selection strategy in neural classification." Intelligent Systems Design and Applications, 2006. ISDA`06. Sixth International Conference on, vol. 1, pp. 741-746. IEEE, 2006.
[15] Kallas, Maya, Gilles Mourot, Didier Maquin, and José Ragot. "Fault estimation of nonlinear processes using kernel principal component analysis." Control Conference (ECC), 2015 European, pp. 3197-3202. IEEE, 2015.