Pre-processing and Classification of Prostate Images for Cancer Detection: A Survey
Swetha.P.C 1 , Mohan G Kabadi2 , Srivinay 3
Section:Survey Paper, Product Type: Journal Paper
Volume-07 ,
Issue-16 , Page no. 93-97, May-2019
CrossRef-DOI: https://doi.org/10.26438/ijcse/v7si16.9397
Online published on May 18, 2019
Copyright © Swetha.P.C, Mohan G Kabadi, Srivinay . This is an open access article distributed under the Creative Commons Attribution License, which permits unrestricted use, distribution, and reproduction in any medium, provided the original work is properly cited.
View this paper at Google Scholar | DPI Digital Library
How to Cite this Paper
- IEEE Citation
- MLA Citation
- APA Citation
- BibTex Citation
- RIS Citation
IEEE Style Citation: Swetha.P.C, Mohan G Kabadi, Srivinay, “Pre-processing and Classification of Prostate Images for Cancer Detection: A Survey,” International Journal of Computer Sciences and Engineering, Vol.07, Issue.16, pp.93-97, 2019.
MLA Style Citation: Swetha.P.C, Mohan G Kabadi, Srivinay "Pre-processing and Classification of Prostate Images for Cancer Detection: A Survey." International Journal of Computer Sciences and Engineering 07.16 (2019): 93-97.
APA Style Citation: Swetha.P.C, Mohan G Kabadi, Srivinay, (2019). Pre-processing and Classification of Prostate Images for Cancer Detection: A Survey. International Journal of Computer Sciences and Engineering, 07(16), 93-97.
BibTex Style Citation:
@article{Kabadi_2019,
author = {Swetha.P.C, Mohan G Kabadi, Srivinay},
title = {Pre-processing and Classification of Prostate Images for Cancer Detection: A Survey},
journal = {International Journal of Computer Sciences and Engineering},
issue_date = {5 2019},
volume = {07},
Issue = {16},
month = {5},
year = {2019},
issn = {2347-2693},
pages = {93-97},
url = {https://www.ijcseonline.org/full_spl_paper_view.php?paper_id=1285},
doi = {https://doi.org/10.26438/ijcse/v7i16.9397}
publisher = {IJCSE, Indore, INDIA},
}
RIS Style Citation:
TY - JOUR
DO = {https://doi.org/10.26438/ijcse/v7i16.9397}
UR - https://www.ijcseonline.org/full_spl_paper_view.php?paper_id=1285
TI - Pre-processing and Classification of Prostate Images for Cancer Detection: A Survey
T2 - International Journal of Computer Sciences and Engineering
AU - Swetha.P.C, Mohan G Kabadi, Srivinay
PY - 2019
DA - 2019/05/18
PB - IJCSE, Indore, INDIA
SP - 93-97
IS - 16
VL - 07
SN - 2347-2693
ER -
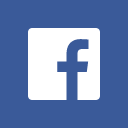
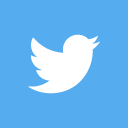
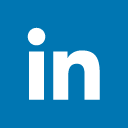
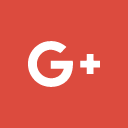
Abstract
In the recent years, prostate cancer has become the major cause of deaths in the male population around the world. Numerous computer aided techniques such as, Computer Aided Diagnosis (CAD) systems have been designed in order to detect prostate cancer. The CAD systems majorly consist of four stages namely preprocessing, segmentation, feature extraction and finally the classification stages that are interdependent on one another. The CAD systems perform the analysis based on the various screening techniques such as X-Ray, CT scans, TRUS images, MRI scan, and mp-MRI scans. Though the existing CAD systems are considered feasible, the major research challenge is in improving the accuracy, specificity, speed and usability of the existing CAD systems. This paper presents a survey on the various methodologies used for detecting the prostate carcinoma using various types of screening images.
Key-Words / Index Term
MRI(Magnetic Resonance Imaging), TRUS (Trans rectal Ultrasound), mp-MRI (Multi parametric-Magnetic ResonanceImaging).
References
[1] T. F. Cootes, A. Hill, C. J. Taylor, J. Haslam, The Use of Active Shape Model for Locating Structures in Medical Images, Image and Vision Computing 12 (1994) 355–66.
[2] R. G. Aarnink, S. D. Pathak, J. J. M. C. H. de la Rosette, F. M. J. Debruyne, Y. Kim, H. Wijkstra, Edge Detection in Prostatic Ultrasound Images Using Integrated Edge Map, Ultrasonics (1998) 635–42
[3] X. Tang, Y. Jeong, R. J. Radke, G. T. Y. Chen, Geometric Model Based Segmentation of the Prostate and Surrounding Structures for Image Guided Radiotherapy, in: Proceedings of the SPIE Medical Imaging : Visual Communications and Image Processing, SPIE, USA, 2004, pp. 168–76.
[4] Mark A. Sheppard and Liwen Shih, “Efficient Image Texture Analysis and Classification for Prostate Ultrasound Diagnosis”, Proceedings of the 2005 IEEE Computational Systems Bioinformatics Conference Workshops (CSBW’05).
[5] Y. Zhu, S. Williams, R. Zwiggelaar, Computer Technology in Detection and Staging of Prostate Carcinoma: A Review, Medical Image Analysis 10 (2006) 178–99.
[6] M. Samiee, G. Thomas, R. Fazel-Rezai, Semi-Automatic Prostate Segmentation of MR Images Based on Flow Orientation, in: IEEE International Symposium on Signal Processing and Information Technology, IEEE Computer Society Press, USA, 2006, pp. 203–7.
[7] J. Li, S.S. Mohamed, M.M.A. Salama, and G.H. Freeman, “Prostate Tissue Texture Feature Extraction for Cancer Recognition in TRUS Images Using Wavelet Decomposition”, M. Kamel and A. Campilho (Eds.): ICIAR 2007, LNCS 4633, pp. 993–1004, 2007. © Springer-Verlag Berlin Heidelberg 2007.
[8] X. Liu, D. L. Langer, M. A. Haider, Y. Yang, M. N. Wernick, I. S. Yetik, Prostate Cancer Segmentation with Simultaneous Estimation of Markov Random Field Parameters and Class, IEEE Transactions on Medical Imaging 28 (2009) 906–15.
[9]R.Manavalan and K.Thangave, “Evaluation ofTextural Feature Extraction from GRLM for Prostate Cancer TRUS Medical Images”, International Journal of Advances in Engineering & Technology, Jan 2012. ©IJAET.
[10] Clara Mosquera-Lopez, SosAgaian, Alejandro Velez-Hoyos, and Ian Thompson, “Computer-aided Prostate Cancer Diagnosis from Digitized Histopathology: A Review on Texture-based Systems”, IEEE Reviews in Biomedical Engineering, ©2013 IEEE.
[11] Aaron Greenblatt, Clara Mosquera-Lopez, SosAgaian, “Quaternion Neural Networks Applied to Prostate Cancer Gleason Grading”, 2013 IEEE International Conference on Systems, Man, and Cybernetics, ©2013 IEEE.
[12] Eman Magdy, Nourhan Zayed, and Mahmoud Fakhr, “Automatic Classification of Normal and Cancer Lung CT Images Using Multiscale AM-FM Features”, International Journal of Biomedical Imaging, Volume 2015, September 2015
[13] Duc Fehra, Harini Veeraraghavana, Andreas Wibmerb , Tatsuo Gondoc , Kazuhiro Matsumotoc ,Herbert Alberto Vargasb, EvisSalab, HedvigHricakb , and Joseph O. Deasya, “Automatic classification of prostate cancer Gleason scores from multiparametric magnetic resonance images”, PNAS Published online November 2, 2015.
[14] Chuan-Yu Chang, Hui-Ya Hu, Yuh-Shyan Tsai, “Prostate Cancer Detection in Dynamic MRIs”, ©2015 IEEE.
[15] Islam Reda, Ahmed Shalaby, Fahmi Khalifa, Mohammed Elmogy, Ahmed Aboulfotouh, Mohamed Abou El-Ghar, Ehsan Hosseini-Asl, Naoufel Werghi, Robert Keynton, and Ayman El-Baz, “COMPUTER-AIDED DIAGNOSTIC TOOL FOR EARLY DETECTION OF PROSTATE CANCER”, 978-1-4673-9961-6/16 ©2016 IEEE.
[16] Yunjie Chen, Liangbin Zhang, Wenjun Ma, Su Zhang, Fen Gao, Yunkai Zhu, Yaqing Chen, “Cancer Lesion Screening in MRI for Prostate Biopsy”, ©2016 IEEE.
[17] Pooja R. Katre; AnuradhaThakare, “ Detection of lung cancer stages using image processing and data classification techniques”, IEEE 2nd International Conference for Convergence in Technology (I2CT), pp 402-404, ISBN: 978-1-5090-4307-1, April 2017
[18] LilikAnifah; Haryanto; RinaHarimurti; ZaimahPermatasari; PuputWanartiRusimamto; Adam RidianthoMuhamad, “Cancer lungs detection on CT scan image using artificial neural network backpropagation based gray level coocurrence matrices feature”, IEEE International Conference on Advanced Computer Science and Information Systems (ICACSIS), pp 327-332, ISBN: 978-1- 5386 - 3172 – 0, October 2017, Jakarta, Indonesia
[19] Ying Liu and Xiaomei An, “A Classification Model for the Prostate Cancer Based on Deep Learning”, 2017 10th International Congress on Image and Signal Processing, BioMedical Engineering and Informatics (CISP-BMEI 2017).
[20] Salsabil Amin El-Regaily; Mohammed Abdel Megeed Salem; Mohamed Hassan Abdel Aziz; Mohamed Ismail Roushdy, “ Lung nodule segmentation and detection in computed tomography”, IEEE Eighth International Conference on Intelligent Computing and Information Systems (ICICIS),pp 72-78, ISBN 977-237-172-3,December 2017, Egypt.
[21] Islam Reda, Ahmed Shalaby, Mohammed Elmogy, Mohammed Ghazal, Ahmed Aboulfotouh, Mohamed Abou El-Ghar, Adel Elmaghraby, Robert Keynton, and Ayman El-Baz, “A New Fast Framework for Early Detection of Prostate Cancer Without Prostate Segmentation”, 978-1-5386-6628-9/18 ©2018 IEEE.
[22] Xiang-Xia Li; Bin Li; Lian-Fang Tian; Li Zhang, “ Automatic benign and malignant classification of pulmonary nodules in thoracic computed tomography based on RF algorithm”, IET Image processing Journal , volume 12, issue 7, pp 1253-1264, 2018, Print ISSN: 1751-9659
[23] R.R. Wildeboer , A.W. Postema , L. Demi , M.P.J. Kuenen , H. Wijkstra and M. Mischi “Multiparametric Dynamic Contrast-Enhanced Ultrasound Classification of Prostate Cancer “, ©2016 IEEE.
[24] Islam Reda, Ahmed Shalaby , Mohamed Abou El-Ghar, Fahmi Khalifa, Mohammed Elmogy, Ahmed Aboulfotouh1 , Ehsan Hosseini-Asl, Ayman El-Baz, and Robert Keynton, “A NEW NMF-AUTOENCODER BASED CAD SYSTEM FOR EARLY DIAGNOSIS OF PROSTATE CANCER”, ©2016 IEEE.
[25] Yujie Feng, Fan Yang, Xichuan Zhou Member, IEEE, Yanli Guo, Fang Tang, Fengbo Ren, Jishun Guo, Shuiwang Ji Member, IEEE, “A Deep Learning Approach for Targeted Contrast-Enhanced Ultrasound Based Prostate Cancer Detection”, IEEE 2018.
[26] aapm.onlinelibrary.wiley.com Internet Source.
[27] ijarcet.org Internet Source.
[28] hal.archives-ouvertes.fr Internet Source.
[29] www.africaangel.com Internet Source.
[30] www.pcf.org Internet Source.