Urban Built-up Change Detection with Minimum Redundancy Maximum Relevance Approach
Kyi Thar Oo1 , Khin Myo Kyi2 , Zarli Cho3
- University of Computer Studies, Thaton, Myanmar.
- University of Computer Studies, Taungoo, Myanmar.
- University of Computer Studies, Taungoo, Myanmar.
Section:Research Paper, Product Type: Journal Paper
Volume-8 ,
Issue-4 , Page no. 34-37, Apr-2020
CrossRef-DOI: https://doi.org/10.26438/ijcse/v8i4.3437
Online published on Apr 30, 2020
Copyright © Kyi Thar Oo, Khin Myo Kyi, Zarli Cho . This is an open access article distributed under the Creative Commons Attribution License, which permits unrestricted use, distribution, and reproduction in any medium, provided the original work is properly cited.
View this paper at Google Scholar | DPI Digital Library
How to Cite this Paper
- IEEE Citation
- MLA Citation
- APA Citation
- BibTex Citation
- RIS Citation
IEEE Style Citation: Kyi Thar Oo, Khin Myo Kyi, Zarli Cho, “Urban Built-up Change Detection with Minimum Redundancy Maximum Relevance Approach,” International Journal of Computer Sciences and Engineering, Vol.8, Issue.4, pp.34-37, 2020.
MLA Style Citation: Kyi Thar Oo, Khin Myo Kyi, Zarli Cho "Urban Built-up Change Detection with Minimum Redundancy Maximum Relevance Approach." International Journal of Computer Sciences and Engineering 8.4 (2020): 34-37.
APA Style Citation: Kyi Thar Oo, Khin Myo Kyi, Zarli Cho, (2020). Urban Built-up Change Detection with Minimum Redundancy Maximum Relevance Approach. International Journal of Computer Sciences and Engineering, 8(4), 34-37.
BibTex Style Citation:
@article{Oo_2020,
author = {Kyi Thar Oo, Khin Myo Kyi, Zarli Cho},
title = {Urban Built-up Change Detection with Minimum Redundancy Maximum Relevance Approach},
journal = {International Journal of Computer Sciences and Engineering},
issue_date = {4 2020},
volume = {8},
Issue = {4},
month = {4},
year = {2020},
issn = {2347-2693},
pages = {34-37},
url = {https://www.ijcseonline.org/full_paper_view.php?paper_id=5071},
doi = {https://doi.org/10.26438/ijcse/v8i4.3437}
publisher = {IJCSE, Indore, INDIA},
}
RIS Style Citation:
TY - JOUR
DO = {https://doi.org/10.26438/ijcse/v8i4.3437}
UR - https://www.ijcseonline.org/full_paper_view.php?paper_id=5071
TI - Urban Built-up Change Detection with Minimum Redundancy Maximum Relevance Approach
T2 - International Journal of Computer Sciences and Engineering
AU - Kyi Thar Oo, Khin Myo Kyi, Zarli Cho
PY - 2020
DA - 2020/04/30
PB - IJCSE, Indore, INDIA
SP - 34-37
IS - 4
VL - 8
SN - 2347-2693
ER -
![]() |
![]() |
![]() |
231 | 361 downloads | 157 downloads |
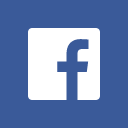
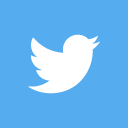
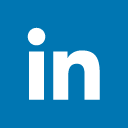
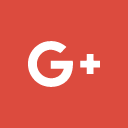
Abstract
Urban built-up area information is required in various applications of land use planning and management. Urban environment is made up with the complex interactions with built up environment and the human communities living within the urban area. The aim of the system is to assess an effective building change detection system that can identify gains and losses of built-up areas in relation to other land cover of Multi-temporal satellite image of Mandalay city in Myanmar. The proposed system apply to combine with gray level histogram features with minimum redundancy maximum relevance (MRMR) approach for built-up change detection system. The experimental analysis revealed that the proposed system combination with histogram features based on MRMR which is more reliable in urban built-up change detection system.
Key-Words / Index Term
MRMR, Hitogram feature,classification, detection
References
[1]Liow, Y.-T., and T. Pavlidis. 1990. “Use of Shadows for Extracting Buildings in Aerial Images.” Computer Vision, Graphics, and Image Processing 49: 242–277.
[2]Sirmacek, B., and C. Unsalan. 2009. “Urban-Area and Building Detection Using SIFT Keypoints and Graph Theory.” IEEE Transactions on Geoscience and Remote Sensing 47: 1156–1167.
[3] X. Huang and L. Zhang, “An SVM ensemble approach combining spectral, structural, and semantic features for the classification of high-resolution remotely sensed imagery,” IEEE Trans. Geosci. Remote Sens., vol. 51, no. 1, pp. 257–272, Jan. 2013.
[4] V. Walter, “Object-based classification of remote sensing data for change detection,” ISPRS J. Photogramm. Remote Sens., vol. 58, no. 3/4,pp. 225–238, Jan. 2004.
[5] T. Celik and K.-K. Ma, “Multitemporal image change detection using undecimated discrete wavelet transform and active contours,” IEEE Trans.Geosci. Remote Sens., vol. 49, no. 2, pp. 706–716, Feb. 2011.
[6] T. Celik, “Multiscale change detection in multitemporal satellite images,”IEEE Geosci. Remote Sens. Lett., vol. 6, no. 4, pp. 820–824, Oct. 2009.
[7] M. Dalla Mura, J. A. Benediktsson, F. Bovolo, and L. Bruzzone, “An unsupervised technique based on morphological filters for change detection in very high resolution images,” IEEE Geosci. Remote Sens. Lett., vol. 5,no. 3, pp. 433–437, Jul. 2008.
[8] M. Volpi, D. Tuia, F. Bovolo, M. Kanevski, and L. Bruzzone, “Supervised change detection in VHR images using contextual information and support vector machines,” Int. J. Appl. Earth Observ. Geoinf., vol. 20, pp. 77–85, Feb. 2013.
[9]Yawai Tint, Yoshiki Mikami, A Minimum Redundancy Maximum Relevance-based Causal Assessment of Injury Severity, International Journal of Computer Science and Information Security, Vol 15, No. 7, pp. 248-264, July 2017.
[10]Yawai Tint, Yoshiki Mikami, A Minimum Redundancy Maximum Relevance - based Approach for Multivariate Causality Analysis, International Journal of Advanced Computer Science and Applications, Vol 8, No.9, pp. 13-20, 2017.