New Approach to Product Recommendation System by Using Blog Data for E-Commerce Applications
G Kiran Prabhu1 , D Sai Kumar2 , Ayasya V Bulusu3 , E Poojitha4 , Raghavendra Reddy5
Section:Survey Paper, Product Type: Journal Paper
Volume-07 ,
Issue-14 , Page no. 53-58, May-2019
CrossRef-DOI: https://doi.org/10.26438/ijcse/v7si14.5358
Online published on May 15, 2019
Copyright © G Kiran Prabhu, D Sai Kumar, Ayasya V Bulusu, E Poojitha, Raghavendra Reddy . This is an open access article distributed under the Creative Commons Attribution License, which permits unrestricted use, distribution, and reproduction in any medium, provided the original work is properly cited.
View this paper at Google Scholar | DPI Digital Library
How to Cite this Paper
- IEEE Citation
- MLA Citation
- APA Citation
- BibTex Citation
- RIS Citation
IEEE Style Citation: G Kiran Prabhu, D Sai Kumar, Ayasya V Bulusu, E Poojitha, Raghavendra Reddy, “New Approach to Product Recommendation System by Using Blog Data for E-Commerce Applications,” International Journal of Computer Sciences and Engineering, Vol.07, Issue.14, pp.53-58, 2019.
MLA Style Citation: G Kiran Prabhu, D Sai Kumar, Ayasya V Bulusu, E Poojitha, Raghavendra Reddy "New Approach to Product Recommendation System by Using Blog Data for E-Commerce Applications." International Journal of Computer Sciences and Engineering 07.14 (2019): 53-58.
APA Style Citation: G Kiran Prabhu, D Sai Kumar, Ayasya V Bulusu, E Poojitha, Raghavendra Reddy, (2019). New Approach to Product Recommendation System by Using Blog Data for E-Commerce Applications. International Journal of Computer Sciences and Engineering, 07(14), 53-58.
BibTex Style Citation:
@article{Prabhu_2019,
author = {G Kiran Prabhu, D Sai Kumar, Ayasya V Bulusu, E Poojitha, Raghavendra Reddy},
title = {New Approach to Product Recommendation System by Using Blog Data for E-Commerce Applications},
journal = {International Journal of Computer Sciences and Engineering},
issue_date = {5 2019},
volume = {07},
Issue = {14},
month = {5},
year = {2019},
issn = {2347-2693},
pages = {53-58},
url = {https://www.ijcseonline.org/full_spl_paper_view.php?paper_id=1089},
doi = {https://doi.org/10.26438/ijcse/v7i14.5358}
publisher = {IJCSE, Indore, INDIA},
}
RIS Style Citation:
TY - JOUR
DO = {https://doi.org/10.26438/ijcse/v7i14.5358}
UR - https://www.ijcseonline.org/full_spl_paper_view.php?paper_id=1089
TI - New Approach to Product Recommendation System by Using Blog Data for E-Commerce Applications
T2 - International Journal of Computer Sciences and Engineering
AU - G Kiran Prabhu, D Sai Kumar, Ayasya V Bulusu, E Poojitha, Raghavendra Reddy
PY - 2019
DA - 2019/05/15
PB - IJCSE, Indore, INDIA
SP - 53-58
IS - 14
VL - 07
SN - 2347-2693
ER -
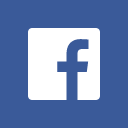
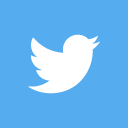
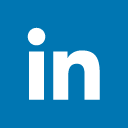
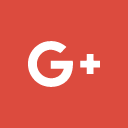
Abstract
Communitarian sifting (CF) calculations have been generally used to manufacture recommender frameworks since they have recognizing ability of sharing aggregate wisdoms and encounters. Notwithstanding, they may effortlessly fall into the snare of the Matthew impact, which will in general prescribe prevalent things and consequently less famous things become progressively less well known. Under this situation, a large portion of the things in the proposal list are now well-known to clients and in this way the execution would truly deteriorate in discovering cold things, i.e., new things and specialty things. To address this issue, a client overview is first directed on the internet shopping propensities in China, in light of which a novel suggestion calculation named trend-setter based CF is recommended that can prescribe cold things to clients by presenting the idea of pioneers. In particular, trend-setters are an extraordinary subset of clients who can find cold things without the assistance of recommender framework. In this way, chilly things can be caught in the suggestion list through trailblazers, accomplishing the harmony among good fortune and precision
Key-Words / Index Term
Recommendation System; E-Commerce Applications; Machine Learning; Sentiment Analysis
References
[1]. J. Wang and Y. Zhang, “Opportunity model for e- commerce recommendation: Right product; right time,” in SIGIR, 2013.
[2]. M. Giering, “Retail sales prediction and item recommenda- tions using customer demographics at store level,” SIGKDD Explor. Newsl., vol. 10, no. 2, Dec.2008.
[3]. G. Linden, B. Smith, and J. York, “Amazon.com recommen- dations: Item-to-item collaborative filtering,” IEEE Internet Computing, vol. 7, no. 1, Jan. 2003.
[4]. A. Zeithaml, “The new demographicsand market fragmen- tation,” Journal of Marketing, vol. 49, pp. 64–75, 1985.
[5]. X. Zhao, Y. Guo, Y. He, H. Jiang, Y. Wu, and X. Li, “We know what you want tobuy: a demographic- based system for product recommendation on microblogs,” in SIGKDD, 2014.
[6]. J. Wang, W. X. Zhao, Y. He, and X. Li, “Leveraging product adopter information from online reviews for product recom- mendation,” in ICWSM, 2015.
[7]. Y. Seroussi, F. Bohnert, and I. Zukerman, “Personalised rating prediction for new users using latent factor models,” in ACM HH, 2011.
[8]. T. Mikolov, I. Sutskever, K. Chen, G. S. Corrado, and J. Dean, “Distributed representations of words and phrases and their compositionality,” in NIPS, 2013.
[9]. Q. V. Le and T. Mikolov, “ Distributed representations of sen- tences and documents,” CoRR, vol. abs/1405.4053, 2014.
[10]. J. Lin, K. Sugiyama, M. Kan, and T. Chua, “Addressing cold start in app recommendation: latent user models constructed from twitter followers,” in SIGIR, 2013.
[11]. T. Mikolov, K. Chen, G. Corrado, and J. Dean, “Efficient estimation of word representations in vector space,” CoRR, vol. abs/1301.3781, 2013.
[12]. Y. Koren, R. Bell, and C. Volinsky, “Matrix factorization techniques forrecommender systems,” Computer, vol. 42, no. 8, pp. 30–37, Aug. 2009.
[13]. Ning Wang, Qiaoling Zhang, Liejun Yang, Mingming Chen, “A Novel E-Commerce Recommendation System Model based on the Pattern Recognition and User Behavior Preference Analysis”, Advanced Science and Technology Letters Vol.138 (ISI 2016), pp.105-110.
[14]. Kota Charishma, , SK. Gopal Krishna, “Connecting OSN Media to E-commerce for Cold Start Product Recommendation using Micro Login Information”, ijitech, ISSN 2321-8665 Vol.06,Issue.01, January-2018, Pages:0014-0016.
[15]. Manish Raka, Prof. Sachin Godse, “Implementing Product Recommendation System using Neural Network by Connection Social Networking to E-Commerce”, IJIRST –International Journal for Innovative Research in Science & Technology, Volume 3, Issue 08, January 2017 ISSN (online): 2349-6010.