Deep Learning Approach Towards T-Rex Game
Abujar Shaikh1 , A.K. Sampath2 , Anupam Choudhary3
Section:Research Paper, Product Type: Journal Paper
Volume-8 ,
Issue-9 , Page no. 24-27, Sep-2020
CrossRef-DOI: https://doi.org/10.26438/ijcse/v8i9.2427
Online published on Sep 30, 2020
Copyright © Abujar Shaikh, A.K. Sampath, Anupam Choudhary . This is an open access article distributed under the Creative Commons Attribution License, which permits unrestricted use, distribution, and reproduction in any medium, provided the original work is properly cited.
View this paper at Google Scholar | DPI Digital Library
How to Cite this Paper
- IEEE Citation
- MLA Citation
- APA Citation
- BibTex Citation
- RIS Citation
IEEE Style Citation: Abujar Shaikh, A.K. Sampath, Anupam Choudhary, “Deep Learning Approach Towards T-Rex Game,” International Journal of Computer Sciences and Engineering, Vol.8, Issue.9, pp.24-27, 2020.
MLA Style Citation: Abujar Shaikh, A.K. Sampath, Anupam Choudhary "Deep Learning Approach Towards T-Rex Game." International Journal of Computer Sciences and Engineering 8.9 (2020): 24-27.
APA Style Citation: Abujar Shaikh, A.K. Sampath, Anupam Choudhary, (2020). Deep Learning Approach Towards T-Rex Game. International Journal of Computer Sciences and Engineering, 8(9), 24-27.
BibTex Style Citation:
@article{Shaikh_2020,
author = {Abujar Shaikh, A.K. Sampath, Anupam Choudhary},
title = {Deep Learning Approach Towards T-Rex Game},
journal = {International Journal of Computer Sciences and Engineering},
issue_date = {9 2020},
volume = {8},
Issue = {9},
month = {9},
year = {2020},
issn = {2347-2693},
pages = {24-27},
url = {https://www.ijcseonline.org/full_paper_view.php?paper_id=5206},
doi = {https://doi.org/10.26438/ijcse/v8i9.2427}
publisher = {IJCSE, Indore, INDIA},
}
RIS Style Citation:
TY - JOUR
DO = {https://doi.org/10.26438/ijcse/v8i9.2427}
UR - https://www.ijcseonline.org/full_paper_view.php?paper_id=5206
TI - Deep Learning Approach Towards T-Rex Game
T2 - International Journal of Computer Sciences and Engineering
AU - Abujar Shaikh, A.K. Sampath, Anupam Choudhary
PY - 2020
DA - 2020/09/30
PB - IJCSE, Indore, INDIA
SP - 24-27
IS - 9
VL - 8
SN - 2347-2693
ER -
![]() |
![]() |
![]() |
736 | 589 downloads | 121 downloads |
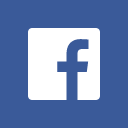
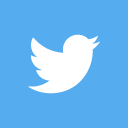
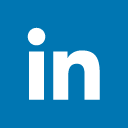
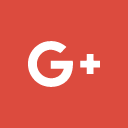
Abstract
In this project, we enforce both feature-extraction based totally algorithms and an end-to-cease deep reinforcement mastering technique to discover ways to control Chrome offline dinosaur recreation directly from high-dimensional sport display input. Results display that as compared with the pixel function based totally algorithms, deep reinforcement learning is more effective and effective. It leverages the high-dimensional sensory input immediately and avoids capability errors in characteristic extraction. Finally, we recommend special schooling strategies to address class imbalance issues due to the boom in game velocity. A simple and smooth GUI is supplied for smooth gameplay. The gameplay layout is so simple that user won’t discover it tough to use and understand. Different images are used within the development of this easy recreation project, the gaming environment is similar to the authentic T-Rex Dino Run sport. In order to run the project, you need to have set up python and pygame in your PC. This might be a new word for many however each and every one of us has learned to stroll the usage of the idea of Reinforcement Learning (RL) and this is how our brain still works. A reward gadget is a foundation for any RL algorithm.
Key-Words / Index Term
Deep reinforcement, Sensory input
References
[1]https://thenextweb.com/dd/2018/09/07/4-years-later-google finally-explains-the-origins-ofits-chrome-dinosaur-game/
[2]V. Mnih, K. Kavukcuoglu, D. Silver, A. Graves, IAntonoglou, D. Riedmiller. Playing Atari with deep reinforcement learning. arXiv preprint arXiv:1312.5602, 2013.
[3] V. Mnih, K. Kavukcuoglu, D. Silver, A. A. Rusu, J. Veness, M. G. Bellemare, A. Graves, M.Riedmiller, A. K. Fidjeland, G. Ostrovski, et al. Human-level control through deep reinforcementlearning. Nature, 518(7540):529–533, 2015.
[4] G. Tesauro. Temporal difference learning and td-gammon. Communications of the ACM,38(3):58–68, 1995.
[5] G. Bradski et al. The opencv library. Doctor Dobb Journal, 25(11):120–126, 2000.
[6] Koray Kayabol, Member, IEEE ”Histogram-Based Contextual Classification of SAR Images”, IEEE geoscience and remote sensing letters, vol. 12, no. 1, January 2015.
[7] Xin Su, Chu He, Member, IEEE, Qian Feng, Xinping Deng, and Hong Sun, Member, IEEE "A Supervised Classification Method Based on Conditional Random Fields With Multiscale Region Connection Calculus Model for SAR Image",IEEE geoscience and remote sensing letters, vol. 8, no. 3, May 2011.
[8] Mery Picco and Gabriela Palacio "Unsupervised Classification of SAR Images Using Markov Random Fields and G0 I Model",IEEE geoscience and remote sensing letters, vol. 8, no. 2, March 2011.
[9] A. Sharma, P.K. Misra, “Aspects of enhancing security in software development life cycle,” Advances in Computational Sciences and Technology, Vol. 10, Issue. 2, pp. 203-210.
[10] Jr. Padal, C.M. Salado, L. Mary Jean May, N.P. Sobejana, “SPAMAST Smart Garbage Bin Monitoring System Using Wireless Sensor Network,” Journal of Engineering Research and Reports, pp. 1-16, 2019.
[11] D. Goel, R. Upadhyay, “Effectiveness of use of Animation in Advertising: A Literature Review,” International Journal of Scientific Research in Network Security and Communication, Vol.5, Issue. 3, pp. 146-159, 2017.