Feature Extraction Techniques in Hyper Spectral Data Sets for Classification Purpose
Anuj Rapaka1 , Arul Murgan Ramu2
Section:Survey Paper, Product Type: Journal Paper
Volume-07 ,
Issue-16 , Page no. 129-135, May-2019
CrossRef-DOI: https://doi.org/10.26438/ijcse/v7si16.129135
Online published on May 18, 2019
Copyright © Anuj Rapaka, Arul Murgan Ramu . This is an open access article distributed under the Creative Commons Attribution License, which permits unrestricted use, distribution, and reproduction in any medium, provided the original work is properly cited.
View this paper at Google Scholar | DPI Digital Library
How to Cite this Paper
- IEEE Citation
- MLA Citation
- APA Citation
- BibTex Citation
- RIS Citation
IEEE Style Citation: Anuj Rapaka, Arul Murgan Ramu, “Feature Extraction Techniques in Hyper Spectral Data Sets for Classification Purpose,” International Journal of Computer Sciences and Engineering, Vol.07, Issue.16, pp.129-135, 2019.
MLA Style Citation: Anuj Rapaka, Arul Murgan Ramu "Feature Extraction Techniques in Hyper Spectral Data Sets for Classification Purpose." International Journal of Computer Sciences and Engineering 07.16 (2019): 129-135.
APA Style Citation: Anuj Rapaka, Arul Murgan Ramu, (2019). Feature Extraction Techniques in Hyper Spectral Data Sets for Classification Purpose. International Journal of Computer Sciences and Engineering, 07(16), 129-135.
BibTex Style Citation:
@article{Rapaka_2019,
author = {Anuj Rapaka, Arul Murgan Ramu},
title = {Feature Extraction Techniques in Hyper Spectral Data Sets for Classification Purpose},
journal = {International Journal of Computer Sciences and Engineering},
issue_date = {5 2019},
volume = {07},
Issue = {16},
month = {5},
year = {2019},
issn = {2347-2693},
pages = {129-135},
url = {https://www.ijcseonline.org/full_spl_paper_view.php?paper_id=1293},
doi = {https://doi.org/10.26438/ijcse/v7i16.129135}
publisher = {IJCSE, Indore, INDIA},
}
RIS Style Citation:
TY - JOUR
DO = {https://doi.org/10.26438/ijcse/v7i16.129135}
UR - https://www.ijcseonline.org/full_spl_paper_view.php?paper_id=1293
TI - Feature Extraction Techniques in Hyper Spectral Data Sets for Classification Purpose
T2 - International Journal of Computer Sciences and Engineering
AU - Anuj Rapaka, Arul Murgan Ramu
PY - 2019
DA - 2019/05/18
PB - IJCSE, Indore, INDIA
SP - 129-135
IS - 16
VL - 07
SN - 2347-2693
ER -
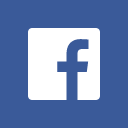
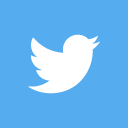
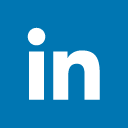
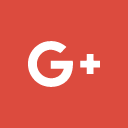
Abstract
A critical increment for utilization of proximal/remote hyper spectral imaging frameworks to contemplate plant properties, types, and conditions. Various budgetary and ecological advantages of utilizing such frameworks have been the driving constrain inside this development. This paper is worried about the examination of hyper spectral information for identifying plant sicknesses and stress conditions and ordering crop types by methods for cutting edge machine learning strategies. Primary commitment of the work lies in the utilization of an inventive order system for the examination, in which versatile component choice, curiosity recognition, and troupe learning are coordinated. Three hyper spectral datasets and a non-imaging hyper spectral dataset were utilized in the assessment of the proposed structure. Show critical upgrades accomplished by the proposed technique contrasted with the utilization of exact ghastly lists and existing arrangement techniques.
Key-Words / Index Term
Hyper spectral Data imaging, ND, plant monitoring, remote sensing, SVM
References
[1] A. F. Goetz, G. Vane, J. E. Solomon, and B. N. Shake, "Imaging spectrometry for earth remote detecting," Science, vol. 228, no. 4704, pp. 1147– 1153,1985.
[2] P. L. M. Geladi, H. F. Grahn, and J. E. Burger, "Multivariate pictures, hyper spectral imaging: Background and gear," in Techniques and Uses of Hyperspectral Image Analysis. New York, NY, USA: Wiley, 2007, pp. 1– 15.
[3] G. ElMasry and D.- W. Sun, "Section 1: Principles of hyper spectral imaging innovation," in Hyperspectral Imaging for Food Quality Analysis and Control, D.- W. Sun, Ed. San Diego, CA, USA: Academic, 2010, pp. 3– 43.
[4] J. Campbell and R. Wynne, Introduction to Remote Sensing, fifth ed. New York, NY, USA: Guilford Publications, 2011.
[5] A. Cracknell and L. Hayes, Introduction to Remote Sensing, second ed. Boca Raton, FL, USA: CRC Press, 2007.
[6] Y. Zhang, D. C. Butcher, and E. S. Staab, "Hearty hyper spectral visionbased characterization for multi-season weed mapping," ISPRS J. Photogramm. Remote Sens., vol. 69, pp. 65– 73, 2012.
[7] H. Liu, S.- H. Lee, and J. S. Chahl, "Advancement of a proximal machine vision framework for off-season weed mapping in broadacre no-culturing fallows," J. Comput. Sci., vol. 9, no. 12, pp. 1803– 1821, 2013.
[8] P. Geladi, E. Bengtsson, K. Esbensen, and H. Grahn, "Picture examination in science I. Properties of pictures, greylevel activities, the multivariate picture," Trends Anal. Chem., vol. 11, no. 1, pp. 41– 53, 1992.
[9] G. Lu and B. Fei, "Therapeutic hyper spectral imaging: An audit," J. Biomed. Select., vol. 19, no. 1, 2014, Art. no. 010 901.
[10] C. Duchesne, J. Liu, and J. MacGregor, "Multivariate picture examination in the process ventures: An audit," Chemometrics Intell. Lab. Syst., vol. 117, pp. 116– 128, 2012.
[11] E. Barrett and L. Curtis, Introduction to Environmental Remote Sensing. Dordrecht, The Netherlands: Springer, 1992.
[12] J. Qin, "Section 5: Hyperspectral imaging instruments," in Hyperspectral Imaging for Food Quality Analysis and Control, D.- W. Sun, Ed. San Diego, CA, USA: Academic, 2010, pp. 129– 172.
[13] I. Herrmann, U. Shapira, S. Kinast, A. Karnieli, and D. J. Bonfil, "Groundlevel hyper spectral symbolism for recognizing weeds in wheat fields," Precis. Agribusiness, vol. 14, no. 6, pp. 637– 659, 2013.
[14] A. AlSuwaidi, "Dubaisat-2 mission outline," SPIE Remote Sens., vol. 8533, 2012, Art. no. 85330W.
[15] A. AlRais, A. AlSuwaidi, and H. Ghedira, "Dubaisat-1: Mission outline, advancement status and future applications," in Proc. IEEE Int. Geosci. Remote Sens. Symp., vol. 5, Jul. 2009, pp. V-196– V-199.
[16] A. AlSuwaidi, C. Veys, M. Hussey, B. Lament, and H. Yin, "Hyperspectral choice based calculation for plant characterization," in Proc. IEEE Int. Conf. Imag. Syst. Techn., Oct. 2016, pp. 395– 400.
[17] A. AlSuwaidi, C. Veys, M. Hussey, B. Lament, and H. Yin, "Hyperspectral highlight choice outfit for plant arrangement," in Proc. Hyperspectr. Imag. Appl., Session 4-12.00, Oct. 2016.
[18] A. M. Martinez and A. C. Kak, "PCA versus LDA," IEEE Trans. Example Butt-centric. Mach. Intell., vol. 23, no. 2, pp. 228– 233, Feb. 2001.
[19] R. O. Duda, P. E. Hart, and D. G. Stork, Pattern Classification, second ed. New York, NY, USA: Wiley-Interscience, 2000.
[20] L. Jiang, B. Zhu, and Y. Tao, "Section 3: Hyperspectral picture arrangement techniques," in Hyperspectral Imaging for Food Quality Analysis also, Control, D.- W. Sun, Ed. San Diego, CA, USA: Academic, 2010, pp. 79– 98.
[21] H. Liu and H. Motoda, Feature Selection for Knowledge Discovery and Information Mining. Norwell, MA, USA: Kluwer, 1998.
[22] L. C. Molina, L. Belanche, and A. Nebot, "Highlight choice calculations: An overview and exploratory assessment," in Proc. IEEE Int. Conf. Information Mining, 2002, pp. 306– 313.
[23] K. Kira and L. A. Rendell, "The element choice issue: Traditional strategies and another calculation," in Proc. tenth Nat. Conf. Artif. Intell., 1992, pp. 129– 134.
[24] M. Robnik-Sikonja and I. Kononenko, "Hypothetical and experimental investigation ˇ of Relieff and RRelieff," Mach. Learn., vol. 53, no. 1, pp. 23– 69, 2003.
[25] M. A. Lobby and L. A. Smith, "Highlight determination for machine learning: Contrasting a relationship based channel approach with the wrapper," in Proc. twelfth Int. Florida Artif. Intell. Res. Soc. Conf., 1999, pp. 235– 239.
[26] I. H. Witten, E. Forthcoming, and M. A. Corridor, "Section 8: Ensemble learning," in Data Mining: Practical Machine Learning Tools and Techniques (The Morgan Kaufmann Series in Data Management Systems), I. H. Witten, E. Forthcoming, and M. A. Corridor, Eds., third ed. Boston, MA, USA: Morgan Kaufmann, 2011, pp. 351– 373.
[27] T. G. Dietterich, "Machine-learning research: Four ebb and flow headings," Artif. Intell. Mag., vol. 18, pp. 97– 136, 1997.
[28] R. Polikar, "Group based frameworks in basic leadership," IEEE Circuits Syst. Mag., vol. 6, no. 3, pp. 21– 45, Third Quart. 2006.
[29] L. O. Jimenez, A. Spirits Morell, and A. Creus, "Arrangement of hyperdimensional information dependent on highlight and choice combination approaches utilizing projection interest, lion`s share casting a ballot, and neural systems," IEEE Trans. Geosci. Remote Sens., vol. 37, no. 3, pp. 1360– 1366, May 1999.
[30] U. G. Mangai, S. Samanta, S. Das, and P. R. Chowdhury, "An overview of choice combination and highlight combination techniques for example arrangement," IETE Tech. Rev., vol. 27, no. 4, pp. 293– 307, 2010.
[31] Y. Zhong, Q. Cao, J. Zhao, A. Mama, B. Zhao, and L. Zhang, "Ideal choice combination for urban land-use/arrive cover grouping dependent on versatile differential development utilizing hyper spectral and LiDAR information," Remote Sens., vol. 9, no. 8, 2017, Art. no. 868.
[32] P. Ghamisi, J. Square, Y. Chen, J. Li, and A. J. Square, "Progressed otherworldly classifiers for hyper spectral pictures: An audit," IEEE Geosci. Remote Sens. Mag., vol. 5, no. 1, pp. 8– 32, Mar. 2017.
[33] F. Melgani and L. Bruzzone, "Characterization of hyper spectral remote detecting pictures with help vector machines," IEEE Trans. Geosci. Remote Sens., vol. 42, no. 8, pp. 1778– 1790, Aug. 2004.
[34] P. Ghamisi, A. R. Ali, M. S. Couceiro, and J. A. Benediktsson, "An epic developmental swarm fluffy bunching approach for hyper spectral symbolism," IEEE J. Select. Subjects Appl. Earth Observ. Remote Sens., vol. 8, no. 6, pp. 2447– 2456, Jun. 2015.
[35] B. M. Shahshahani and D. A. Landgrebe, "The impact of unlabeled examples in decreasing the little example measure issue and alleviating the Hughes marvel," IEEE Trans. Geosci. Remote Sens., vol. 32, no. 5, pp. 1087– 1095, Sep. 1994.
[36] C. Persello and L. Bruzzone, "Dynamic and semisupervised learning for the characterization of remote detecting pictures," IEEE Trans. Geosci. Remote Sens., vol. 52, no. 11, pp. 6937– 6956, Nov. 2014.
[37] L. Hamel, "Curiosity location," in Knowledge Discovery With Support Vector Machines. New York, NY, USA: Wiley, 2009, pp. 209– 217.
[38] M. A. Pimentel, D. A. Clifton, L. Clifton, and L. Tarassenko, "An audit of curiosity discovery," Signal Process., vol. 99, pp. 215– 249, 2014.
[39] L. Clifton, D. A. Clifton, Y. Zhang, P. Watkinson, L. Tarassenko, and H. Yin, "Probabilistic curiosity discovery with help vector machines," IEEE Trans. Reliab., vol. 63, no. 2, pp. 455– 467, Jun. 2014.
[40] F. de Morsier, D. Tuia, M. Borgeaud, V. Gass, and J. P. Thiran, "Semisupervised oddity identification utilizing SVM whole arrangement way," IEEE Trans. Geosci. Remote Sens., vol. 51, no. 4, pp. 1939– 1950, Apr. 2013.
[41] D. A. Clifton, L. A. Clifton, P. R. Railing, and L. Tarassenko, "Computerized curiosity discovery in modern frameworks," in Advances of Computational Intelligence in Industrial Systems, Y. Liu, A. Sun, H. T. Loh, W. F. Lu, and E.- P. Lim, Eds. Berlin, Germany: Springer, 2008, pp. 269– 296.
[42] W. Fisher, "Advancement in remote detecting (1972– 1976)," Photogrammetria, vol. 32, pp. 33– 72, 1976.
[43] F. A. Kruse, J. W. Boardman, and J. F. Huntington, "Examination of airborne hyper spectral information and EO-1 Hyperion for mineral mapping," IEEE Trans. Geosci. Remote Sens., vol. 41, no. 6, pp. 1388– 1400, Jun. 2003.
[44] H. Breit, T. Fritz, U. Balss, M. Lachaise, A. Niedermeier, and M. Vonavka, "TerraSAR-X SAR preparing and items," IEEE Trans. Geosci. Remote Sens., vol. 48, no. 2, pp. 727– 740, Feb. 2010.
[45] A. AlSuwaidi, A. AlRais, and H. Ghedira, "Building up a satellite-based device to screen dust a dust storms in the UAE," in Proc. IEEE Int. Geosci. Remote Sens. Symp., Jul. 2010, pp. 1434– 1437.
[46] A. Merentitis, C. Debes, and R. Heremans, "Group learning in hyper spectral picture arrangement: Toward choosing a great predisposition change tradeoff," IEEE J. Select. Points Appl. Earth Observ. Remote Sens., vol. 7, no. 4, pp. 1089– 1102, Apr. 2014.
[47] J. Xia, J. Chanussot, P. Du, and X. He, "Turn based help vector machine group in arrangement of hyper spectral information with restricted preparing tests," IEEE Trans. Geosci. Remote Sens., vol. 54, no. 3, pp. 1519– 1531, Mar. 2016.
[48] K. H. Holland, D. W. Sheep, and J. S. Schepers, "Radiometry of proximal dynamic optical sensors (AOS) for farming detecting," IEEE J. Select. Points Appl. Earth Observ. Remote Sens., vol. 5, no. 6, pp. 1793– 1802, Dec. 2012.
[49] D. A. Sims and J. A. Gamon, "Connections between leaf shade content also, unearthly reflectance over a wide scope of animal varieties, leaf structures and formative stages," Remote Sens. Environ., vol. 81, no. 23, pp. 337– 354, 2002.
[50] G. Rondeaux, M. Steven, and F. Baret, "Improvement of soil-balanced vegetation lists," Remote Sens. Environ., vol. 55, no. 2, pp. 95– 107, 1996.
[51] A. Gitelson and M. N. Merzlyak, "Phantom reflectance changes related with harvest time senescence of Aesculus hippocastanum L. what`s more, Acer platanoides L. leaves. ghostly highlights and connection to chlorophyll estimation," J. Plant Physiol., vol. 143, no. 3, pp. 286– 292, 1994.
[52] T. P. Dawson and P. J. Curran, "another method for adding the reflectance red edge position," Int. J. Remote Sens., vol. 19, no. 11, pp. 2133– 2139, 1998.
[53] A.- K. Mahlein, T. Rumpf, P. Welke, H.- W. Dehne, L. Plmer, U. Steiner, what`s more, E.- C. Oerke, "Advancement of ghostly files for distinguishing and recognizing plant ailments," Remote Sens. Environ., vol. 128, pp. 21– 30, 2013.
[54] J. Penuelas, J. Pinol, R. Ogaya, and I. Filella, "Estimation of plant water fixation by the reflectance water list WI (R900/R970)," Int. J. Remote Sens., vol. 18, no. 13, pp. 2869– 2875, 1997.
[55] B.- C. Gao, "NDWI—A standardized distinction water list for remote detecting of vegetation fluid water from space," Remote Sens. Environ., vol. 58, no. 3, pp. 257– 266, 1996.
[56] T. Rumpf, A.- K. Mahlein, U. Steiner, E.- C. Oerke, H.- W. Dehne, and L. Plmer, "Early discovery and order of plant ailments with help vector machines dependent on hyper spectral reflectance," Comput. Electron. Farming, vol. 74, no. 1, pp. 91– 99, 2010.
[57] A. Apan, A. Held, S. Phinn, and J. Markley, "Recognizing sugarcane orange rust malady utilizing EO-1 Hyperion hyper spectral symbolism," Int. J. Remote Sens., vol. 25, no. 2, pp. 489– 498, 2004.
[58] S. L. Ustin, D. A. Roberts, M. Gardner, and P. Dennison, "Assessment of the capability of Hyperion information to appraise out of control fire risk in the Santa Ynez front range, Santa Barbara, California," in Proc. IEEE Int. Geosci. Remote Sens. Symp., vol. 2, 2002, pp. 796– 798.
[59] I. Guyon and A. Elisseeff, "A prologue to variable and highlight determination," J. Mach. Learn. Res., vol. 3, pp. 1157– 1182, Mar. 2003.
[60] H. Liu and L. Yu, "Toward incorporating highlight choice calculations for characterization and bunching," IEEE Trans. Knowl. Information Eng., vol. 17, no. 4, pp. 491– 502, Apr. 2005.
[61] A. M. De Silva and P. H. W. Leong, "Highlight determination," in GrammarBased Feature Generation for Time-Series Prediction. Singapore: Springer, 2015, pp. 13– 24.
[62] G. Dark colored, A. Pocock, M.- J. Zhao, and M. Lujn, "Restrictive probability boost: A binding together structure for data theoretic element choice," J. Mach. Learn. Res., vol. 13, pp. 27– 66, 2012.
[63] J. Reiche, C. M. Souza, D. H. Hoekman, J. Verbesselt, H. Persaud, and M. Herold, "Highlight level combination of multi-fleeting ALOS PALSAR and Landsat information for mapping and observing of tropical deforestation and backwoods debasement," IEEE J. Select. Themes Appl. Earth Observ. Remote Sens., vol. 6, no. 5, pp. 2159– 2173, Oct. 2013.
[64] W. Liao, A. Piurica, R. Bellens, S. Gautama, and W. Philips, "Summed up diagram based combination of hyper spectral and LiDAR information utilizing morphological highlights," IEEE Geosci. Remote Sens. Lett., vol. 12, no. 3, pp. 552– 556, Blemish. 2015.
[65] X. Bai, C. Liu, P. Ren, J. Zhou, H. Zhao, and Y. Su, "Article arrangement by means of highlight combination based underestimated parts," IEEE Geosci. Remote Sens. Lett., vol. 12, no. 1, pp. 8– 12, Jan. 2015.
[66] G. Dark colored, J. Wyatt, R. Harris, and X. Yao, "Decent variety creation techniques: An overview and categorisation," J. Inf. Combination, vol. 6, pp. 5– 20, 2005.
[67] S. Prasad and L. M. Bruce, "Choice combination with certainty based weight task for hyper spectral target acknowledgment," IEEE Trans. Geosci. Remote Sens., vol. 46, no. 5, pp. 1448– 1456, May 2008.
[68] A. C. Turlapaty, H. K. Goru, and B. Gokaraju, "An element subset based choice combination approach for scene grouping utilizing shading, otherworldly, and surface insights," in Proc. IEEE seventh Int. Adv. Comput. Conf., Jan. 2017, pp. 624– 628.
[69] D. M. Duty and R. P. Duin, "Bolster vector information portrayal," Mach. Learn., vol. 54, no. 1, pp. 45– 66, 2004.
[70] V. Chandola, A. Banerjee, and V. Kumar, "Oddity identification: A review," ACM Comput. Surv., vol. 41, no. 3, pp. 15:1– 15:58, Jul. 2009.
[71] C. M. Cleric, Pattern Recognition and Machine Learning (Information Science and Statistics). Secaucus, NJ, USA: Springer, 2006.
[72] V. Hautamaki, I. Karkkainen, and P. Franti, "Anomaly identification utilizing Knearest neighbor diagram," in Proc. seventh Int. Conf. Example Recog., vol. 3, Aug. 2004, pp. 430– 433. [73] B. Scholkopf, R. Williamson, A. Smola, J. Shawe-Taylor, and J. Platt, ¨"Bolster vector technique for oddity identification," in Proc. twelfth Int. Conf. Neural Inf. Process. Syst., 1999, pp. 582– 588.
[74] B. Scholkopf, J. C. Platt, J. C. Shawe-Taylor, A. J. Smola, and R. C. ¨ Williamson, "Evaluating the help of a high-dimensional dispersion," Neural Comput., vol. 13, no. 7, pp. 1443– 1471, Jul. 2001.
[75] T. M. Cover and J. A. Thomas, Elements of Information Theory (Wiley Arrangement in Telecommunications and Signal Processing). Hoboken, NJ, USA: Wiley-Interscience, 2006.
[76] S. Kulkarni and G. Harman, An Elementary Introduction to Statistical Learning Theory, first ed. New York, NY, USA: Wiley, 2011.
[77] G. Camps-Valls and L. Bruzzone, "Bit based techniques for hyper spectral picture characterization," IEEE Trans. Geosci. Remote Sens., vol. 43, no. 6, pp. 1351– 1362, Jun. 2005.
[78] A.- K. Mahlein, U. Steiner, H.- W. Dehne, and E.- C. Oerke, "Ghostly marks of sugar beet leaves for the location and separation of illnesses," Summary. Horticulture, vol. 11, no. 4, pp. 413– 431, 2010.
[79] D. H. Cultivate, K. Amano, and S. M. C. Nascimento, "Shading steadiness in regular scenes clarified by worldwide picture insights," Vis. Neurosci., vol. 23, nos. 3– 4, pp. 341– 349, 2006.
[80] D. H. Cultivate, K. Amano, S. M. C. Nascimento, and M. J. Cultivate, "Recurrence of metamerism in common scenes," J. Pick. Soc. Amer. A, vol. 23, no. 10, pp. 2359– 2372, Oct. 2006.
[81] A.- K. Mahlein, U. Steiner, C. Hillnhutter, H.- W. Dehne, and E.- C. Oerke, ¨ "Hyperspectral imaging for little scale examination of indications caused by various sugar beet illnesses," Plant Methods, vol. 8, no. 1, 2012, Workmanship. no. 3.
[82] A.- K. Mahlein, S. Hammersley, E.- C. Oerke, H.- W. Dehne, H. Goldbach, furthermore, B. Lament, "Supplemental blue LED lighting exhibit to enhance the flag quality in hyper spectral imaging of plants," Sensors, vol. 15, no. 6, pp. 12834– 12840, 2015.
[83] G. A. Carter, "Proportions of leaf reflectances in thin wavebands as pointers of plant pressure," Int. J. Remote Sens., vol. 15, no. 3, pp. 697– 703, 1994.
[84] C. Daughtry, C. Walthall, M. Kim, E. de Colstoun, and J. McMurtrey III, "Assessing corn leaf chlorophyll fixation from leaf and shade reflectance," Remote Sens. Environ., vol. 74, no. 2, pp. 229– 239, 2000.
[85] D. Haboudane, J. R. Mill operator, N. Tremblay, P. J. Zarco-Tejada, and L. Dextraze, "Coordinated tight band vegetation lists for forecast of edit chlorophyll content for application to accuracy agribusiness," Remote Sens. Environ., vol. 81, no. 23, pp. 416– 426, 2002.
[86] J. Gamon, J. Peuelas, and C. Field, "A limited waveband phantom record that tracks diurnal changes in photosynthetic effectiveness," Remote Sens. Environ., vol. 41, no. 1, pp. 35– 44, 1992.
[87] G.- Z. Li and J. Y. Yang, "Highlight determination for group learning and its application," in Machine Learning in Bioinformatics. New York, NY, USA: Wiley, 2008, pp. 135– 155.