Re-Ranking of Images Using Semantic Signatures with Queries
B. UshaRani1 , M.V.S.N.Maheswar 2
Section:Review Paper, Product Type: Journal Paper
Volume-3 ,
Issue-10 , Page no. 71-75, Oct-2015
Online published on Oct 31, 2015
Copyright © B. UshaRani , M.V.S.N.Maheswar . This is an open access article distributed under the Creative Commons Attribution License, which permits unrestricted use, distribution, and reproduction in any medium, provided the original work is properly cited.
View this paper at Google Scholar | DPI Digital Library
How to Cite this Paper
- IEEE Citation
- MLA Citation
- APA Citation
- BibTex Citation
- RIS Citation
IEEE Style Citation: B. UshaRani , M.V.S.N.Maheswar, “Re-Ranking of Images Using Semantic Signatures with Queries,” International Journal of Computer Sciences and Engineering, Vol.3, Issue.10, pp.71-75, 2015.
MLA Style Citation: B. UshaRani , M.V.S.N.Maheswar "Re-Ranking of Images Using Semantic Signatures with Queries." International Journal of Computer Sciences and Engineering 3.10 (2015): 71-75.
APA Style Citation: B. UshaRani , M.V.S.N.Maheswar, (2015). Re-Ranking of Images Using Semantic Signatures with Queries. International Journal of Computer Sciences and Engineering, 3(10), 71-75.
BibTex Style Citation:
@article{UshaRani_2015,
author = {B. UshaRani , M.V.S.N.Maheswar},
title = {Re-Ranking of Images Using Semantic Signatures with Queries},
journal = {International Journal of Computer Sciences and Engineering},
issue_date = {10 2015},
volume = {3},
Issue = {10},
month = {10},
year = {2015},
issn = {2347-2693},
pages = {71-75},
url = {https://www.ijcseonline.org/full_paper_view.php?paper_id=707},
publisher = {IJCSE, Indore, INDIA},
}
RIS Style Citation:
TY - JOUR
UR - https://www.ijcseonline.org/full_paper_view.php?paper_id=707
TI - Re-Ranking of Images Using Semantic Signatures with Queries
T2 - International Journal of Computer Sciences and Engineering
AU - B. UshaRani , M.V.S.N.Maheswar
PY - 2015
DA - 2015/10/31
PB - IJCSE, Indore, INDIA
SP - 71-75
IS - 10
VL - 3
SN - 2347-2693
ER -
![]() |
![]() |
![]() |
2422 | 2348 downloads | 2361 downloads |
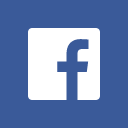
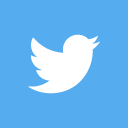
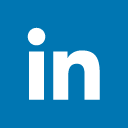
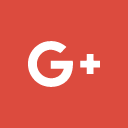
Abstract
Image re-ranking, is an emphatic way to advance the results of web-based image search and has been accept by current economic search engines such as Bing and Google. When a query keyword is given, a list of images are first redeem based on textual dossier given by the user. By asking the user to select a query image from the pool of images, the remaining images are re-ranked based on their index with the query image. A major contempt is that sometimes semantic meanings may clarify user’s search intention. Many people recently expected to match images in a semantic space which used attributes or mention classes closely associated to the semantic meanings of images as basis. In this paper, we introduce a novel image re -ranking framework, in which axiomatically offline learns different linguistic spaces for different query keywords and displays with the image particulars in the form of augmented images. The images are envisaged into their associated semantic spaces to get semantic signatures with the help of one click feedback from the user. At the online stage, images are re-ranked by analyze their semantic signatures access from the semantic space described by the query keyword given by the user. The expected query-specific semantic signatures significantly advance both the efficiency and capability of image re-ranking. Experimental results show that 25-40 percent related improvement has been accomplished on re-ranking precisions correlated with the state-of-the-art methods.
Key-Words / Index Term
Image search, Image re-ranking, Semantic space, Semantic signature, Keyword extension, One click feedback
References
[1] Xiaogang Wang, Member, IEEE , Shi Qiu, Ke Liu, and Xiaoou Tang, Fellow, IEEE, “Web Image Re-Ranking Using Query-Specific Semantic Signatures,” IEEE transactions on pattern analysis and machine intelligence, vol. 36, no. 4, april 2014
[2] R. Datta, D. Joshi, and J.Z. Wang, “Image Retrieval: Ideas, Influences, and Trends of the New Age,” ACM Computing Surveys, vol. 40, article 5, 2007.
[3] A.W.M. Smeulders, M. Worring, S. Santini, A. Gupta, and R. Jain, “Content-Based Image Retrieval,” IEEE Trans. Pattern Analysis and Machine Intelligence, vol. 22, no. 12, pp. 1349-1380, Dec. 2000.
[4] Y. Rui, T.S. Huang, M. Ortega, and S. Mehrotra, “Relevance Feedback: A Power Tool for Interactive Content-Based Image Retrieval,” IEEE Trans. Circuits and Systems for Video Technology, vol. 8, no. 5, pp. 644-655, Sept. 1998.
[5] X.S. Zhou and T.S. Huang, “Relevance Feedback in Image Retrieval: A Comprehensive Review,” Multimedia Systems, vol. 8, pp. 536-544, 2003.
[6] D. Tao, X. Tang, X. Li, and X. Wu, “Asymmetric Bagging and Random Subspace for Support Vector Machines-Based Relevance Feedback in Image Retrieval,” IEEE Trans. Pattern Analysis and Machine Intelligence, vol. 28, no. 7, pp. 1088-1099, July 2006.
[7] J. Cui, F. Wen, and X. Tang, “Real Time Google and Live Image Search Re-Ranking,” Proc. 16th ACM Int’l Conf. Multimedia, 2008.
[8] J. Cui, F. Wen, and X. Tang, “Intent Search: Interactive on-Line Image Search Re-Ranking,” Proc. 16th ACM Int’l Conf. Multimedia, 2008.
[9] X. Tang, K. Liu, J. Cui, F. Wen, and X. Wang, “Intent Search: Capturing User Intention for One-Click Internet Image Search,” IEEE Trans. Pattern Analysis and Machine Intelligence, vol. 34, no. 7, pp. 1342-1353, July 2012.
[10] W. Hsu, L. Kennedy, and S.F. Chang, “Video Search Re-ranking via Information Bottleneck Principle,” Proc. 14th Ann. ACM Int’l Conf. Multimedia, 2006.
[11] D. Tao, X. Tang, X. Li, and X. Wu, “Asymmetric Bagging and Random Subspace for Support Vector Machines-Based Relevance Feedback in Image Retrieval,” IEEE Trans. Pattern Analysis and Machine Intelligence, vol. 28, no. 7, pp. 1088-1099, July 2006.
[12] Y. Rui, T.S. Huang, and S. Mehrotra, ”Content-Based Image Retrieval with Relevance Feedback in MARS,” Proc. IEEE Int’l Conf. Image Processing, vol. 2, pp. 815-818, 1997.
[13] G. Cauwenberghs and T. Poggio, “Incremental and Decremental Support Vector Machine Learning,” Proc. Advances in Neural Information Processing Systems (NIPS), 2001.
[14] J.C. Platt, “Fast Training of Support Vector Machines Using Sequential Minimum Optimization,” in Sch¨olkopf, Burges and Smola, Eds., Advances in Kernel Methods– Support VectorLearning, Cambridge MA: MIT Press, 1998, pp 185-208.
[15] T.-T. Frieß, N. Cristianini and C. Campbell, “The Kernel Adatron Algorithm: A Fast and Simple Learning Procedure for Support Vector Machines,” in 15th Int. Conf. Machine Learning,Morgan Kaufman, 1998.
[16] L. Torresani, M. Szummer, and A. Fitzgibbon, “Efficient Object Category Recognition using Classemes,” Proc. European Conf. Computer Vision (ECCV), 2010.
[17] J. Lui, B. Kuipers, and S. Savarese, “Recognizing Human Actions by Attributes,” Proc. IEEE Conf. Computer Vision and Pattern Recognition (CVPR), 2011.
[18] M. Sahami and T.D. Heilman, “A Web-Based Kernel Function for Measuring the Similarity of Short Text Snippets,” Proc. 15th Int’l Conf. World Wide Web (WWW), 2006.
[19] W.J. Scheirer, N. Kumar, P.N. Belhumeur, and T.E. Boult, “Multi-Attribute Spaces: Calibration for Attribute Fusion and Similarity Search,” Proc. IEEE Conf. Computer Vision and Pattern Recognition (CVPR), 2012.