Conservative Procedures for Web Image Re-Ranking Precisions Using Semantic Signatures
P. Amani1 , Maddali M. V. M. Kumar2
Section:Research Paper, Product Type: Journal Paper
Volume-3 ,
Issue-9 , Page no. 7-10, Sep-2015
Online published on Oct 01, 2015
Copyright © P. Amani , Maddali M. V. M. Kumar . This is an open access article distributed under the Creative Commons Attribution License, which permits unrestricted use, distribution, and reproduction in any medium, provided the original work is properly cited.
View this paper at Google Scholar | DPI Digital Library
How to Cite this Paper
- IEEE Citation
- MLA Citation
- APA Citation
- BibTex Citation
- RIS Citation
IEEE Style Citation: P. Amani , Maddali M. V. M. Kumar, “Conservative Procedures for Web Image Re-Ranking Precisions Using Semantic Signatures,” International Journal of Computer Sciences and Engineering, Vol.3, Issue.9, pp.7-10, 2015.
MLA Style Citation: P. Amani , Maddali M. V. M. Kumar "Conservative Procedures for Web Image Re-Ranking Precisions Using Semantic Signatures." International Journal of Computer Sciences and Engineering 3.9 (2015): 7-10.
APA Style Citation: P. Amani , Maddali M. V. M. Kumar, (2015). Conservative Procedures for Web Image Re-Ranking Precisions Using Semantic Signatures. International Journal of Computer Sciences and Engineering, 3(9), 7-10.
BibTex Style Citation:
@article{Amani_2015,
author = {P. Amani , Maddali M. V. M. Kumar},
title = {Conservative Procedures for Web Image Re-Ranking Precisions Using Semantic Signatures},
journal = {International Journal of Computer Sciences and Engineering},
issue_date = {9 2015},
volume = {3},
Issue = {9},
month = {9},
year = {2015},
issn = {2347-2693},
pages = {7-10},
url = {https://www.ijcseonline.org/full_paper_view.php?paper_id=631},
publisher = {IJCSE, Indore, INDIA},
}
RIS Style Citation:
TY - JOUR
UR - https://www.ijcseonline.org/full_paper_view.php?paper_id=631
TI - Conservative Procedures for Web Image Re-Ranking Precisions Using Semantic Signatures
T2 - International Journal of Computer Sciences and Engineering
AU - P. Amani , Maddali M. V. M. Kumar
PY - 2015
DA - 2015/10/01
PB - IJCSE, Indore, INDIA
SP - 7-10
IS - 9
VL - 3
SN - 2347-2693
ER -
![]() |
![]() |
![]() |
2645 | 2530 downloads | 2403 downloads |
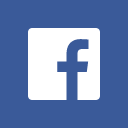
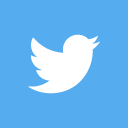
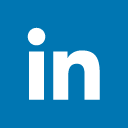
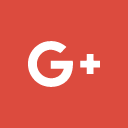
Abstract
Image re-ranking, as an effectual way to get better the outputs of web-based image search, has been legitimate by existing mercantile search engines such as Bing and Google. Specified a query keyword, a pond of images is first cultivated based on textual in sequence. By inquisitive the users to pick a query image from the pool, the outstanding pictures are re-ranked based on their ocular concurrences with the query image. A most important confront is that the correspondences of ocular features do not glowing correlate with images’ semantic meanings which construe users’ search intention. In recent time’s people wished-for to match pictures in a semantic space which worn essences or orientation classes closely allied to the semantic meanings of pictures as basis. However, wisdom a universal visual semantic space to illustrate extremely varied images from the web is difficult and ineffective. We put forward a novel image re-ranking context, which routinely offline learns diverse semantic spaces for dissimilar query keywords. The ocular features of pictures are predicted keen on their related semantic spaces to acquire semantic signatures. On the internet arena, images are re-ranked by examine their semantic signatures accomplish from the semantic space specified by the query keyword. The wished-for query-specific semantic signatures appreciably get better both the accurateness and efficiency of image re-ranking. The pioneering visual characteristics of thousands of proportions can be predicted to the semantic signatures as squat as 25 dimensions. Preliminary results show that 25-40 percent relative enrichment has been accomplished on re-ranking precisions contrasted with the state-of-the-art approaches.
Key-Words / Index Term
Image Search, Semantic Space, Semantic Signature, Context, Query Image, Query Keyword
References
[1] Y. Cao, C. Wang, Z. Li, L. Zhang, and L. Zhang. “Spatial-bag-of features.” In Proc. CVPR, 2010.
[2] Bart and S. Ullman. “Single-example learning of novel classes using representation by similarity.” In Proc. BMVC, 2005.
[3] B. Luo, X. Wang, and X. Tang. “A world wide web based image search engine using text and image content features.” In Proceedings of the SPIE Electronic Imaging, 2003.
[4] J. Cui, F. Wen, and X. Tang, “Intent Search: Interactive on-Line Image Search Re-Ranking,” Proc. 16th ACM Int’l Conf. Multimedia, 2008.
[5] J. Cui, F. Wen, and X. Tang, “Real Time Google and Live Image Search Re-Ranking,” Proc. 16th ACM Int’l Conf. Multimedia, 2008.
[6] C. Lampert, H. Nickisch, and S. Harmeling. “Learning to detect unseen object classes by between-class attribute transfer.” In Proc. CVPR, 2005.
[7] J. Philbin, M. Isard, J. Sivic, and A. Zisserman. “Descriptor Learning for Efficient Retrieval.” In Proc. ECCV, 2010.
[8] N. Rasiwasia, P. J. Moreno, and N. Vasconcelos. “Bridging the gap: Query by semantic example”. IEEE Trans. On Multimedia, 2007.
[9] M. Rohrbach, M. Stark, G. Szarvas, I. Gurevych, and B. Schiele. “What helps where and why? Semantic relatedness for knowledge transfer”. In Proc. CVPR, 2010.
[10] Q. Yin, X. Tang, and J. Sun. “An associate-predict model for face recognition”. In Proc. CVPR, 2011.
[11] Lowe. “Distinctive image features from scale-invariant key points”. Int’l Journal of Computer Vision, 2004.
[12] Xiaogang Wang, Shi Qiu, Ke Liu, and Xiaoou Tang, “Web Image Re-Ranking Using Query-Specific Semantic Signatures”, IEEE Transactions on Pattern Analysis and Machine Intelligence, vol. 36, no. 4, April 2014.