Improving Forecasting Efficiency Using Machine Learning and IoT
Manisha Gaidhane1 , Mona Mulchandani2 , Priyanka Dudhe3
Section:Research Paper, Product Type: Journal Paper
Volume-07 ,
Issue-12 , Page no. 63-66, May-2019
Online published on May 12, 2019
Copyright © Manisha Gaidhane, Mona Mulchandani, Priyanka Dudhe . This is an open access article distributed under the Creative Commons Attribution License, which permits unrestricted use, distribution, and reproduction in any medium, provided the original work is properly cited.
View this paper at Google Scholar | DPI Digital Library
How to Cite this Paper
- IEEE Citation
- MLA Citation
- APA Citation
- BibTex Citation
- RIS Citation
IEEE Style Citation: Manisha Gaidhane, Mona Mulchandani, Priyanka Dudhe, “Improving Forecasting Efficiency Using Machine Learning and IoT,” International Journal of Computer Sciences and Engineering, Vol.07, Issue.12, pp.63-66, 2019.
MLA Style Citation: Manisha Gaidhane, Mona Mulchandani, Priyanka Dudhe "Improving Forecasting Efficiency Using Machine Learning and IoT." International Journal of Computer Sciences and Engineering 07.12 (2019): 63-66.
APA Style Citation: Manisha Gaidhane, Mona Mulchandani, Priyanka Dudhe, (2019). Improving Forecasting Efficiency Using Machine Learning and IoT. International Journal of Computer Sciences and Engineering, 07(12), 63-66.
BibTex Style Citation:
@article{Gaidhane_2019,
author = {Manisha Gaidhane, Mona Mulchandani, Priyanka Dudhe},
title = {Improving Forecasting Efficiency Using Machine Learning and IoT},
journal = {International Journal of Computer Sciences and Engineering},
issue_date = {5 2019},
volume = {07},
Issue = {12},
month = {5},
year = {2019},
issn = {2347-2693},
pages = {63-66},
url = {https://www.ijcseonline.org/full_spl_paper_view.php?paper_id=1044},
publisher = {IJCSE, Indore, INDIA},
}
RIS Style Citation:
TY - JOUR
UR - https://www.ijcseonline.org/full_spl_paper_view.php?paper_id=1044
TI - Improving Forecasting Efficiency Using Machine Learning and IoT
T2 - International Journal of Computer Sciences and Engineering
AU - Manisha Gaidhane, Mona Mulchandani, Priyanka Dudhe
PY - 2019
DA - 2019/05/12
PB - IJCSE, Indore, INDIA
SP - 63-66
IS - 12
VL - 07
SN - 2347-2693
ER -
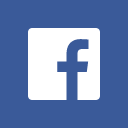
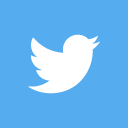
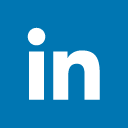
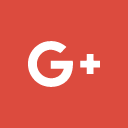
Abstract
Forecasting can be defined as prediction of what is going to happen in the future by analyzing the past and current available data. It can be done for power, weather, business, company management, economics, investors etc. Although it varies based on the area for which it is going to be applied. Forecasting has technical and business impacts. If it is not done properly, it can cause inefficient usage of resources. In traditional load forecasting, predicting future demands is a quite time consuming and sometimes it results in the incorrect output. To overcome these challenges, new generation technologies should be utilized such as internet of things, cloud computing, and machine learning. It can also help in improving existing established systems. The purpose of this study paper is to know, participation of new technologies in improving the efficiency of forecasting. Forecasting using machine-learning and IoT would really help to achieve high forecast accuracy. In machine learning forecasting, processors learn from mining loads of cloud data without human intervention to fulfill the demand. While doing this paper, by the manner of literature review, first, the trend of improvement, diversification and the new characteristics of the system will be evaluated. Then, the forecasting technology will be reviewed and analyzed from two different aspects, elementary analysis and application research. This review paper study will help to create a new system idea that would provide more accurate forecasting with reduction in time consumption.
Key-Words / Index Term
Internet of Things, machine learning, cloud data, forecasting, load
References
[1] A. Yousuf Saber, “IoT based Online Load Forecasting”, 2017 Ninth Annual IEEE Green Technologies Conference, Denver, CO, USA 978-1-5090-4535-8, 2017.
[2] Chandini M.S, Pushpalatha R, Dr. R. Boraiah, “A Brief study on Prediction of Load in Cloud Environment”, International Journal of Advanced Research in Computer and Communication Engineering Vol. 5, Issue 5, May 2016
[3] J. Kennedy and R. C. Eberhart, “Particle swarm optimization,” in Proc.IEEE Int. Conf. Neural Networks, 1995, vol. 4, pp. 1942–1948.
[4] K. Lru, S. Subbarayan, R. R. Shoults, M. T. Manry, C. Kwan, F. L. lewis, and J. Naccarino, “Comparison of very short term load forecasting techniques,” IEEE Trans. Power Syst., vol. 11, no. 2, pp. 877-882, May 1996.
[5] I. Drezga and S. Rahman,, “Short-term load forecasting with local ANN predictors,” IEEE Trans. Power Syst., vol. 14, no. 3, pp. 844–850, Aug 1999.
[6] D. Alex and C. Timothy, “A regression-based approach to short term system load forecasting,” IEEE Trans. Power Syst., vol. 5, no. 4, pp. 1535–1550, Nov 1990.
[7] S. Rahman and O. Hazim, “A generalized knowledge-based short-term load forecasting technique”, IEEE Trans. Power Syst., vol. 8, no. 2, pp. 508-514, May 1993.
[8] B. J. Chen, M. W. Chang, and C. J. Lin, “Load forecasting using support vector machines: A study on EUNITE competition 2001,” IEEE Trans. Power Syst., vol. 19, no. 4, pp. 1821–1830, Nov 2004.
[9] I. Drezga and S. Rahman,, “Short-term load forecasting with local ANN predictors,” IEEE Trans. Power Syst., vol. 14, no. 3, pp. 844–850, Aug 1999.
[10] T. A. Farrag, and E. E. Elattar “Cloud Computing for Short Term Load Forecasting Based on Machine Learning Technique” International Journal of Applied Engineering Research ISSN 0973-4562 Volume 12, Number 19 (2017) pp. 8689-8695
[11] S. Makridakis, E. Spiliotis, V. Assimakopoulos “Statistical and Machine Learning forecasting methods: Concerns and ways forward” Research article