Salary Prediction in It Job Market
Navyashree M1 , Navyashree M K2 , Neetu M3 , Pooja G R4 , Arun Biradar5
Section:Research Paper, Product Type: Journal Paper
Volume-07 ,
Issue-15 , Page no. 78-84, May-2019
CrossRef-DOI: https://doi.org/10.26438/ijcse/v7si15.7884
Online published on May 16, 2019
Copyright © Navyashree M, Navyashree M K, Neetu M, Pooja G R, Arun Biradar . This is an open access article distributed under the Creative Commons Attribution License, which permits unrestricted use, distribution, and reproduction in any medium, provided the original work is properly cited.
View this paper at Google Scholar | DPI Digital Library
How to Cite this Paper
- IEEE Citation
- MLA Citation
- APA Citation
- BibTex Citation
- RIS Citation
IEEE Style Citation: Navyashree M, Navyashree M K, Neetu M, Pooja G R, Arun Biradar, “Salary Prediction in It Job Market,” International Journal of Computer Sciences and Engineering, Vol.07, Issue.15, pp.78-84, 2019.
MLA Style Citation: Navyashree M, Navyashree M K, Neetu M, Pooja G R, Arun Biradar "Salary Prediction in It Job Market." International Journal of Computer Sciences and Engineering 07.15 (2019): 78-84.
APA Style Citation: Navyashree M, Navyashree M K, Neetu M, Pooja G R, Arun Biradar, (2019). Salary Prediction in It Job Market. International Journal of Computer Sciences and Engineering, 07(15), 78-84.
BibTex Style Citation:
@article{M_2019,
author = {Navyashree M, Navyashree M K, Neetu M, Pooja G R, Arun Biradar},
title = {Salary Prediction in It Job Market},
journal = {International Journal of Computer Sciences and Engineering},
issue_date = {5 2019},
volume = {07},
Issue = {15},
month = {5},
year = {2019},
issn = {2347-2693},
pages = {78-84},
url = {https://www.ijcseonline.org/full_spl_paper_view.php?paper_id=1205},
doi = {https://doi.org/10.26438/ijcse/v7i15.7884}
publisher = {IJCSE, Indore, INDIA},
}
RIS Style Citation:
TY - JOUR
DO = {https://doi.org/10.26438/ijcse/v7i15.7884}
UR - https://www.ijcseonline.org/full_spl_paper_view.php?paper_id=1205
TI - Salary Prediction in It Job Market
T2 - International Journal of Computer Sciences and Engineering
AU - Navyashree M, Navyashree M K, Neetu M, Pooja G R, Arun Biradar
PY - 2019
DA - 2019/05/16
PB - IJCSE, Indore, INDIA
SP - 78-84
IS - 15
VL - 07
SN - 2347-2693
ER -
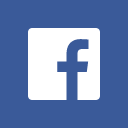
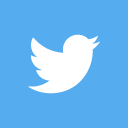
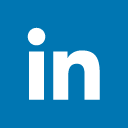
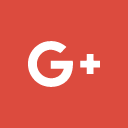
Abstract
In this study, Random Forest Regression machine learning rule is applied to predict salary levels of individuals supported their years of expertise. Random forest regression is employed since it gave higher accuracy compared to decision tree regression and Support Vector Regression classifier. Choosing the foremost effective machine learning rule so as to unravel the problems of classification and prediction of data is the most vital part of machine learning which depends on dataset likewise. The predictive accuracy of the Random forest regression on test data is 97%, while the accuracy of Decision tree regression and Support Vector Regression is 85% and 90% respectively. The model has been used on the training data to predict dependent variables and to extract features with highest impact on salary prediction.
Key-Words / Index Term
Machine learning, Random forest regression, Decision tree regression, Support vector regression, salary prediction
References
[1] A. Parkavi1, K. Lakshmi “Predicting the Course Knowledge Level of Students using Data Mining Techniques “,IEEE International Conference on Smart Technologies and Management for Computing, Communication, Controls, Energy and Materials (ICSTM), (2017).
[2] A. M Shahiri, W. Husain and N.A Rashid, “A Review on Predicting Students‟ Performance using Data mining Techniques,” in The Third Information System Information Council
[3] R.A. Huebner, “A survey of educational data mining research,” Research in Higher Education Journal.
[4] Improving Students‟ Motivation to Study using Salary
Prediction System Pornthep Khongchai, Pokpong Songmuang Department of Computer Science Faculty of Science and Technology
[5] S. Anupama Kumar Vijayalakshmi M.N. “Inference of Naïve Baye‟s Technique on Student Assessment Data”, R.V.
College of Engineering, Bangalore, India
[6] John Jerrim, “Do college students make better predictions of their future income than young adults in the labor force?”, Education Economics, 23:2, p 162-179, 2013.
[7] Karlar Hamlen and William A. Hamlen,” Faculty Salary as a predictor of student outgoing salaries from MBA programs”, Journal of Education for Business
[8] ”Efficient Classification of Data Using Decision Tree” by Bhaskar N. Patel, Satish G. Prajapati, and Dr. Kamaljit I. Lakhtaria.
[9] Kayah, F. “Discretizing Continuous Features for Naive Bayes and C4. Classifiers”. University of Maryland publications: College Park, MD, USA.
[10] S. Taruna, Mrinal Pandey, “An empirical analysis of
classification techniques for predicting academic performance”, IEEE Advances Computing Conference (2004).All references arranged in the following format for and remove website (URL) references or replaced by Journal references.
[11] F. Amato, R. Boselli, M. Cesarini, F. Mercorio, M. Mezzanzanica, V. Moscato, F. Persia, and A. Picariello. Challenge: Processing web texts for classifying job offers. In Semantic Computing (ICSC), 2015 IEEE International Conference on, pages 460– 463, Feb 2015.
[12] Y. Abbound, A. Boyer, and A. Brun. Predict the emergence: Application to competencies in job offers. In Tools with Artificial Intelligence (ICTAI), 2015 IEEE 27th International Conference on, pages 612– 619, Nov 2015.
[13] Hamidah Jantan, Abdul Razak Hamdan, and Zulaiha Ali Othman. Knowledge discovery techniques for talent forecasting in human resource application. World Academy of Science, Engineering and Technology, 50:775–783, 2009.
[14] Chen-Fu Chien and Li-Fei Chen. Data mining to improve personnel selection and enhance human capital: A case study in high-technology industry. Expert Systems with Applications, 34(1):280 – 290, 2008.
[15] Alessandro Bozzon, Marco Brambilla, Stefano Ceri, Matteo Silvestri, and Giuliano Vesci. Choosing the right crowd: expert finding in social networks. In Proceedings of the 16th International Conference on Extending Database Technology, pages 637–648. ACM, 2013.
[16] R Schlogel, I Marchesini, M Alvioli, P Reichenbach, ¨ M Rossi, and J-P Malet. Optimizing landslide susceptibility zonation: Effects of dem spatial resolution and slope unit delineation on logistic regression models. Geomorphology, 2017.
Faruk Bulut and Mehmet Fatih Amasyali. Locally adaptive k parameter selection for nearest neighbor classifier: one nearest cluster. Pattern Analysis and Applications, 20(2):415–425, 2017.
[17] Antonio Alarcon-Paredes, Gustavo Adolfo Alonso, ´ Eduardo Cabrera, and Rene Cuevas-Valencia. Simul- ´ taneous gene selection and weighting in nearest neighbor classifier for gene expression data. In International Conference on Bioinformatics and Biomedical Engineering, pages 372–381. Springer, 2017.
[18] Binh Thai Pham, Dieu Tien Bui, Hamid Reza Pourghasemi, Prakash Indra, and MB Dholakia. Landslide susceptibility assesssment in the uttarakhand area (india) using gis: a comparison study of prediction capability of na¨ıve bayes, multilayer perceptron neural networks, and functional trees methods. Theoretical and Applied Climatology, 128(1-2):255–273, 2017.
[19] Yudong Zhang, Yi Sun, Preetha Phillips, Ge Liu, Xingxing Zhou, and Shuihua Wang. A multilayer perceptron based smart pathological brain detection system by fractional fourier entropy. Journal of medical systems, 40(7):173, 2016.
[20] Jie Xu, Xianglong Liu, Zhouyuan Huo, Cheng Deng, Feiping Nie, and Heng Huang. Multi-class support vector machine via maximizing multi-class margins. In The 26th International Joint Conference on Artificial Intelligence (IJCAI 2017), 2017.
[21] Ying Zhou, Wanjun Su, Lieyun Ding, Hanbin Luo, and Peter ED Love. Predicting safety risks in deep foundation pits in subway infrastructure projects: Support vector machine approach. Journal of Computing in Civil Engineering, 31(5):04017052, 2017.
[22] F Provost, C Hibert, and J-P Malet. Automatic classification of endogenous landslide seismicity using the random forest supervised classifier. Geophysical Research Letters, 44(1):113–120, 2017.
[23] Siddharth Hariharan, Siddhesh Tirodkar, Alok Porwal, Avik Bhattacharya, and Aurore Joly. Random forest-based prospectivity modelling of greenfield terrains using sparse deposit data: An example from the tanami region, western australia. Natural Resources Research, pages 1–19, 2017.
[24] Michael Sprenger, Sebastian Schemm, Roger Oechslin, and Johannes Jenkner. Nowcasting foehn wind events using the adaboost machine learning algorithm. Weather and Forecasting, 32(3):1079–1099, 2017.