Development of Machine Learning-Based Predictive Models for Air Quality Analysis and Prediction
Ashok Shah1 , Prayash Rimal2 , Dev Bhasker Singh3 , Jagadeesh B N4
Section:Research Paper, Product Type: Journal Paper
Volume-07 ,
Issue-15 , Page no. 32-35, May-2019
CrossRef-DOI: https://doi.org/10.26438/ijcse/v7si15.3235
Online published on May 16, 2019
Copyright © Ashok Shah, Prayash Rimal, Dev Bhasker Singh, Jagadeesh B N . This is an open access article distributed under the Creative Commons Attribution License, which permits unrestricted use, distribution, and reproduction in any medium, provided the original work is properly cited.
View this paper at Google Scholar | DPI Digital Library
How to Cite this Paper
- IEEE Citation
- MLA Citation
- APA Citation
- BibTex Citation
- RIS Citation
IEEE Style Citation: Ashok Shah, Prayash Rimal, Dev Bhasker Singh, Jagadeesh B N, “Development of Machine Learning-Based Predictive Models for Air Quality Analysis and Prediction,” International Journal of Computer Sciences and Engineering, Vol.07, Issue.15, pp.32-35, 2019.
MLA Style Citation: Ashok Shah, Prayash Rimal, Dev Bhasker Singh, Jagadeesh B N "Development of Machine Learning-Based Predictive Models for Air Quality Analysis and Prediction." International Journal of Computer Sciences and Engineering 07.15 (2019): 32-35.
APA Style Citation: Ashok Shah, Prayash Rimal, Dev Bhasker Singh, Jagadeesh B N, (2019). Development of Machine Learning-Based Predictive Models for Air Quality Analysis and Prediction. International Journal of Computer Sciences and Engineering, 07(15), 32-35.
BibTex Style Citation:
@article{Shah_2019,
author = {Ashok Shah, Prayash Rimal, Dev Bhasker Singh, Jagadeesh B N},
title = {Development of Machine Learning-Based Predictive Models for Air Quality Analysis and Prediction},
journal = {International Journal of Computer Sciences and Engineering},
issue_date = {5 2019},
volume = {07},
Issue = {15},
month = {5},
year = {2019},
issn = {2347-2693},
pages = {32-35},
url = {https://www.ijcseonline.org/full_spl_paper_view.php?paper_id=1196},
doi = {https://doi.org/10.26438/ijcse/v7i15.3235}
publisher = {IJCSE, Indore, INDIA},
}
RIS Style Citation:
TY - JOUR
DO = {https://doi.org/10.26438/ijcse/v7i15.3235}
UR - https://www.ijcseonline.org/full_spl_paper_view.php?paper_id=1196
TI - Development of Machine Learning-Based Predictive Models for Air Quality Analysis and Prediction
T2 - International Journal of Computer Sciences and Engineering
AU - Ashok Shah, Prayash Rimal, Dev Bhasker Singh, Jagadeesh B N
PY - 2019
DA - 2019/05/16
PB - IJCSE, Indore, INDIA
SP - 32-35
IS - 15
VL - 07
SN - 2347-2693
ER -
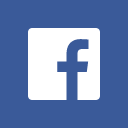
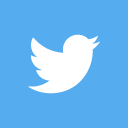
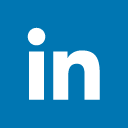
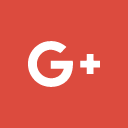
Abstract
One of the biggest environmental problems right now is air pollution. Air quality is needed to be consistently monitored and assessed to ensure better living conditions. The U.S. Environmental Protection Agency (EPA) uses the air quality index (AQI) to standardize the air quality. However, AQI requires precise and accurate sensor readings and complex calculations, making it not feasible for portable air quality monitoring devices. The aim of this paper is to find an alternative way of monitoring and characterizing air quality through the use of integrated gas sensors and building predictive models using machine learning algorithms that can be used to obtain data-driven solutions to mitigate the risk of air pollution.
Key-Words / Index Term
AQI(Air Quality Index),Data Cleaning, Softmax Function
References
[1] R. Tibshirani, “Regression shrinkage and selection via the lasso,”Journal of the Royal Statistical Society. Series B(Methodological),vol. 58, no. 1, pp. 267–288, 1996.
[2] M. Yuan and Y. Lin, “Model selection and estimation in regressionwith grouped variables,” Journal of the Royal Statistical Society:Series B (Statistical Methodology), vol. 68, pp. 49–67, 2006.
[3] L. Li, X. Zhang, J. Holt, J. Tian, and R. Piltner, “Spatiotemporal interpolation methods for air pollution exposure,” in Symposiumon Abstraction, Reformulation, and Approximation, 2011.
[4] Y. Zheng, F. Liu, and H.-P. Hsieh, “U-air: When urban air quality inference meets big data,” in Proceedings of the 19th ACM SIGKDD International Conference on Knowledge Discovery and Data Mining, ser. KDD ’13, 2013, pp. 1436–1444.
[5] World Health Organization (WHO), “7 million premature deaths annually linked to air pollution,” Mar. 2014. [Online]. Available: http://www.who.int/mediacentre/news/releases/2014/airpollution/en
[6] Y.C. Wang and G.W. Chen, “Efficient Data Gathering and Estimationfor Metropolitan Air Quality Monitoring by Using Vehicular Sensor Networks,” IEEE Trans. Veh. Technol., vol. 66, no. 8, pp. 7234–7248,2017.
[7] Y. Li and J. He, “Design of an intelligent indoor air quality monitoringand purification device,” in 2017 IEEE 3rd Information Technology andMechatronics Engineering Conference (ITOEC), 2017, pp. 1147–1150.
[8] G. O. Avendanoet al., “Microcontroller and app-based air quality monitoring system for particulate matter 2.5 (PM2.5) and particulate matter 1 (PM1),” in 2017 IEEE 9th International Conference onHumanoid, Nanotechnology, Information Technology, Communicationand Control, Environment and Management (HNICEM), 2017, vol. 5, pp. 1–4.
[9] J. Molka-Danielsen, P. Engelseth, V. Olesnanikova, P. Sarafin, and R. Zalman, “Big Data Analytics for Air Quality Monitoring at a Logistics Shipping Base via Autonomous Wireless Sensor NetworkTechnologies,” 2017 5th Int. Conf. Enterp. Syst., pp. 38–45, 2017.
[10] Y. Wu et al., “Mobile Microscopy and Machine Learning Provide Accurate and High-throughput Monitoring of Air Quality,” in Conference on Lasers and Electro-Optics, 2017.