Impact of Near Real Time Data on Data Science Model Predictions
Ankush Ramprakash Gautam1 , Ritu Sharma2
- Senior Manager Engineering, Datastax, Frisco, Texas, USA.
- Lead Data Scientist, JPMorgan Chase & Co, Plano, Texas, USA.
Section:Research Paper, Product Type: Journal Paper
Volume-12 ,
Issue-4 , Page no. 55-60, Apr-2024
CrossRef-DOI: https://doi.org/10.26438/ijcse/v12i4.5560
Online published on Apr 30, 2024
Copyright © Ankush Ramprakash Gautam, Ritu Sharma . This is an open access article distributed under the Creative Commons Attribution License, which permits unrestricted use, distribution, and reproduction in any medium, provided the original work is properly cited.
View this paper at Google Scholar | DPI Digital Library
How to Cite this Paper
- IEEE Citation
- MLA Citation
- APA Citation
- BibTex Citation
- RIS Citation
IEEE Style Citation: Ankush Ramprakash Gautam, Ritu Sharma, “Impact of Near Real Time Data on Data Science Model Predictions,” International Journal of Computer Sciences and Engineering, Vol.12, Issue.4, pp.55-60, 2024.
MLA Style Citation: Ankush Ramprakash Gautam, Ritu Sharma "Impact of Near Real Time Data on Data Science Model Predictions." International Journal of Computer Sciences and Engineering 12.4 (2024): 55-60.
APA Style Citation: Ankush Ramprakash Gautam, Ritu Sharma, (2024). Impact of Near Real Time Data on Data Science Model Predictions. International Journal of Computer Sciences and Engineering, 12(4), 55-60.
BibTex Style Citation:
@article{Gautam_2024,
author = {Ankush Ramprakash Gautam, Ritu Sharma},
title = {Impact of Near Real Time Data on Data Science Model Predictions},
journal = {International Journal of Computer Sciences and Engineering},
issue_date = {4 2024},
volume = {12},
Issue = {4},
month = {4},
year = {2024},
issn = {2347-2693},
pages = {55-60},
url = {https://www.ijcseonline.org/full_paper_view.php?paper_id=5679},
doi = {https://doi.org/10.26438/ijcse/v12i4.5560}
publisher = {IJCSE, Indore, INDIA},
}
RIS Style Citation:
TY - JOUR
DO = {https://doi.org/10.26438/ijcse/v12i4.5560}
UR - https://www.ijcseonline.org/full_paper_view.php?paper_id=5679
TI - Impact of Near Real Time Data on Data Science Model Predictions
T2 - International Journal of Computer Sciences and Engineering
AU - Ankush Ramprakash Gautam, Ritu Sharma
PY - 2024
DA - 2024/04/30
PB - IJCSE, Indore, INDIA
SP - 55-60
IS - 4
VL - 12
SN - 2347-2693
ER -
![]() |
![]() |
![]() |
17 | 35 downloads | 14 downloads |
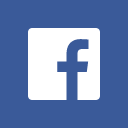
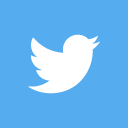
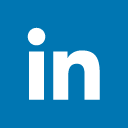
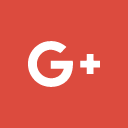
Abstract
The article delves into an exploration of how the integration of almost real time data streams impacts the accuracy, strength and effectiveness of models, in the ever changing field of data science. groups go beyond boundaries to examine sectors carefully analyzing the effects of data velocity on model performance in industries like finance, healthcare and transportation. Through an investigation the article reveals a story that highlights not the many benefits but also examines the complex challenges involved in utilizing almost real time data for modeling purposes. Additionally the article takes a look at the details discussing the necessary setup requirements and explaining the various methodological approaches needed to seamlessly integrate rapidly updating data streams into existing modeling frameworks. The paper also covers considerations and privacy requirements, for handling data responsibly emphasizing the importance of preserving individual privacy and data integrity. In the end this research acts as a signal emphasizing the importance of utilizing nearly real time data to enhance predictive abilities and drive a significant change in how decisions are made in various fields. This pushes us towards a future of opportunities and transformative possibilities.
Key-Words / Index Term
Data Science, Data Quality, Real Time
References
[1] The Art of Data Science & Big Data Analytics Inspecting & transforming data , Akella Subhadra , Survey Paper | Journal Paper, Jun, Vol.8, Issue.6, pp.91-100, 2020, CrossRef-DOI: , https://doi.org/10.26438/ijcse/v8i6.91100
[2] LONGBING, University of Technology Sydney, Australia, Data Science: A Comprehensive Overview, ACM Computing. Vol.50, Issue.3, pp.1–42, 2017.
[3] Dmitry Ivanov, Omer Ben-Porat, Real-Time Customer Journey Personalization using Online Machine Learning. Published: August 2021.
[4] Pankaj Gupta, "Leveraging Machine Learning and Artificial Intelligence for Fraud Prevention," SSRG International Journal of Computer Science and Engineering, Vol.10, No.5, pp.47-52, 2023. Crossref, https://doi.org/10.14445/23488387/IJCSE V10I5P107
[5] Rahnuma Mahzabin, Fahim Hossain Sifat, Sadia Anjum, Al-Akhir Nayan, Muhammad Golam Kibria, Blockchain associated machine learning and IoT based hypoglycemia detection system with auto-injection feature, Vol.27, No.1, pp.447-455, 2022. Cross Ref-DOI https://doi.org/10.48550/arXiv.2208.02222
[6] Niklas Christoffer Petersen a,b,*, Filipe Rodrigues b, Francisco Camara Pereira b, Multi-output Deep Learning for Bus Arrival Time Predictions, Cross Ref-DOI, Vol.41, pp.138-145, 2019. https://doi.org/10.1016/j.trpro.2019.09.025
[7] Mohamed Medhat Gaber, Arkady Zaslavsky and Shonali Krishnaswamy, Mining Data Streams: A Review , ACM SIGMOD Record, Vol.34, Issue.2, pp.18–26, 2005. https://doi.org/10.1145/1083784.1083789
[8] Xinjun Qi, Mingkui Zong, An Overview of Privacy Preserving Data Mining, Data Privacy challenges, Vol.12, pp.1341-1347, 2012. https://doi.org/10.1016/j.proenv.2012.01.432
[9] J.D. Kelleher, B.M. Namee, A. D’Arcy, “Fundamentals of Machine Learning for Predictive Data Analytics: Algorithms, Worked Examples, and Case Studies” The MIT Press, 2015.
[10] Damiano Perri,Marco Simonetti,Osvaldo Gervasi , Deploying Efficiently Modern Applications on Cloud, Electronics, Vol.11, Issue.3, pp.450, 2022. https://doi.org/10.3390/electronics11030450