Predicting Autism Spectrum Disorder Using Machine Learning Algorithms: A Review
Kalpana C.1 , Anitha Kumari K.2
Section:Review Paper, Product Type: Journal Paper
Volume-8 ,
Issue-5 , Page no. 31-36, May-2020
CrossRef-DOI: https://doi.org/10.26438/ijcse/v8i5.3136
Online published on May 30, 2020
Copyright © Kalpana C., Anitha Kumari K. . This is an open access article distributed under the Creative Commons Attribution License, which permits unrestricted use, distribution, and reproduction in any medium, provided the original work is properly cited.
View this paper at Google Scholar | DPI Digital Library
How to Cite this Paper
- IEEE Citation
- MLA Citation
- APA Citation
- BibTex Citation
- RIS Citation
IEEE Style Citation: Kalpana C., Anitha Kumari K., “Predicting Autism Spectrum Disorder Using Machine Learning Algorithms: A Review,” International Journal of Computer Sciences and Engineering, Vol.8, Issue.5, pp.31-36, 2020.
MLA Style Citation: Kalpana C., Anitha Kumari K. "Predicting Autism Spectrum Disorder Using Machine Learning Algorithms: A Review." International Journal of Computer Sciences and Engineering 8.5 (2020): 31-36.
APA Style Citation: Kalpana C., Anitha Kumari K., (2020). Predicting Autism Spectrum Disorder Using Machine Learning Algorithms: A Review. International Journal of Computer Sciences and Engineering, 8(5), 31-36.
BibTex Style Citation:
@article{C._2020,
author = {Kalpana C., Anitha Kumari K.},
title = {Predicting Autism Spectrum Disorder Using Machine Learning Algorithms: A Review},
journal = {International Journal of Computer Sciences and Engineering},
issue_date = {5 2020},
volume = {8},
Issue = {5},
month = {5},
year = {2020},
issn = {2347-2693},
pages = {31-36},
url = {https://www.ijcseonline.org/full_paper_view.php?paper_id=5105},
doi = {https://doi.org/10.26438/ijcse/v8i5.3136}
publisher = {IJCSE, Indore, INDIA},
}
RIS Style Citation:
TY - JOUR
DO = {https://doi.org/10.26438/ijcse/v8i5.3136}
UR - https://www.ijcseonline.org/full_paper_view.php?paper_id=5105
TI - Predicting Autism Spectrum Disorder Using Machine Learning Algorithms: A Review
T2 - International Journal of Computer Sciences and Engineering
AU - Kalpana C., Anitha Kumari K.
PY - 2020
DA - 2020/05/30
PB - IJCSE, Indore, INDIA
SP - 31-36
IS - 5
VL - 8
SN - 2347-2693
ER -
![]() |
![]() |
![]() |
344 | 479 downloads | 140 downloads |
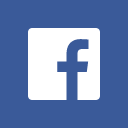
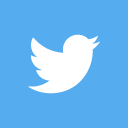
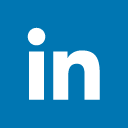
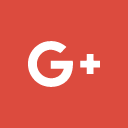
Abstract
Among many psychiatric disorders, Autism Spectrum Disorder (ASD) affects people in diverse ways. Nowadays the prevalence of Autism spectrum disorder has increased gradually worldwide. Difficulty in social interaction, trouble with speech and nonverbal communication, repetitive actions, avoidance of eye contact and abnormal facial expressions are the primary symptoms of ASD. Predicting ASD at an early stage is important to provide necessary developmental support. Machine Learning algorithms play a vital role in prediction of ASD. In this study, Machine Learning algorithms like Linear Discriminant Analysis (LDA), K-Nearest Neighbor (KNN), Decision trees, Random forest –CART and merging of Random Forest-CART and Random forest - Iterative Dichotomiser 3 (ID3) are compared for ASD prediction.
Key-Words / Index Term
Autism Spectrum Disorder, Autistic Spectrum Disorders in Children and Adults, Machine Learning, Linear Discriminant Analysis, K-Nearest Neighbour, SVM, K-NN, Decision Trees, Random Forest CART & ID3
References
[1] Louridas P and Ebert C., “Machine Learning,” IEEE Softw., vol. 33, no. 5, pp. 110–115, 2016.
[2] Jordan, M. I., & Mitchell, T. M. “Machine learning: Trends, perspectives, and prospects”. Science, 349(6245), 255–260. doi:10.1126/science.aaa8415, 2015.
[3] Lichman, M “UCI Machine Learning Repository Irvine”, CA: University of California, School of Information and Computer Science, 2013.
[4] Stevens, E., Atchison, A., Stevens, L., Hong, E., Granpeesheh, D., Dixon, D., & Linstead, E., “A Cluster Analysis of Challenging Behaviors in Autism Spectrum Disorder”. 2017 16th IEEE International Conference on Machine Learning and Applications (ICMLA). doi:10.1109/icmla.2017.00-85, 2017.
[5] Maenner MJ, Shaw KA, Baio J, et al., “Prevalence of Autism Spectrum Disorder Among Children Aged 8 Years — Autism and Developmental Disabilities Monitoring Network”, 11 Sites, United States, 2016. MMWR Surveill Summ 2020;69(No. SS-4):1–12. DOI: http://dx.doi.org/10.15585/mmwr.ss6904a1, 2016.
[6] Omar, K. S., Mondal, P., Khan, N. S., Rizvi, M. R. K., & Islam, M. N., “ A Machine Learning Approach to Predict Autism Spectrum Disorder”. 2019 International Conference on Electrical, Computer and Communication Engineering (ECCE). doi:10.1109/ecace.2019.8679454, 2019.
[7] Satu, M. S., Farida Sathi, F., Arifen, M. S., Hanif Ali, M., & Moni, M. A. “Early Detection of Autism by Extracting Features: A Case Study in Bangladesh”. 2019 International Conference on Robotics,Electrical and Signal Processing Techniques (ICREST). doi:10.1109/icrest.2019.8644357, 2019.
[8] Pal, R., Poray, J., & Sen, M, “Application of machine learning algorithms on diabetic retinopathy”. 2017 2nd IEEE International Conference on Recent Trends in Electronics, Information & Communication Technology (RTEICT). doi:10.1109/rteict.2017.8256959, 2017.
[9] Liu, W., Yu, X., Raj, B., Yi, L., Zou, X., & Li, M., “ Efficient autism spectrum disorder prediction with eye movement: A machine learning framework”. 2015 International Conference on Affective Computing and Intelligent Interaction (ACII). doi:10.1109/acii.2015.7344638, 2015.
[10] Ecker, C., Rocha-Rego, V., Johnston, P., Mourao-Miranda, J., Marquand, A., Daly, E. M. Murphy, D. G., “Investigating the predictive value of whole-brain structural MR scans in autism: A pattern classification approach”. NeuroImage, 49(1), 44–56.
[11] Altay, O., & Ulas, M., “Prediction of the autism spectrum disorder diagnosis with linear discriminant analysis classifier and K-nearest neighbor in children”. 2018 6th International Symposium on Digital Forensic and Security (ISDFS). doi:10.1109/isdfs.2018.8355354, 2018.
[12] Chorianopoulou, A., Tzinis, E., Iosif, E., Papoulidi, A., Papailiou, C., & Potamianos, A., “Engagement detection for children with Autism Spectrum Disorder”. 2017 IEEE International Conference on Acoustics, Speech and Signal Processing (ICASSP). doi:10.1109/icassp.2017.7953119, 2017.
[13] Mitsumoto, D., Hori, T., Sagayama, S., Yamasue, H., Owada, K., Kojima, MOno, N., “Autism Spectrum Disorder Discrimination Based on Voice Activities Related to Fillers and Laughter”. 2019 53rd Annual Conference on Information Sciences and Systems (CISS). doi:10.1109/ciss.2019.8692794,2019.
[14] Satu, M. S., Farida Sathi, F., Arifen, M. S., Hanif Ali, M., & Moni, M. A., “Early Detection of Autism by Extracting Features: A Case Study in Bangladesh”. 2019 International Conference on Robotics,Electrical and Signal Processing Techniques (ICREST). doi:10.1109/icrest.2019.8644357, 2019.
[15] Hyde, K. K., Novack, M. N., LaHaye, N., Parlett-Pelleriti, C., Anden, R., Dixon, D. R., & Linstead, E., “ Applications of Supervised Machine Learning in Autism Spectrum Disorder Research: a Review”. Review Journal of Autism and Developmental Disorders. doi:10.1007/s40489-019-00158-x, 2019
[16] Dutta, S. R., Datta, S., & Roy, M., “ Using Cogency and Machine Learning for Autism Detection from a Preliminary Symptom”. 2019 9th International Conference on Cloud Computing, Data Science & Engineering ,2019.
[17] Tyagi, B., Mishra, R., & Bajpai, N., “ Machine Learning Techniques to Predict Autism Spectrum Disorder”. 2018 IEEE Punecon. doi:10.1109/punecon.2018.8745405, 2018.
[18] Wall, D. P., Dally, R., Luyster, R., Jung, J.-Y., & DeLuca, T. F., “Use of Artificial Intelligence to Shorten the Behavioral Diagnosis of Autism”. PLoS ONE, 7(8), e43855. doi:10.1371/journal.pone.0043855, 2012.
[19] Allison, C., Auyeung, B., & Baron-Cohen, S. “ Toward Brief “Red Flags” for Autism Screening: The Short Autism Spectrum Quotient and the Short Quantitative Checklist in 1,000 Cases and 3,000 Controls”. Journal of the American Academy of Child & Adolescent Psychiatry, 51(2), 202–212.e7. doi:10.1016/j.jaac.2011.11.003, 2012.
[20] Sonar, P., & JayaMalini, K., “ Diabetes Prediction Using Different Machine Learning Approaches”. 2019 3rd International Conference on Computing Methodologies and Communication (ICCMC). doi:10.1109/iccmc.2019.8819841, 2019.
[21] Granpeesheh, Dennis Dixon and Erik Linstead.,” A cluster Analysis of challenging Behaviors in Autism Spectrum Disorder”. 2017 16th IEEE International Conference on Machine Learning and Applications (ICMLA), 2017.
[22] Omar, K. S., Mondal, P., Khan, N. S., Rizvi, M. R. K., & Islam, M. N.,“A Machine Learning Approach to Predict Autism Spectrum Disorder. 2019 International Conference on Electrical, Computer and Communication Engineering (ECCE). doi:10.1109/ecace.2019.8679454, 2019
[23] Heinsfeld, A. S., Franco, A. R., Craddock, R. C., Buchweitz, A., & Meneguzzi, F. “ Identification of autism spectrum disorder using deep learning and the ABIDE dataset. NeuroImage”: Clinical, 17, 16–23. doi:10.1016/j.nicl.2017.08.017, 2018.
[24] Duda, M., Kosmicki, J. A., & Wall, D. P., “ Testing the accuracy of an observation-based classifier for rapid detection of autism risk”. Translational Psychiatry, 4(8), e424–e424. doi:10.1038/tp.2014.65, 2014.
[25] Kosmicki, J. A., Sochat, V., Duda, M., & Wall, D. P. “Searching for a minimal set of behaviors for autism detection through feature selection-based machine learning”. Translational Psychiatry, 5(2), e514–e514. doi:10.1038/tp.2015.7, 2015.
[26] Jain, A., & Huang, J., “ Integrating independent components and linear discriminant analysis for gender classification”. Sixth IEEE International Conference on Automatic Face and Gesture Recognition, 2004. Proceedings. doi:10.1109/afgr.2004.1301524, 2004.
[27] Aparna Shenoy, K.V. Viswanatha, Raju Ramakrishna Gondkar, "Early Sepsis Prediction in Intensive Care Patients using Random Forest Classifier", International Journal of Computer Sciences and Engineering, Vol.8, Issue.1, pp.17-22, 2020.
[28] Rosy Mishra, Y. Sowjanya, Sushanta Meher, Mousumi Meher, "A Voice Signal Interpreter using Machine Learning Techniques," International Journal of Scientific Research in Network Security and Communication, Vol.8, Issue.2, pp.22-27, 2020.