Retail Assortment Planning Using Data Science
S.K. Begur1 , K. Gururaj2 , K.K. Bhowmik3 , K. Kumar4 , K. Malik5 , S.A. Rabbani6 , N. Tengli7
Section:Research Paper, Product Type: Journal Paper
Volume-07 ,
Issue-14 , Page no. 12-17, May-2019
CrossRef-DOI: https://doi.org/10.26438/ijcse/v7si14.1217
Online published on May 15, 2019
Copyright © S.K. Begur, K. Gururaj, K.K. Bhowmik, K. Kumar, K. Malik, S.A. Rabbani, N. Tengli . This is an open access article distributed under the Creative Commons Attribution License, which permits unrestricted use, distribution, and reproduction in any medium, provided the original work is properly cited.
View this paper at Google Scholar | DPI Digital Library
How to Cite this Paper
- IEEE Citation
- MLA Citation
- APA Citation
- BibTex Citation
- RIS Citation
IEEE Style Citation: S.K. Begur, K. Gururaj, K.K. Bhowmik, K. Kumar, K. Malik, S.A. Rabbani, N. Tengli, “Retail Assortment Planning Using Data Science,” International Journal of Computer Sciences and Engineering, Vol.07, Issue.14, pp.12-17, 2019.
MLA Style Citation: S.K. Begur, K. Gururaj, K.K. Bhowmik, K. Kumar, K. Malik, S.A. Rabbani, N. Tengli "Retail Assortment Planning Using Data Science." International Journal of Computer Sciences and Engineering 07.14 (2019): 12-17.
APA Style Citation: S.K. Begur, K. Gururaj, K.K. Bhowmik, K. Kumar, K. Malik, S.A. Rabbani, N. Tengli, (2019). Retail Assortment Planning Using Data Science. International Journal of Computer Sciences and Engineering, 07(14), 12-17.
BibTex Style Citation:
@article{Begur_2019,
author = {S.K. Begur, K. Gururaj, K.K. Bhowmik, K. Kumar, K. Malik, S.A. Rabbani, N. Tengli},
title = {Retail Assortment Planning Using Data Science},
journal = {International Journal of Computer Sciences and Engineering},
issue_date = {5 2019},
volume = {07},
Issue = {14},
month = {5},
year = {2019},
issn = {2347-2693},
pages = {12-17},
url = {https://www.ijcseonline.org/full_spl_paper_view.php?paper_id=1080},
doi = {https://doi.org/10.26438/ijcse/v7i14.1217}
publisher = {IJCSE, Indore, INDIA},
}
RIS Style Citation:
TY - JOUR
DO = {https://doi.org/10.26438/ijcse/v7i14.1217}
UR - https://www.ijcseonline.org/full_spl_paper_view.php?paper_id=1080
TI - Retail Assortment Planning Using Data Science
T2 - International Journal of Computer Sciences and Engineering
AU - S.K. Begur, K. Gururaj, K.K. Bhowmik, K. Kumar, K. Malik, S.A. Rabbani, N. Tengli
PY - 2019
DA - 2019/05/15
PB - IJCSE, Indore, INDIA
SP - 12-17
IS - 14
VL - 07
SN - 2347-2693
ER -
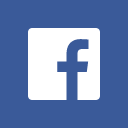
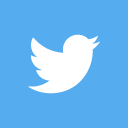
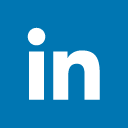
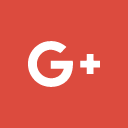
Abstract
This project is based on providing solution to retail giants to address their current Assortment strategy and increase their profit. Assortment AI is an AI project under the trade name reMark which is a social analytical platform of Kigyan Techno Solutions that provides retail marketing solutions. The concept of the project: Today majority of the giant retail companies are facing a lot of issues in their current assortment planning of their products. These include the total dump of products being 45%. This wrong assortment planning leads to products being out of stock which causes loss to businesses and major customer dissatisfaction, also this assortment planning requires a lot of manual strategies which are very costly and hence these assortment strategies then turn out to be costly, time taking, biased and working on mostly non relevant data. Due to the above factors the retail companies have understood that the current assortment planning strategies are not working and are only causing business loss and customer attrition. Hence there is a need of new assortment plan. The project that we are currently working on is building Assortment AI Module in a retail market and so the project is an Artificial Intelligence block which will process the current situation and will build a proper assortment plan. This would then address various problems that the retail companies were facing and present them with proper assortment plan which would include unbiased assortment strategy, the dump would be reduced to less than 20%, increase the customer satisfaction and reduce the customer attrition
Key-Words / Index Term
Kigyan Techno Solutions,data science,attrition,retail,assortment dumpstrategy
References
[1] K. M. Murali, L. Michael, E.K. Barbara, "Why is AssortmentPlanning so Difficult for Retailers? A Framework and Research Agenda", Journal of Retailing, vol. 85, no. 1, pp. 71-83, 2009.Show Context CrossRef Google Scholar.
[2]M. K. Mantrala, K. Manfred, S. Leopold, Krafft Manfred, MuraliK. Mantrala, "Entrepreneurship in Retailing: Leopold Stiefel`s ‘Big Idea’ and the Growth of Media Markt-Saturn" in Retailing in the 21st Century: Current and Emerging Trends, Berlin/Heidelberg/New York:Springer., 2008.
[3]K. Wong, Major Retailers Rev Up Green Campaigns, February 2008.
[4]P.D. Larson, R.A. DeMarais, "Psychic stock: An independent variable category of inventory", International Journal of Physical Distribution and Logistics Management, vol. 20, no. 7, pp. 28-34, 1990.
[5]Albuquerque P, Bronnenberg BJ (2009) Estimating demand heterogeneity using aggregated data: An application to the frozen pizza category. Marketing Sci. 28(2):356–372. [6] David A. Freedman (2009). Statistical Models: Theory and Practice. Cambridge University Press. p. 26. A simple regression equation has on the right hand side an intercept and an explanatory variable with a slope coefficient. A multiple regression equation has two or more explanatory variables on the right hand side, each with its own slope coefficient
.[7] Hilary L. Seal (1967). "The historical development of the Gauss linear model". Biometrika. 54 (1/2): 1–24. doi:10.1093/biomet/54.1-2.1. JSTOR 2333849.
[8] Yan, Xin (2009), Linear Regression Analysis: Theory and Computing, World Scientific, pp. 1–2, ISBN 9789812834119, Regression analysis ... is probably one of the oldest topics in mathematical statistics dating back to about two hundred years ago. The earliest form of the linear regression was the least squares method, which was published by Legendre in 1805, and by Gauss in 1809 ... Legendre and Gauss both applied the method to the problem of determining, from astronomical observations, the orbits of bodies about the sun.