Extractive Approaches for Automatic Text Summarization
S. Gandotra1 , B. Arora2
Section:Review Paper, Product Type: Journal Paper
Volume-06 ,
Issue-03 , Page no. 40-80, Apr-2018
CrossRef-DOI: https://doi.org/10.26438/ijcse/v6si3.4080
Online published on Apr 30, 2018
Copyright © S. Gandotra, B. Arora . This is an open access article distributed under the Creative Commons Attribution License, which permits unrestricted use, distribution, and reproduction in any medium, provided the original work is properly cited.
View this paper at Google Scholar | DPI Digital Library
How to Cite this Paper
- IEEE Citation
- MLA Citation
- APA Citation
- BibTex Citation
- RIS Citation
IEEE Style Citation: S. Gandotra, B. Arora, “Extractive Approaches for Automatic Text Summarization,” International Journal of Computer Sciences and Engineering, Vol.06, Issue.03, pp.40-80, 2018.
MLA Style Citation: S. Gandotra, B. Arora "Extractive Approaches for Automatic Text Summarization." International Journal of Computer Sciences and Engineering 06.03 (2018): 40-80.
APA Style Citation: S. Gandotra, B. Arora, (2018). Extractive Approaches for Automatic Text Summarization. International Journal of Computer Sciences and Engineering, 06(03), 40-80.
BibTex Style Citation:
@article{Gandotra_2018,
author = {S. Gandotra, B. Arora},
title = {Extractive Approaches for Automatic Text Summarization},
journal = {International Journal of Computer Sciences and Engineering},
issue_date = {4 2018},
volume = {06},
Issue = {03},
month = {4},
year = {2018},
issn = {2347-2693},
pages = {40-80},
url = {https://www.ijcseonline.org/full_spl_paper_view.php?paper_id=322},
doi = {https://doi.org/10.26438/ijcse/v6i3.4080}
publisher = {IJCSE, Indore, INDIA},
}
RIS Style Citation:
TY - JOUR
DO = {https://doi.org/10.26438/ijcse/v6i3.4080}
UR - https://www.ijcseonline.org/full_spl_paper_view.php?paper_id=322
TI - Extractive Approaches for Automatic Text Summarization
T2 - International Journal of Computer Sciences and Engineering
AU - S. Gandotra, B. Arora
PY - 2018
DA - 2018/04/30
PB - IJCSE, Indore, INDIA
SP - 40-80
IS - 03
VL - 06
SN - 2347-2693
ER -
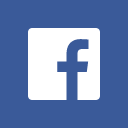
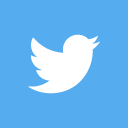
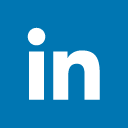
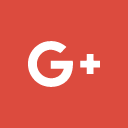
Abstract
With the flooding of a huge amount of data on the web with reference to the text, a technique to condense this data in summary form is very important so that the users can have access to relevant information regardless of enormous content on the web that is available to the user. This content could be informative, relevant or even important to the user or could be purely irrelevant. Text summarization techniques help in reducing the time and effort of the user looking for content about a particular topic on the internet by summarizing the content of the documents and the user by only looking at the summarized content can decide whether the document is relevant or irrelevant. Thus, automatic text summarization techniques play a key role in information retrieval from the web. In this paper, a study of various text summarization techniques has been conducted based on parameters like a number of documents, content, output, language, availability of training data etc. Also, the summary evaluation processes i.e. intrinsic and extrinsic are discussed. Extractive approaches for text summarization are also discussed and the recent work done in each of these approaches is compared and contrasted.
Key-Words / Index Term
Text Summarization, Extractive summarization, Intrinsic Evaluation, Extrinsic Evaluation
References
[1] H. P. Luhn, “The Automatic Creation of Literature Abstracts,” IBM J. Res. Dev., vol. 2, no. 2, pp. 159–165, 1958.
[2] M. Gambhir and V. Gupta, “Recent automatic text summarization techniques :,” Artif. Intell. Rev., vol. 47, no. 1, pp. 1–66, 2017.
[3] M. Hassel, “Evaluation of Automatic Text Summarization.”
[4] “Extractive Summarization - Google Search.” [Online]. Available: https://www.google.co.in/search?q=Extractive+Summarization&oq=Extractive+&aqs=chrome.1.69i57j69i59j69i61l3j0.7516j0j7&sourceid=chrome&ie=UTF-8. [Accessed: 25-Feb-2018].
[5] V. K. Gupta and T. J. Siddiqui, “Multi-document summarization using sentence clustering,” in 2012 4th International Conference on Intelligent Human Computer Interaction (IHCI), 2012, pp. 1–5.
[6] Xiao-Ying Liu, Yi-Ming Zhou, and Ruo-Shi Zheng, “Measuring semantic similarity within sentences,” in 2008 International Conference on Machine Learning and Cybernetics, 2008, pp. 2558–2562.
[7] R. Ferreira et al., “Assessing sentence scoring techniques for extractive text summarization,” 2013.
[8] A. N. Gulati and S. D. Sawarkar, “A novel technique for multidocument Hindi text summarization,” 2017 Int. Conf. Nascent Technol. Eng. ICNTE 2017 - Proc., 2017.
[9] L. Yang, X. Cai, S. Pan, H. Dai, and D. Mu, “Multi-document summarization based on sentence cluster using non-negative matrix factorization,” J. Intell. Fuzzy Syst., vol. 33, no. 3, pp. 1867–1879, 2017.
[10] G. Yang, D. Wen, Kinshuk, N.-S. Chen, and E. Sutinen, “A novel contextual topic model for multi-document summarization,” Expert Syst. Appl., vol. 42, no. 3, pp. 1340–1352, Feb. 2015.
[11] Z. Wu et al., “A topic modeling based approach to novel document automatic summarization,” Expert Syst. Appl., vol. 84, pp. 12–23, 2017.
[12] E. Baralis, L. Cagliero, N. Mahoto, and A. Fiori, “GRAPHSUM: Discovering correlations among multiple terms for graph-based summarization,” 2013.
[13] S. alZahir, Q. Fatima, and M. Cenek, “New graph-based text summarization method,” in 2015 IEEE Pacific Rim Conference on Communications, Computers and Signal Processing (PACRIM), 2015, pp. 396–401.
[14] C. Fang, D. Mu, Z. Deng, and Z. Wu, “Word-sentence co-ranking for automatic extractive text summarization,” Expert Syst. Appl., vol. 72, pp. 189–195, Apr. 2017.
[15] M. A. Fattah and F. Ren, “GA, MR, FFNN, PNN and GMM based models for automatic text summarization,” Comput. Speech Lang., vol. 23, no. 1, pp. 126–144, Jan. 2009.
[16] L. Yang, X. Cai, Y. Zhang, and P. Shi, “Enhancing sentence-level clustering with ranking-based clustering framework for theme-based summarization,” Inf. Sci. (Ny)., vol. 260, pp. 37–50, Mar. 2014.
[17] W. Li, W.-K. Ng, Y. Liu, and K.-L. Ong, “Enhancing the Effectiveness of Clustering with Spectra Analysis,” IEEE Trans. Knowl. Data Eng., vol. 19, no. 7, pp. 887–902, Jul. 2007.
[18] N. Desai and P. Shah, “AUTOMATIC TEXT SUMMARIZATION USING SUPERVISED MACHINE LEARNING TECHNIQUE FOR HINDI LANGAUGE.”