A Survey of DDoS Attack Detection Schemes: Methods, Challenges, and Datasets
V. Sughanthini1 , P. Bharathisindhu2
- Dept. of Computer Science, Vellalar College for Women in Thindal, Erode, India.
- Dept. of Computer Science, Vivekanandha Arts and Science College for Women, India.
Section:Survey Paper, Product Type: Journal Paper
Volume-12 ,
Issue-4 , Page no. 68-74, Apr-2024
CrossRef-DOI: https://doi.org/10.26438/ijcse/v12i4.6874
Online published on Apr 30, 2024
Copyright © V. Sughanthini, P. Bharathisindhu . This is an open access article distributed under the Creative Commons Attribution License, which permits unrestricted use, distribution, and reproduction in any medium, provided the original work is properly cited.
View this paper at Google Scholar | DPI Digital Library
How to Cite this Paper
- IEEE Citation
- MLA Citation
- APA Citation
- BibTex Citation
- RIS Citation
IEEE Style Citation: V. Sughanthini, P. Bharathisindhu, “A Survey of DDoS Attack Detection Schemes: Methods, Challenges, and Datasets,” International Journal of Computer Sciences and Engineering, Vol.12, Issue.4, pp.68-74, 2024.
MLA Style Citation: V. Sughanthini, P. Bharathisindhu "A Survey of DDoS Attack Detection Schemes: Methods, Challenges, and Datasets." International Journal of Computer Sciences and Engineering 12.4 (2024): 68-74.
APA Style Citation: V. Sughanthini, P. Bharathisindhu, (2024). A Survey of DDoS Attack Detection Schemes: Methods, Challenges, and Datasets. International Journal of Computer Sciences and Engineering, 12(4), 68-74.
BibTex Style Citation:
@article{Sughanthini_2024,
author = {V. Sughanthini, P. Bharathisindhu},
title = {A Survey of DDoS Attack Detection Schemes: Methods, Challenges, and Datasets},
journal = {International Journal of Computer Sciences and Engineering},
issue_date = {4 2024},
volume = {12},
Issue = {4},
month = {4},
year = {2024},
issn = {2347-2693},
pages = {68-74},
url = {https://www.ijcseonline.org/full_paper_view.php?paper_id=5681},
doi = {https://doi.org/10.26438/ijcse/v12i4.6874}
publisher = {IJCSE, Indore, INDIA},
}
RIS Style Citation:
TY - JOUR
DO = {https://doi.org/10.26438/ijcse/v12i4.6874}
UR - https://www.ijcseonline.org/full_paper_view.php?paper_id=5681
TI - A Survey of DDoS Attack Detection Schemes: Methods, Challenges, and Datasets
T2 - International Journal of Computer Sciences and Engineering
AU - V. Sughanthini, P. Bharathisindhu
PY - 2024
DA - 2024/04/30
PB - IJCSE, Indore, INDIA
SP - 68-74
IS - 4
VL - 12
SN - 2347-2693
ER -
![]() |
![]() |
![]() |
17 | 28 downloads | 10 downloads |
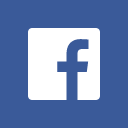
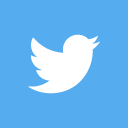
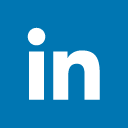
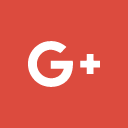
Abstract
Cloud computing makes use of a significant amount of virtual storage to provide services on demand via the Internet. The main benefits of cloud computing are reduced service costs and the elimination of the need for consumers to set up expensive computer hardware. The rapid integration of cloud computing with business and numerous other domains has prompted scholars to investigate novel, related technologies. Because of the cloud storage server`s scale and accessibility, individual businesses and users bring their apps, data, and facilities to it for computing operations. Despite the advantages, switching from local to remote computing has created several challenges and security issues for service providers as well as clients. The cloud service provider uses several web technologies to supply its services via the Internet, raising fresh security concerns. The DDoS assault, which aims to prevent legitimate users from accessing a target system or network by overloading it with traffic, is the most serious security issue in cloud computing. In light of this, the article covers the principles of cloud computing, as well as its various forms, security concerns, DDoS assaults, and methods for detecting them using performance metrics and datasets. Lastly, a discussion of cloud computing`s difficulties is included.
Key-Words / Index Term
Cloud Computing, Distributed Denial of Services (DDoS), DDoS attack Detection; Machine learning; Deep learning
References
[1] M. Masdari and M. Jalali, "A survey and taxonomy of DoS attacks in cloud computing," Security and Communication Networks, Vol.9, No.16, pp.3724-3751, 2016.
[2] K. Lee, J. Kim, K. H. Kwon, Y. Han, and S. Kim, "DDoS attack detection method using cluster analysis," Expert systems with applications, Vol.34, No.3, pp.1659-1665, 2008.
[3] N. Aslam, S. Srivastava, and M. Gore, "A comprehensive analysis of machine learning-and deep learning-based solutions for DDoS attack detection in SDN," Arabian Journal for Science and Engineering, Vol.49, No.3, pp.3533-3573, 2024.
[4] N. Agrawal and S. Tapaswi, "Defense schemes for variants of distributed denial-of-service (DDoS) attacks in cloud computing: A survey," Information Security Journal: A Global Perspective, Vol.26, No.2, pp.61-73, 2017.
[5] O. Osanaiye, K.-K. R. Choo, and M. Dlodlo, "Distributed denial of service (DDoS) resilience in cloud: Review and conceptual cloud DDoS mitigation framework," Journal of Network and Computer Applications, Vol.67, pp.147-165, 2016.
[6] A. Rawashdeh, M. Alkasassbeh, and M. Al-Hawawreh, "An anomaly-based approach for DDoS attack detection in cloud environment," International Journal of Computer Applications in Technology, vol. 57, no. 4, pp. 312-324, 2018.
[7] N. Agrawal and S. Tapaswi, "Defense Mechanisms Against DDoS Attacks in a Cloud Computing Environment: State-of-the-Art and Research Challenges," IEEE Communications Surveys & Tutorials, vol.21, no.4, pp.3769-3795, 2019, doi: 10.1109/COMST.2019.2934468.
[8] R. Kavitha, K. Saravanan, S. A. Jebakumari, and K. Velusamy, "Machine learning algorithms for IoT applications," Artificial Intelligence for Internet of Things: Design Principle, Modernization, and Techniques, p. 185, 2022.
[9] H. Polat, O. Polat, and A. Cetin, "Detecting DDoS Attacks in Software-Defined Networks Through Feature Selection Methods and Machine Learning Models," Sustainability, vol. 12, no. 3, p. 1035, 2020.
[10] M. Idhammad, K. Afdel, and M. Belouch, "Detection system of HTTP DDoS attacks in a cloud environment based on information theoretic entropy and random forest," Security and Communication Networks, vol. 2018, 2018.
[11] A. Saied, R. E. Overill, and T. Radzik, "Detection of known and unknown DDoS attacks using Artificial Neural Networks," Neurocomputing, vol. 172, pp. 385-393, 2016.
[12] A. Amjad, T. Alyas, U. Farooq, and M. Tariq, "Detection and mitigation of DDoS attack in cloud computing using machine learning algorithm," EAI Endorsed Transactions on Scalable Information Systems, vol. 6, no. 23, 2019.
[13] M. Ghanbari and W. Kinsner, "Detecting DDoS Attacks Using Polyscale Analysis and Deep Learning," International Journal of Cognitive Informatics and Natural Intelligence (IJCINI), vol. 14, no. 1, pp. 17-34, 2020.
[14] A. Naithani, S. N. Singh, K. K. Singh, and S. Kumar, "Machine Learning for Cloud-Based DDoS Attack Detection: A Comprehensive Algorithmic Evaluation," in 2024 14th International Conference on Cloud Computing, Data Science & Engineering (Confluence), 2024: IEEE, pp. 561-567.
[15] O. A. Osanaiye and M. Dlodlo, "TCP/IP header classification for detecting spoofed DDoS attack in Cloud environment," in IEEE EUROCON 2015-International Conference on Computer as a Tool (EUROCON),: IEEE, pp. 1-6, 2015.
[16] G. Somani, M. S. Gaur, D. Sanghi, M. Conti, and R. Buyya, "DDoS attacks in cloud computing: Issues, taxonomy, and future directions," Computer Communications, vol. 107, pp. 30-48, 2017.
[17] B. B. Gupta and O. P. Badve, "Taxonomy of DoS and DDoS attacks and desirable defense mechanism in a cloud computing environment," Neural Computing and Applications, vol. 28, pp. 3655-3682, 2017.
[18] S. Velliangiri, P. Karthikeyan, and V. Vinoth Kumar, "Detection of distributed denial of service attack in cloud computing using the optimization-based deep networks," Journal of Experimental & Theoretical Artificial Intelligence, vol. 33, no. 3, pp. 405-424, 2021.
[19] G. S. Kushwah and V. Ranga, "Detecting DDoS Attacks in Cloud Computing Using Extreme Learning Machine and Adaptive Differential Evolution," Wireless Personal Communications, pp. 1-24, 2022.
[20] M. G. Alam, S. J. N. Kumar, R. U. Mageswari, and T. M. Raj, "An Efficient SVM Based DEHO Classifier to Detect DDoS Attack in Cloud Computing Environment," Computer Networks, p. 109138, 2022.
[21] A. Rezaeipanah, S. E. Mousavipoor, M. Asayeshjoo, and M. Sadeghzadeh, "Combining Particle Swarm Optimization and Entropy to Detect DDoS Attacks in the Cloud Computing," Journal of Business Data Science Research, vol. 1, no. 1, pp. 33-43, 2021.
[22] A. Agarwal, M. Khari, and R. Singh, "Detection of DDOS attack using deep learning model in cloud storage application," Wireless Personal Communications, pp. 1-21, 2021.
[23] P. S. Muhuri, P. Chatterjee, X. Yuan, K. Roy, and A. Esterline, "Using a long short-term memory recurrent neural network (LSTM-RNN) to classify network attacks," Information, vol. 11, no. 5, pp. 243, 2020.
[24] M. Wang, Y. Lu, and J. Qin, "A dynamic MLP-based DDoS attack detection method using feature selection and feedback," Computers & Security, vol. 88, p. 101645, 2020.
[25] N. Ahuja, G. Singal, D. Mukhopadhyay, and N. Kumar, "Automated DDOS attack detection in software defined networking," Journal of Network and Computer Applications, vol.187, pp.103108, 2021.
[26] M. Arunadevi and V. Sathya, "DDoS Attack Detection using Optimized Back Propagation Neural Network with Artificial Plant Optimization in Cloud Computing," in 2022 3rd International Conference on Smart Electronics and Communication (ICOSEC),: IEEE, pp.815-820, 2022.
[27] G. S. Kushwah and S. T. Ali, "Distributed denial of service attacks detection in cloud computing using extreme learning machine," International Journal of Communication Networks and Distributed Systems, vol.23, no.3, pp. 328-351, 2019.
[28] G. S. Kushwah and V. Ranga, "Voting extreme learning machine based distributed denial of service attack detection in cloud computing," Journal of Information Security and Applications, vol. 53, p. 102532, 2020.
[29] G. S. Kushwah and V. Ranga, "Optimized extreme learning machine for detecting DDoS attacks in cloud computing," Computers & Security, vol. 105, pp.102260, 2021.
[30] G. S. Kushwah and V. Ranga, "Detecting DDoS attacks in cloud computing using extreme learning machine and adaptive differential evolution," Wireless Personal Communications, vol. 124, no. 3, pp. 2613-2636, 2022.
[31] G. S. Kushwah, S. Singh, and S. K. Mahana, "One-Class ELM Ensemble-Based DDoS Attack Detection in Multimedia Cloud Computing," in Examining Multimedia Forensics and Content Integrity: IGI Global, 2023, pp. 38-55.
[32] A. Fatani, M. Abd Elaziz, A. Dahou, M. A. Al-Qaness, and S. Lu, "IoT intrusion detection system using deep learning and enhanced transient search optimization," IEEE Access, vol.9, pp.123448-123464, 2021.
[33] Y. Sanjalawe and T. Althobaiti, "DDoS Attack Detection in Cloud Computing Based on Ensemble Feature Selection and Deep Learning," Computers, Materials & Continua, vol. 75, no. 2, 2023.
[34] A. Thangasamy, B. Sundan, and L. Govindaraj, "A Novel Framework for DDoS Attacks Detection Using Hybrid LSTM Techniques," Computer Systems Science & Engineering, vol. 45, no. 3, 2023.
[35] U. Islam, A. Al-Atawi, H. S. Alwageed, M. Ahsan, F. A. Awwad, and M. R. Abonazel, "Real-Time Detection schemes for Memory DoS (M-DoS) Attacks on Cloud Computing Applications," IEEE Access, 2023.
[36] S. Balasubramaniam et al., "Optimization Enabled Deep Learning-Based DDoS Attack Detection in Cloud Computing," International Journal of Intelligent Systems, vol. 2023, 2023.
[37] M. Malik and M. Dutta, "Feature engineering and machine learning framework for DDoS attack detection in the standardized internet of things," IEEE Internet of Things Journal, 2023.