Kernel Induced Possiblistic Unsupervised Clustering Techniques in Analyzing Breast Cancer Database
S.R. Kannan1 , M. Siva2 , R. Devi3 , Mark Last4 , Ramathilagam 5
Section:Research Paper, Product Type: Journal Paper
Volume-07 ,
Issue-14 , Page no. 93-98, May-2019
CrossRef-DOI: https://doi.org/10.26438/ijcse/v7si14.9398
Online published on May 15, 2019
Copyright © S.R. Kannan, M. Siva, R. Devi, Mark Last, Ramathilagam . This is an open access article distributed under the Creative Commons Attribution License, which permits unrestricted use, distribution, and reproduction in any medium, provided the original work is properly cited.
View this paper at Google Scholar | DPI Digital Library
How to Cite this Paper
- IEEE Citation
- MLA Citation
- APA Citation
- BibTex Citation
- RIS Citation
IEEE Style Citation: S.R. Kannan, M. Siva, R. Devi, Mark Last, Ramathilagam, “Kernel Induced Possiblistic Unsupervised Clustering Techniques in Analyzing Breast Cancer Database,” International Journal of Computer Sciences and Engineering, Vol.07, Issue.14, pp.93-98, 2019.
MLA Style Citation: S.R. Kannan, M. Siva, R. Devi, Mark Last, Ramathilagam "Kernel Induced Possiblistic Unsupervised Clustering Techniques in Analyzing Breast Cancer Database." International Journal of Computer Sciences and Engineering 07.14 (2019): 93-98.
APA Style Citation: S.R. Kannan, M. Siva, R. Devi, Mark Last, Ramathilagam, (2019). Kernel Induced Possiblistic Unsupervised Clustering Techniques in Analyzing Breast Cancer Database. International Journal of Computer Sciences and Engineering, 07(14), 93-98.
BibTex Style Citation:
@article{Kannan_2019,
author = {S.R. Kannan, M. Siva, R. Devi, Mark Last, Ramathilagam},
title = {Kernel Induced Possiblistic Unsupervised Clustering Techniques in Analyzing Breast Cancer Database},
journal = {International Journal of Computer Sciences and Engineering},
issue_date = {5 2019},
volume = {07},
Issue = {14},
month = {5},
year = {2019},
issn = {2347-2693},
pages = {93-98},
url = {https://www.ijcseonline.org/full_spl_paper_view.php?paper_id=1098},
doi = {https://doi.org/10.26438/ijcse/v7i14.9398}
publisher = {IJCSE, Indore, INDIA},
}
RIS Style Citation:
TY - JOUR
DO = {https://doi.org/10.26438/ijcse/v7i14.9398}
UR - https://www.ijcseonline.org/full_spl_paper_view.php?paper_id=1098
TI - Kernel Induced Possiblistic Unsupervised Clustering Techniques in Analyzing Breast Cancer Database
T2 - International Journal of Computer Sciences and Engineering
AU - S.R. Kannan, M. Siva, R. Devi, Mark Last, Ramathilagam
PY - 2019
DA - 2019/05/15
PB - IJCSE, Indore, INDIA
SP - 93-98
IS - 14
VL - 07
SN - 2347-2693
ER -
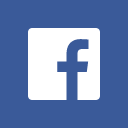
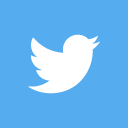
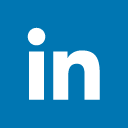
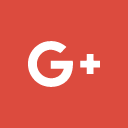
Abstract
The challenge in medical breast cancer database is to differentiate the sub types of cancers in the data. Analyzing the medical breast cancer database is most important one in identifying cancer types which cause deaths. Therefore in order to analyze the types of diseases in cancer databases this paper develops fuzzy set based unsupervised effective clustering technique and implements it with breast cancer database to divide it into available subtypes. This paper introduces the objective function of unsupervised effective proposed clustering technique by incorporating kernel induced distance, kernel functions, and possibilistic memberships. Through the experimental part of this paper the efficiency of proposed method is proved.
Key-Words / Index Term
Clustering, Fuzzy C-Means, Kernel Distance, Breast Cancer Data
References
[1] Akay, Mehmet Fatih, "Support vector machines combined with feature selection for breast cancer diagnosis" , Expert systems with applications, Vol.36, Issue 2, PP.3240-3247, 2009.
[2] Antony, S. Julian Savari, "Detected Breast Cancer on Mammographic Image Classification Using Fuzzy C-Means Algorithm", International Journal of Innovations in Engineering and Technology, Vol.36, Issue 2, 2014.
[3] Basha, S. Saheb, K. Satya Prasad, "Automatic detection of breast cancer mass in mammograms using morphological operators and Fuzzy C-Means clustering”, Journal of Theoretical & Applied Information Technology, Vol.5, Issue.6, 2009
[4] Bezdek J.C, “Pattern Recognition with Fuzzy Objective Function Algorithms”, Plenum Press, New York, 1981.
[5] Bing Liu, Chunru Wan, L.P. Wang, "An efficient semi-unsupervised gene selection method via spectral biclustering", IEEE Transactions on Nano-Bioscience, Vol.5, Issue.2, pp.110-114, 2006.
[6] Carlos Alzate, Johan A.K. Suykens, “Sparse kernel spectral clustering models for large scale dataanalysis”, Neurocomputing, Vol.74, Issue.9, pp.1382-1390, 2011.
[7] Chen, Hui-Ling, "A support vector machine classifier with rough set-based feature selection for breast cancer diagnosis", Expert Systems with Applications, Vol.38, Issue.7, pp. 9014-9022, 2011.
[8] Chih-Hsuan Wang, “Outlier identification and market segmentation using kernel-based clustering techniques”, Expert Systems with Applications Vol.36, Issue.2, pp. 3744-3750, 2009.
[9] Ching-Hao Lai et al., “Oncogenes and Subtypes of Diffuse Large B-Cell Lymphoma Discoveries from Microarray Database”, JCIS, Atlantis Press, 2006.
[10] Etehad Tavakol, M., Saeed Sadri, E. Y. K. Ng, "Application of K-and fuzzy c-means for color segmentation of thermal infrared breast images", Journal of medical systems, Vol.34, Issue.1, pp. 35-42, 2010.
[11] Francesco Masulli, Schenone A, “A fuzzy clustering based segmentation system as support to diagnosis in medical imaging”, Artificial Intelligence in Medicine, Vol.16, Issue.2, pp.129-147, 1999.
[12] Frank Klawonn, “What Can Fuzzy Cluster Analysis Contribute to Clustering of High-Dimensional Data?”, International workshop on Fuzzy Logic and Applications, Springer, Cham,pp.1-47, 2013.
[13] Hongmei Zhang, Guidong Yu, “A Novel Clustering and Mining Algorithm for High Dimensional Data based on Uncertainty Criteria and Fuzzy Mathematics”, Rev. Téc. Ing. Univ. Zulia, Vol.39, Issue.2, pp.1-11, 2016.
[14] Hu Yang, Nicolino J. Pizzi, “Biomedical Data Classification Using Hierarchical Clustering”, Proc IEEE Canadian Conf Elect Comput. Eng, Niagara Falls, Vol.4, pp.1861-1864, 2004.
[15] Jezewski M, “An application of modified fuzzy clustering to medical data classification”, Journal of Medical Informatics and Technologies, Vol.17, pp.51-57, 2011.
[16] Klifa, C., et al. "Quantification of breast tissue index from MR data using fuzzy clustering", Engineering in medicine and biology society, IEEE, Vol.3, pp.1667-70, 2004.
[17] Liu, Xiaoming, et al. "Microcalcification detection in full-field digital mammograms with PFCM clustering and weighted SVM-based method", EURASIP Journal on Advances in Signal Processing, Vol.1, Issue.73, 2015.
[18] Muhic Indira, "Fuzzy analysis of breast cancer disease using fuzzy c-means and pattern recognition", Southeast Europe Journal of Soft Computing, Vol.39, Issue.2, 2013.
[19] Roland Winkler, Frank Klawonn, Rudolf Kruse, “Fuzzy C-Means in High Dimensional Spaces, International Journal of Fuzzy System Applications”, Vol.1, Issue.1, pp.1-16, 2011.
[20] Rousseeuw PJ, “Silhouettes: a Graphical Aid to the Interpretation and Validation of Cluster Analysis”, Journal of Computational and Applied Mathematics, vol.20, pp.53-65, 1987.
[21] Şahan, S., Polat, K., Kodaz, H., & Güneş, S, “A new hybrid method based on fuzzy-artificial immune system and k-nn algorithm for breast cancer diagnosis”, Computers in Biology and Medicine, Vol.37, Issue.3, pp.415-423, 2007.
[22] Senthilkumar, B., and G. Umamaheswari, "Combination of novel enhancement technique and fuzzy c means clustering technique in breast cancer detection", Biomedical Research, Vol.24, Issue.2, pp.252-256, 2013.
[23] Soria, Daniele et al., "A methodology to identify consensus classes from clustering algorithms applied to immunohistochemical data from breast cancer patients", Computers in biology and medicine,Vol. 40, Issue.3, pp.318-330, 2010.
[24] Singh Nalini et al., "GUI Based Automatic Breast Cancer Mass and Calcification Detection in Mammogram Images using K-means and Fuzzy C-means Methods", International Journal of Machine Learning and Computing, Vol.2, Issue.1, pp.7-12, 2012.
[25] D.Vanisri, C.Loganathan, “An Efficient Fuzzy Possibilistic C-Means with Penalized and Compensated Constraints”, Global Journal of Computer Science and Technology, Vol.11, Issue.3, pp.15-22, 2011.
[26] Vivona Letizia, et al., "Fuzzy technique for micro calcifications clustering in digital mammograms", BMC medical imaging, Vol.14, Issue.1, 23, 2014.
[27] Zheng, Bichen, Sang Won Yoon, Sarah S. Lam, "Breast cancer diagnosis based on feature extraction using a hybrid of K-means and support vector machine algorithms", Expert Systems with Applications, Vol.41, Issue.4, pp.1476-1482, 2014.