A Systematic Analysis of Two-Dimensional Face Recognition Methods
Arpit Agrawal1
Section:Research Paper, Product Type: Journal Paper
Volume-5 ,
Issue-11 , Page no. 242-249, Nov-2017
CrossRef-DOI: https://doi.org/10.26438/ijcse/v5i11.242249
Online published on Nov 30, 2017
Copyright © Arpit Agrawal . This is an open access article distributed under the Creative Commons Attribution License, which permits unrestricted use, distribution, and reproduction in any medium, provided the original work is properly cited.
View this paper at Google Scholar | DPI Digital Library
How to Cite this Paper
- IEEE Citation
- MLA Citation
- APA Citation
- BibTex Citation
- RIS Citation
IEEE Style Citation: Arpit Agrawal, “A Systematic Analysis of Two-Dimensional Face Recognition Methods,” International Journal of Computer Sciences and Engineering, Vol.5, Issue.11, pp.242-249, 2017.
MLA Style Citation: Arpit Agrawal "A Systematic Analysis of Two-Dimensional Face Recognition Methods." International Journal of Computer Sciences and Engineering 5.11 (2017): 242-249.
APA Style Citation: Arpit Agrawal, (2017). A Systematic Analysis of Two-Dimensional Face Recognition Methods. International Journal of Computer Sciences and Engineering, 5(11), 242-249.
BibTex Style Citation:
@article{Agrawal_2017,
author = {Arpit Agrawal},
title = {A Systematic Analysis of Two-Dimensional Face Recognition Methods},
journal = {International Journal of Computer Sciences and Engineering},
issue_date = {11 2017},
volume = {5},
Issue = {11},
month = {11},
year = {2017},
issn = {2347-2693},
pages = {242-249},
url = {https://www.ijcseonline.org/full_paper_view.php?paper_id=5584},
doi = {https://doi.org/10.26438/ijcse/v5i11.242249}
publisher = {IJCSE, Indore, INDIA},
}
RIS Style Citation:
TY - JOUR
DO = {https://doi.org/10.26438/ijcse/v5i11.242249}
UR - https://www.ijcseonline.org/full_paper_view.php?paper_id=5584
TI - A Systematic Analysis of Two-Dimensional Face Recognition Methods
T2 - International Journal of Computer Sciences and Engineering
AU - Arpit Agrawal
PY - 2017
DA - 2017/11/30
PB - IJCSE, Indore, INDIA
SP - 242-249
IS - 11
VL - 5
SN - 2347-2693
ER -
![]() |
![]() |
![]() |
66 | 1534 downloads | 62 downloads |
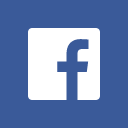
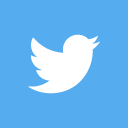
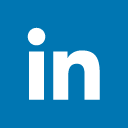
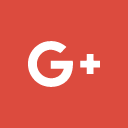
Abstract
Face recognition is the most effective and extensively used biometric method. It`s cheap, natural, and non-intrusive. Researchers have created hundreds of facial recognition methods in recent years. Based on face data processing, these methods fall into three groups. The suggested recognition system may employ the full face, particular features or parts of the face, or both global and local face characteristics. This article reviews well-known approaches in each category. First, we discuss biometric facial recognition`s pros and cons. We then describe each well-known method`s concept. Next, the three facial recognition methods are compared. These algorithms are applied to face recognition databases, and some results are shown. Finally, we discuss intriguing new research areas.
Key-Words / Index Term
biometrics; face recognition; person identification
References
[1]. Aoun, N.B. et. al., “Graph-based approach for human action recognition using spatio-temporal features,” J. Vis. Commun. Image Represent, Vol.25, pp.329-338, 2014.
[1]. El’Arbi, et. al., “Video watermarking based on neural networks,” In Proceedings of the 2006 IEEE International Conference on Multimedia and Expo, Toronto, ON, Canada, pp. 1577-1580, 2006.
[2]. El’Arbi, et. al., “A dynamic video watermarking algorithm in fast motion areas in the wavelet domain,” Multimed. Tools Application, Vol. 55, pp.579-600, 2011.
[3]. Wali, A.; et. al., “A new system for event detection from video surveillance sequences,” In Advanced Concepts for Intelligent Vision Systems, Proceedings of the 12th International Conference, ACIVS 2010, Sydney, Australia, 13-16 December 2010;
[4]. Blanc-Talon, et. al., “Lecture Notes in Computer Science. Springer: Berlin/Heidelberg, Germany, Vol.6475, pp. 110-120, 2010.
[5]. Koubaa, M. et. al., “MPEG4 compression and frame dropping resistant video watermarking,” Multimed. Tools Application, Vol.56, pp.281-301, 2012.
[6]. Mejdoub et. al., “Classification improvement of local feature vectors over the KNN algorithm,” Multimed. Tools Application, Vol.64, pp.197-218, 2013.
[7]. Dammak, M. et. al., “Feature vector approximation based on wavelet network,” In Proceedings of the 4th International Conference on Agents and Artificial Intelligence, Vilamoura, Portugal, pp. 394-399, 2009.
[8]. Borgi, M.A. et. al., “Shearlet network-based sparse coding augmented by facial texture features for face recognition,” In Image Analysis and Processing—ICIAP 2013, Proceedings of the 17th International Conference, Naples, Italy, 9-13 September 2013.
[9]. Huang, D. et. al., “3D face analysis: Advances and perspectives,” In Proceedings of the Chinese Conference on Biometric Recognition, Shenyang, China, 7–9 November 2014.
[10]. Drira, H. et. al., “ 3D face recognition under expressions, occlusions, and pose variations,” IEEE Trans. Pattern Anal. Mach. Intell. Vol.35, pp.2270–2283, 2013.
[11]. Huang, D. et. al., “3-D face recognition using eLBP-based facial description and local feature hybrid matching,” IEEE Trans. Inf. Forensics Secur. Vol.7, pp.1551–1565, 2012.
[12]. Said, S. et. al., “3D face recognition using wavelet networks,” In Proceedings of the 2009 16th IEEE International Conference on Image Processing (ICIP), Cairo, Egypt, pp. 4153–4156, 2009.
[13]. Borgi, M.A. et. al., “Wavelet network and geometric features fusion using belief functions for 3D face recognition,” In Computer Analysis of Images and Patterns, Proceedings of the 15th International Conference, CAIP 2013,
[14]. Soltana, W.B. et. al., “Multi library wavelet neural networks for 3D face recognition using 3D facial shape representation,” In Proceedings of the 2009 17th European Signal Processing Conference, Glasgow, Scotland, pp. 55–59 2009.
[15]. Bowyer et. al., “A survey of approaches and challenges in 3D and multimodal 3D+2D face recognition,” Comput. Vis. Image Understand, Vol.101, pp1–15, 2006.
[16]. Lakshmiprabha, N.S. et. al., “Face recognition using multimodal biometric features,” In Proceedings of the International Conference on Image Information Processing (ICIIP), Shimla, India, 3–5 November 2011; pp. 1–6, 2011.
[17]. Radhey, S. et. al., “Identifying individuals using multimodal face recognition techniques,” Procedia Computer Science, Vol.48, pp.666–672, 2015.
[18]. Stephen, B. Deep learning and face recognition: The state of the art,” Proc. SPIE, 9457, 2015.
[19]. Li, S.Z. et. al., “Illumination invariant face recognition using near-infrared images,” IEEE Trans. Pattern Anal. Mach. Intell. Vol.29, pp.627–639, 2007.
[20]. Huang, D. et. al., “robust method for near infrared face recognition based on extended local binary pattern,” In Proceedings of the International Symposium on Visual Computing, Lake Tahoe, NV, USA, 26–28 November 2007.
[21]. Friedrich, G. et. al., “Seeing people in the dark: Face recognition in infrared images. In Biologically Motivated Computer Vision; Springer: Berlin/Heidelberg, Germany, 2003.
[22]. Jeni, L.A. et. al., “Robust facial expression recognition using near infrared cameras,” J. Adv. Comput. Intell. Intell. Inform., Vol.16, pp.341–348, 2018.
[23]. Wang, R. et. al., “Multimodal biometrics based on near-infrared face,” In Biometrics: Theory, Methods, and Applications; Wiley-IEEE Press: Hoboken, NJ, USA, Vol.9, 2009.
[24]. Ngiam, J. et. al., “Multimodal deep learning,” In Proceedings of the 28th International Conference on Machine Learning ICML-11, Bellevue, WA, USA, pp. 689–696, 2011.