Random Forest for Multitemporal and Multiscale Classification of Remote Sensing Satellite Imagery
A. Chitradevi1 , S. Vijayalakshmi2
Section:Research Paper, Product Type: Journal Paper
Volume-4 ,
Issue-2 , Page no. 59-65, Feb-2016
Online published on Feb 29, 2016
Copyright © A. Chitradevi , S. Vijayalakshmi . This is an open access article distributed under the Creative Commons Attribution License, which permits unrestricted use, distribution, and reproduction in any medium, provided the original work is properly cited.
View this paper at Google Scholar | DPI Digital Library
How to Cite this Paper
- IEEE Citation
- MLA Citation
- APA Citation
- BibTex Citation
- RIS Citation
IEEE Style Citation: A. Chitradevi , S. Vijayalakshmi, “Random Forest for Multitemporal and Multiscale Classification of Remote Sensing Satellite Imagery,” International Journal of Computer Sciences and Engineering, Vol.4, Issue.2, pp.59-65, 2016.
MLA Style Citation: A. Chitradevi , S. Vijayalakshmi "Random Forest for Multitemporal and Multiscale Classification of Remote Sensing Satellite Imagery." International Journal of Computer Sciences and Engineering 4.2 (2016): 59-65.
APA Style Citation: A. Chitradevi , S. Vijayalakshmi, (2016). Random Forest for Multitemporal and Multiscale Classification of Remote Sensing Satellite Imagery. International Journal of Computer Sciences and Engineering, 4(2), 59-65.
BibTex Style Citation:
@article{Chitradevi_2016,
author = {A. Chitradevi , S. Vijayalakshmi},
title = {Random Forest for Multitemporal and Multiscale Classification of Remote Sensing Satellite Imagery},
journal = {International Journal of Computer Sciences and Engineering},
issue_date = {2 2016},
volume = {4},
Issue = {2},
month = {2},
year = {2016},
issn = {2347-2693},
pages = {59-65},
url = {https://www.ijcseonline.org/full_paper_view.php?paper_id=795},
publisher = {IJCSE, Indore, INDIA},
}
RIS Style Citation:
TY - JOUR
UR - https://www.ijcseonline.org/full_paper_view.php?paper_id=795
TI - Random Forest for Multitemporal and Multiscale Classification of Remote Sensing Satellite Imagery
T2 - International Journal of Computer Sciences and Engineering
AU - A. Chitradevi , S. Vijayalakshmi
PY - 2016
DA - 2016/02/29
PB - IJCSE, Indore, INDIA
SP - 59-65
IS - 2
VL - 4
SN - 2347-2693
ER -
![]() |
![]() |
![]() |
1726 | 1617 downloads | 1539 downloads |
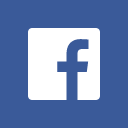
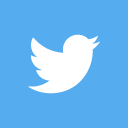
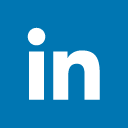
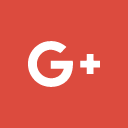
Abstract
An increasing number of optical High-Resolution (HR) remote sensing satellite systems, offering multispectral images. However, acquiring multi temporal HR data may not always be economically viable, particularly for large areas. Data having medium resolution (i.e., a GSD of 30 m) do not offer as much detail, but cover a larger area and may often be preferable from an economical point of view. In this research work present a new method for the multi temporal and contextual classification of georeferenced optical remote sensing images acquired at different epochs with having different geometrical resolutions. The method is based on Conditional Random Fields (CRFs) for contextual classification. But in CRF, pool of features used in this work is rather limited, particularly for the medium-resolution images. To solve this problem proposed work is expanded to pool of features for the medium-resolution images to improve the classification results. The Gaussian model used in the CRF is should be replaced by more sophisticated Random Forests (RFs) classifiers. RF is an ensemble of many decision trees, which have been trained on randomly selected pool of features for the medium-resolution images subsets of the training data, in order to decorrelate the individual trees. Extend such a framework to multitemporal classification and change detection, taking into account interactions between images acquired at different epochs and considering the fact that these images may have different geometrical resolutions. Results are given for two different test sites in Germany, where Ikonos, RapidEye, and Landsat images are available. State-of-the-art multitemporal classification method and that it is feasible to detect changes in lower resolution images.
Key-Words / Index Term
Remote sensing satellite;Multitemporal classification;Random forest classifier
References
[1]. Schowengerdt, Robert A. (2007). Remote sensing: models and methods for image processing (3rd ed.). Academic Press. p. 2.
[2]. Schott, John Robert (2007). Remote sensing: the image chain approach (2nd ed.). Oxford University Press. p. 1.
[3]. Guo, H., Huang, Q., Li, X., Sun, Z., & Zhang, Y. (2014). Spatiotemporal analysis of urban environment based on the vegetation–impervious surface–soil model. Journal of Applied Remote Sensing, 8(1), 084597-084597.
[4]. Liu, Jian Guo & Mason, Philippa J. (2009). Essential Image Processing for GIS and Remote Sensing. Wiley-Blackwell. p. 4.
[5]. http://hurricanes.nasa.gov/earth-sun/technology/remote_sensing.html
[6]. Mills, J. P., Newton, I., & Twiss, S. D. (1997). Photogrammetry from archived digital imagery for seal monitoring. The Photogrammetric Record, 15(89), 715-724.
[7]. Twiss, S. D., Thomas, C. J., & Pomeroy, P. P. (2001). Topographic spatial characterisation of grey seal Halichoerus grypus breeding habitat at a subseal size spatial grain. Ecography, 24(3), 257-266.
[8]. Stewart, J. E., Pomeroy, P. P., Duck, C. D., & Twiss, S. D. (2014). Finescale ecological niche modeling provides evidence that lactating gray seals (Halichoerus grypus) prefer access to fresh water in order to drink. Marine Mammal Science, 30(4), 1456-1472.
[9]. Begni, G., Escadafal, R., Fontannaz, D., & Hong-Nga Nguyen, A. T. (2005). Remote sensing: a tool to monitor and assess desertification. Les dossiers thématiques du CSFD, 2, 44.
[10]. S. Kumar and M. Hebert, “Discriminative random fields,” Int. J. Comput. Vis., vol. 68, no. 2, pp. 179–201, Jun. 2006.
[11]. K. Schindler, “An overview and comparison of smooth labeling methods for land-cover classification,” IEEE Trans. Geosci. Remote Sens., vol. 50, no. 11, pp. 4534–4545, Nov. 2012.
[12]. He, X.; Zemel, R.S.; Carreira-Perpinñán, M.A. (2004). "Multiscale conditional random fields for image labeling". IEEE Computer Society. CiteSeerX: 10.1.1.3.7826.
[13]. Sha, F., & Pereira, F. (2003, May). Shallow parsing with conditional random fields. In Proceedings of the 2003 Conference of the North American Chapter of the Association for Computational Linguistics on Human Language Technology-Volume 1 (pp. 134-141). Association for Computational Linguistics.
[14]. Settles, B. (2004, August). Biomedical named entity recognition using conditional random fields and rich feature sets. In Proceedings of the International Joint Workshop on Natural Language Processing in Biomedicine and its Applications (pp. 104-107). Association for Computational Linguistics.
[15]. Chang, K. Y., Lin, T. P., Shih, L. Y., & Wang, C. K. (2015). Analysis and Prediction of the Critical Regions of Antimicrobial Peptides Based on Conditional Random Fields. PloS one, 10(3).
[16]. Sutton, C., & McCallum, A. (2011). An introduction to conditional random fields. Machine Learning, 4(4), 267-373.
[17]. Yang, M. Y., Förstner, W., & Drauschke, M. (2010). Hierarchical Conditional Random Field for Multi-class Image Classification. In VISAPP (2) (pp. 464-469).
[18]. Schindler, K. (2012). An overview and comparison of smooth labeling methods for land-cover classification. IEEE Transactions on Geoscience and Remote Sensing, 50(11), 4534-4545.
[19]. Jianya, G., Haigang, S., Guorui, M., & Qiming, Z. (2008). A review of multi-temporal remote sensing data change detection algorithms. The International Archives of the Photogrammetry, Remote Sensing and Spatial Information Sciences, 37(B7), 757-762.
[20]. Choi, M. J., Chandrasekaran, V., Malioutov, D. M., Johnson, J. K., & Willsky, A. S. (2008). Multiscale stochastic modeling for tractable inference and data assimilation. Computer Methods in Applied Mechanics and Engineering, 197(43), 3492-3515.
[21]. Hoberg, T., Rottensteiner, F., & Heipke, C. (2011, November). Classification of multitemporal remote sensing data of different resolution using conditional random fields. 2011 IEEE International Conference In Computer Vision Workshops (ICCV Workshops), (pp. 235-242).
[22]. Zhong, P., & Wang, R. (2007). A multiple conditional random fields ensemble model for urban area detection in remote sensing optical images. Geoscience and Remote Sensing, IEEE Transactions on, 45(12), 3978-3988.
[23]. Szeliski, R., Zabih, R., Scharstein, D., Veksler, O., Kolmogorov, V., Agarwala, A., ... & Rother, C. (2008). A comparative study of energy minimization methods for markov random fields with smoothness-based priors. IEEE Transactions on Pattern Analysis and Machine Intelligence, 30(6), 1068-1080.
[24]. Lu, W. L., Murphy, K. P., Little, J. J., Sheffer, A., & Fu, H. (2009). A hybrid conditional random field for estimating the underlying ground surface from airborne lidar data. IEEE Transactions on Geoscience and Remote Sensing, 47(8), 2913-2922.
[25]. D. Lu, P. Mausel, E. Brondizio, and E. Moran, “Change detection techniques,” Int. J. Remote Sens., vol. 25, no. 12, pp. 2365–2401, Jun. 2004.
[26]. Benedek, C., & Szirányi, T. (2009). Change detection in optical aerial images by a multilayer conditional mixed Markov model. Geoscience and Remote Sensing, IEEE Transactions on, 47(10), 3416-3430.
[27]. D. Li, “Remotely sensed images and GIS data fusion for automatic change detection,” Int. J. Image Data Fusion, vol. 1, no. 1, pp. 99–108, Mar. 2010.
[28]. J. Wegner, U. Sörgel, and B. Rosenhahn, “Segment-based building detection with conditional random fields,” in Proc. 6th IEEE/GRSS/ISPRS Joint Urban Remote Sens. Event, 2011, pp. 205–208.
[29]. P. B. C. Leite, R. Q. Feitosa, A. R. Formaggio, G. A. O. P. Costa, and K. Pakzad, “Hidden Markov models for crop recognition in remote sensing image sequences,” Pattern Recognit. Lett., vol. 32, no. 1, pp. 19–26, Jan. 2011.
[30]. J. Shotton, J. Winn, C. Rother, and A. Criminisi, “TextonBoost for image understanding: Multi-class object recognition and segmentation by jointly modeling texture, layout, and context,” Int. J. Comput. Vis., vol. 81, no. 1, pp. 2–23, Jan. 2009.
[31]. P. O. Gislason, J. A. Benediktsson, and J. R. Sveinsson, “Random forests for land cover classification,” Pattern Recognit. Lett., vol. 27, no. 4, pp. 294–300, Mar. 2006.
[32]. J. Shotton, M. Johnson, and R. Cipolla, “Semantic texton forests for image categorization and segmentation,” in Proc. IEEE Conf. Comput. Vis. Pattern Recognit., Anchorage, AK, 2008, pp. 1–8.