Detection of DDoS Attack Using UCLA Dataset on Different Classifiers
Aakriti Aggarwal1 , Ankur Gupta2
Section:Research Paper, Product Type: Journal Paper
Volume-3 ,
Issue-8 , Page no. 33-36, Aug-2015
Online published on Aug 31, 2015
Copyright © Aakriti Aggarwal , Ankur Gupta . This is an open access article distributed under the Creative Commons Attribution License, which permits unrestricted use, distribution, and reproduction in any medium, provided the original work is properly cited.
View this paper at Google Scholar | DPI Digital Library
How to Cite this Paper
- IEEE Citation
- MLA Citation
- APA Citation
- BibTex Citation
- RIS Citation
IEEE Style Citation: Aakriti Aggarwal , Ankur Gupta, “Detection of DDoS Attack Using UCLA Dataset on Different Classifiers,” International Journal of Computer Sciences and Engineering, Vol.3, Issue.8, pp.33-36, 2015.
MLA Style Citation: Aakriti Aggarwal , Ankur Gupta "Detection of DDoS Attack Using UCLA Dataset on Different Classifiers." International Journal of Computer Sciences and Engineering 3.8 (2015): 33-36.
APA Style Citation: Aakriti Aggarwal , Ankur Gupta, (2015). Detection of DDoS Attack Using UCLA Dataset on Different Classifiers. International Journal of Computer Sciences and Engineering, 3(8), 33-36.
BibTex Style Citation:
@article{Aggarwal_2015,
author = {Aakriti Aggarwal , Ankur Gupta},
title = {Detection of DDoS Attack Using UCLA Dataset on Different Classifiers},
journal = {International Journal of Computer Sciences and Engineering},
issue_date = {8 2015},
volume = {3},
Issue = {8},
month = {8},
year = {2015},
issn = {2347-2693},
pages = {33-36},
url = {https://www.ijcseonline.org/full_paper_view.php?paper_id=604},
publisher = {IJCSE, Indore, INDIA},
}
RIS Style Citation:
TY - JOUR
UR - https://www.ijcseonline.org/full_paper_view.php?paper_id=604
TI - Detection of DDoS Attack Using UCLA Dataset on Different Classifiers
T2 - International Journal of Computer Sciences and Engineering
AU - Aakriti Aggarwal , Ankur Gupta
PY - 2015
DA - 2015/08/31
PB - IJCSE, Indore, INDIA
SP - 33-36
IS - 8
VL - 3
SN - 2347-2693
ER -
![]() |
![]() |
![]() |
2705 | 2562 downloads | 2405 downloads |
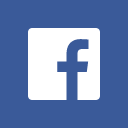
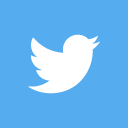
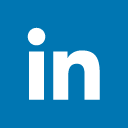
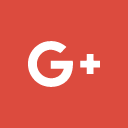
Abstract
Distributed denial of service attack have strong Impact on security of internet because these attacks affects the normal functioning causing loss of billions of dollars. DDoS is very harmful to network as it delays the legitimate users from excessing the server. However these networks were well equipped in security yet they were damaged by DDoS attack. In this paper, the proposed system presents both detecting and classifying schemes of DDoS attack using K-NN, SVM and Naïve Bayesian. The algorithms are developed by using various features of attack packets. By studying the incoming and outgoing network traffic and different classifiers are used to analyze these features. The main objective of this paper is to study classifiers on one dataset for DDoS attack.
Key-Words / Index Term
DDoS attack, Internet Securities, Attack Packet
References
[1]. H. F. Lipson, “Tracking and Tracing” Cyber Attacks: Technical Challenges and Global Policy Issues”, CERT Coordination Centre, Special Report: CMU/SEI-2002-SR-009, 2002
[2]. N. Stephen and N. Judy, Network Intrusion Detection, 3nd ed., New Riders Publishing, United States of America, 2002.
[3]. A. D. Basheer Nayef, “Mitigation and traceback countermeasures for DDoS attacks”, Iowa State University, 2005. .
[4]. Chen, Y. Hwang, K., W. S. Ku, “Distributed change-point detection of DDoS attacks over multiple network domains.” Proceedings of the IEEE International Symposium on Collaborative Technologies and Systems, Las Vegas, NV, 14-17 May. IEEE CS, 2006, pp. 543–550.
[5]. L. Limwiwatkul, A. Rungsawang, “ Distributed denial of service detection using TCP/IP header and traffic measurement analysis”, Proceedings of the IEEE International Symposium Communications and Information Technology, Sapporo, Japan, 26-29 October, IEEE CS, 2006, , pp. 605–610.
[6]. Lee, Juhyun Kim, Ki Hoon Kwon, Younggoo Han, Sehun Kim, “DDoS attack detection method using Cluster analysis”, Expert System with Applications 34, 2008, pp.1659-1665.
[7]. K. Reyhaneh, F. Ahmad, “An Anomaly-Based Method for DDoS Attacks Detection using RBF Neural Networks”, International Conference on Network and Electronics Engineering IPCST vol.11, 2011, IACSIT Press, Singapore.
[8]. Cristóbal Romero, Sebastián Ventura, Pedro G. Espejo and César Hervás,” Data Mining Algorithms to Classify Students”.
[9]. UCLA CSD packet traces. http://www.lasr.cs.ucla.edu/ddos/traces/public/usc.
[10]. N. Abirami, T. Kamalakannan and Dr. A. Muthukumaravel ,” A Study on Analysis of Various Data mining Classification Techniques on Healthcare Data” International Journal of Emerging Technology and Advanced Engineering, Volume 3, Issue 7, July 2013,pp.604-607.
[11]. K. Wisaeng,”A Comparison of Different Classification Techniques for Bank Direct Marketing”, International Journal of Soft Computing and Engineering, Volume-3, Issue-4, September 2013, pp. 116-119.
[12]. S. Archana and Dr. K. Elangovan, “Survey of Classification Techniques in Data Mining”, International Journal of Computer Science and Mobile Applications, Vol.2 Issue. 2, February-2014, pg. 65-71.