Contribution of Machine Learning and Deep Learning for Diagnosis of Retinal Detachment
K. Deviga1 , S. Ramalakshmi2 , T. Ratha Jeyalakshmi3
Section:Research Paper, Product Type: Journal Paper
Volume-07 ,
Issue-16 , Page no. 47-50, May-2019
CrossRef-DOI: https://doi.org/10.26438/ijcse/v7si16.4750
Online published on May 18, 2019
Copyright © K. Deviga, S. Ramalakshmi, T. Ratha Jeyalakshmi . This is an open access article distributed under the Creative Commons Attribution License, which permits unrestricted use, distribution, and reproduction in any medium, provided the original work is properly cited.
View this paper at Google Scholar | DPI Digital Library
How to Cite this Paper
- IEEE Citation
- MLA Citation
- APA Citation
- BibTex Citation
- RIS Citation
IEEE Style Citation: K. Deviga, S. Ramalakshmi, T. Ratha Jeyalakshmi, “Contribution of Machine Learning and Deep Learning for Diagnosis of Retinal Detachment,” International Journal of Computer Sciences and Engineering, Vol.07, Issue.16, pp.47-50, 2019.
MLA Style Citation: K. Deviga, S. Ramalakshmi, T. Ratha Jeyalakshmi "Contribution of Machine Learning and Deep Learning for Diagnosis of Retinal Detachment." International Journal of Computer Sciences and Engineering 07.16 (2019): 47-50.
APA Style Citation: K. Deviga, S. Ramalakshmi, T. Ratha Jeyalakshmi, (2019). Contribution of Machine Learning and Deep Learning for Diagnosis of Retinal Detachment. International Journal of Computer Sciences and Engineering, 07(16), 47-50.
BibTex Style Citation:
@article{Deviga_2019,
author = {K. Deviga, S. Ramalakshmi, T. Ratha Jeyalakshmi},
title = {Contribution of Machine Learning and Deep Learning for Diagnosis of Retinal Detachment},
journal = {International Journal of Computer Sciences and Engineering},
issue_date = {5 2019},
volume = {07},
Issue = {16},
month = {5},
year = {2019},
issn = {2347-2693},
pages = {47-50},
url = {https://www.ijcseonline.org/full_spl_paper_view.php?paper_id=1276},
doi = {https://doi.org/10.26438/ijcse/v7i16.4750}
publisher = {IJCSE, Indore, INDIA},
}
RIS Style Citation:
TY - JOUR
DO = {https://doi.org/10.26438/ijcse/v7i16.4750}
UR - https://www.ijcseonline.org/full_spl_paper_view.php?paper_id=1276
TI - Contribution of Machine Learning and Deep Learning for Diagnosis of Retinal Detachment
T2 - International Journal of Computer Sciences and Engineering
AU - K. Deviga, S. Ramalakshmi, T. Ratha Jeyalakshmi
PY - 2019
DA - 2019/05/18
PB - IJCSE, Indore, INDIA
SP - 47-50
IS - 16
VL - 07
SN - 2347-2693
ER -
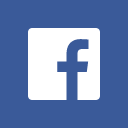
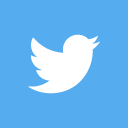
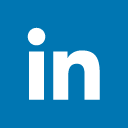
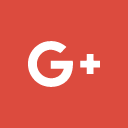
Abstract
In medical specialty the human eye is a very important diagnostic issue. The problem of retinal detachment is commonly for the people over the age of 50 and it affects the people who had previous eye surgery like cataract removal and also severe eye injuries. The Segmentation in fundus imaging that is a non-trivial task because of the variable size of vessels, comparatively low distinction, and potential presence of pathologies like micro-aneurysm. Many machine learning, deep learning algorithms, have been proposed for this purpose. This paper provides recently invented ideas to improve the technique for blood vessel segmentation to enhance retinal fundus photographic images. Many variants of segmentation methods are considered, including Tyler L. Coye where is an improved version of segmentation methodology used to segment the blood vessels for fundus photography image. The proposed approach was tested and evaluated on Agarwal Eye Hospital’s fundus dataset which consists of 100 photographic images.
Key-Words / Index Term
Pre-processing, Image Enhancement, Tyler Coye Segmentation Algorithm, Image extraction Retinal Detachment (RD)
References
[1] Gonzalez, R.C., Woods, R.E: “Digital Imaging Processing”, Prentice Hall: New York, NY, USA, 2002.
[2] Gangwar, S., Chauhan , R.P.: “Survey of Clustering Techniques Enhancing Image Segmentation Process”. International conference on Advances in Computing and Communication Engineering,2015, pp. 34-39Naz, S., Majeed h., Irshad, H: “Image Segmentation fuzzy clustering: A Survey”, International Conference on emerging technologies,2010,pp 181-186.
[3] Karoui I., Boucher J., Augustin J.: “ Variational Region-based Segmentation using Multiple Texture Statistics”, IEEE trans on Image processing,2010,19, (12), pp. 3146-3156.
[4] Solomon, C.J., Breckon, T.P: “Fundamentals of Digital Image Processing: A Practical Approach with Examples in Matlab” Wiley Blackwell 1st edn.2010.
[5] Wang, Q., Chang L., Sun Z., Zhou M., Li Q., Liu, H., Guo F.: “An Improved Support Vector Machine Algorithm for Blood Cell Segmentation from Hyperspectral images”, Institute of Electrical and electronics Engineers,2016, pp. 35-39.
[6] Tan, K.S., Isa, N.A.N.: “Color image segmentation using Histogram thresholding-Fuzzy C-means hybrid approach” Pattern Recognition, 2011, 44, (1), pp. 1-15.
[7] Sahoo, P.K., Soltani S., Wong., A.K.C.: “A Survey of thresholding techniques”, computer Vision, Graphics and Image Processing, 1988,41 (2), pp. 233- 260.
[8] R. Rangaya,F. Oloumi, F. Oloumi, P.Eshghzadeh- Zanjani,and F.Ayres Detection of blood vessels in the retina using Gabor filters, “ in Canadian Conference on Electrical and Computer Engineering, 2007,pp.717-720.
[9] M. Miri and A. Mahloojifar, “Retinal Image Analysis using Curvelet Transform and Multistructure Elements Morphology by Reconstruction,” IEEE Transactions on Biomedical Engineering, vol. 58, no.5, pp. 1183-1192,2011.
[10] B. Al-Diri, A. Hunter, and D. Steel, “An active contour model for segmenting and measuring retinal vessels, “IEEE Transactions on Medical Imaging, vol. 28, no.9, pp. 1488-1497, 2009.
[11] M J. Staal, M. Abramoff, M. Niemeijer, M. Viergever, and B. van Ginneken, “ Ridge-based vessel segmentation in color images of the retina,” IEEE Transactions on medical Imaging, vol. 23, no.4, pp. 501-509, 2004.
[12] Ricci and R. Perfetti, “retinal Blood Vessel Segmentation using line operators and Support Vector Classification,” IEEE Transactions on Medical Imaging, vol. 26, no. 10, pp. 1357-1365, 2007.
[13] M. Fraz, P. Remagnino, A. Hoppe, B. Uyyanonvara, A. Rudnicka, C.Owen, and S. Barman,” An ensemble classification based approach applied to retinal blood vessel segmentation,” IEEE Transaction on Biomedical Engineering, vol.59, no.9, pp. 2538-2548,2012.
[14] Roy Chowdhury, “ Automated Segmentation and Pathology Detection in Ophthalmic Images – Dissertation”.
[15] B.Lam, Y.Gao, and A-C.Liew,”General retinal vessel segmentation using regularization-based multiconcavity modeling,” IEEE Transactions on Medical Imaging , vol. 29, no.7, pp. 1369-1381, 2010.
[16] L.Drever, W. Roa, A. Mcewan,andD.Robinson, “Iterative threshold segmentation for PET target volume detection, “ Medical Physics,vol.34, pp.1253- 1265,2007.
[17] Mendonca and A. Campilho, “ Segmentation of retinal blood vessels by combining the detection of centerlines and morphological reconstruction, “IEEE Transactions on Medical Imaging, vol.25, no.9, pp.1200-1213,2006.
[18] D.Marin, A. Aquino, M.Gegundez-Arias,and J.Bravo, “A new supervised method for blood vessel segmentation in retinal images by using gray-level and moment invaiants-based features,” IEEE Transactions on Medical Imaging, vol.30, no.1, pp.146-158, 2011.
[19] M. Niemeijer, J. Staal. B. vanGinneken, M.Loog, and M. D. Abramoff, “comparative study of retinal vessel segmentation methods on a new publicly available database, “ Proc. SPIE 5730, Medical Imaging , vol. 5370, pp. 648-656,2004.
[20] Yuen Aoi Chee, Masturah Mohamed Mokhtar, Rania Hussien Al-Ashwal and EkoSupriyanto, Image Processing analysis for ultrasound retinal detachment images, Advances in Environment, Biotechnology and Biomedicine,355-360.
[21] HideharuOhsugi, Hitoshi Tabuchi, Hiroki Enno and NaofumiIshitobi, Accuracy of deep learning, a machine-learning, using ultra-wide field fundus Ophthalmoscopy for detecting rhegmatogenous retinal detachment, 2017, 01-33
[22] Mrinal Haloi, “Improved Microaneurysm detection using Deep Neural Networks,” 2016 arXiv:1505.04424v2[cs.CV].
[23] H. Suzuki and J. Toriwaki, “automatic segmentation of head MRI images by knowledge guided thresholding, “ Computerized Medical Imaging & Graphics the Official Journal of the Computerized Medical Imaging Society, vol.15, pp. 233-240, 1991.