A Novel Prediction of Diabetes by Automatic Insulin Therapy Using Machine Learning Algorithm
B. Vinothkumar1 , M. Ramaswami2
Section:Research Paper, Product Type: Journal Paper
Volume-8 ,
Issue-3 , Page no. 18-23, Mar-2020
Online published on Mar 30, 2020
Copyright © B. Vinothkumar, M. Ramaswami . This is an open access article distributed under the Creative Commons Attribution License, which permits unrestricted use, distribution, and reproduction in any medium, provided the original work is properly cited.
View this paper at Google Scholar | DPI Digital Library
How to Cite this Paper
- IEEE Citation
- MLA Citation
- APA Citation
- BibTex Citation
- RIS Citation
IEEE Style Citation: B. Vinothkumar, M. Ramaswami, “A Novel Prediction of Diabetes by Automatic Insulin Therapy Using Machine Learning Algorithm,” International Journal of Computer Sciences and Engineering, Vol.8, Issue.3, pp.18-23, 2020.
MLA Style Citation: B. Vinothkumar, M. Ramaswami "A Novel Prediction of Diabetes by Automatic Insulin Therapy Using Machine Learning Algorithm." International Journal of Computer Sciences and Engineering 8.3 (2020): 18-23.
APA Style Citation: B. Vinothkumar, M. Ramaswami, (2020). A Novel Prediction of Diabetes by Automatic Insulin Therapy Using Machine Learning Algorithm. International Journal of Computer Sciences and Engineering, 8(3), 18-23.
BibTex Style Citation:
@article{Vinothkumar_2020,
author = {B. Vinothkumar, M. Ramaswami},
title = {A Novel Prediction of Diabetes by Automatic Insulin Therapy Using Machine Learning Algorithm},
journal = {International Journal of Computer Sciences and Engineering},
issue_date = {3 2020},
volume = {8},
Issue = {3},
month = {3},
year = {2020},
issn = {2347-2693},
pages = {18-23},
url = {https://www.ijcseonline.org/full_paper_view.php?paper_id=5044},
publisher = {IJCSE, Indore, INDIA},
}
RIS Style Citation:
TY - JOUR
UR - https://www.ijcseonline.org/full_paper_view.php?paper_id=5044
TI - A Novel Prediction of Diabetes by Automatic Insulin Therapy Using Machine Learning Algorithm
T2 - International Journal of Computer Sciences and Engineering
AU - B. Vinothkumar, M. Ramaswami
PY - 2020
DA - 2020/03/30
PB - IJCSE, Indore, INDIA
SP - 18-23
IS - 3
VL - 8
SN - 2347-2693
ER -
![]() |
![]() |
![]() |
318 | 356 downloads | 148 downloads |
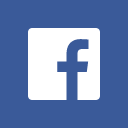
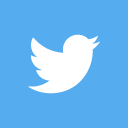
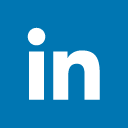
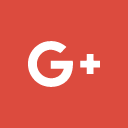
Abstract
Diabetes mellitus is one of the world’s fast-growing diseases. Differentiation is among the most important decision-making approaches in many real-world problems. In this work, the main objective is to classify the diabetic patient’s data into various levels based upon the values. This will help to assist the required dose which should be provided to the patients through an automatic insulin pump. The efficiency of the different classifiers is measured to assess the reliability of the classification. In this analysis, four common algorithms for machine learning, namely Support Vector Machine (SVM), Naive Bayes (NB), Logistic Regression, Random forest, and decision tree, for the estimation of diabetic mellitus on data from the adult population. Based on the comparison of performance parameters like precision, recall, F1-score, and accuracy the algorithms are ranked and selected the best among all. The accuracy value of Logistic Regression is the highest among the other algorithm, therefore Logistic Regression performs best with the patient data in forecasting diabetes mellitus.
Key-Words / Index Term
Diabetes mellitus, Support Vector Machine (SVM), Naive Bayes (NB), Logistic Regression, Random forest, decision tree
References
[1] B. Sobandi, “Strategi Optimalisasi Pendapatan
Asli Daerah (PAD): Kasus Kota Banjarmasin”, LEMHANNAS RI, ISSN 08539340, 2004.
[2] A. Muhtarom, “Analisis PAD Terhadap
Kesejahteraan Masyarakat Kabupaten Lamongan Periode 2010-2015”, EKBIS Journal,
Vol.1, Issue. 8, 2015.
[3] M.A. Manuhutu, L.J. Uktolseja, “Design and
Implementation of Online Students’ Complaint (Case Study of English Study
Program at Victory University, Sorong)”, -IJCSE, Vol-6, Issue. 1, pp. 228-232,
2018.
[4] N. Bharanikumar, P. Dhanalakshmi, “Survey on
Machine Learning Algorithms for Classification and Prediction of Land Use
Changes Using GIS”, International Journal of Computer Sciences and Engineering,
Vol-7, Issue. 4, pp.351, 2019.
[5] R. Rendra, Wasilah, “Use of Cobit Framework
4.1 Method in Auditing The Academic Information System (Siakad) in Intan
Lampung Raden IAIN”, TIM Darmajaya Journal, Vol.1, Issue.1, pp.83-91, 2015
[6] D. Kennedy. “Introducing Geographic
Information Systems with Arc-GIS: A Workbook Approach to Learning GIS, 3rd
Edition”, Wiley, Chapter 9, 2013. ISBN no 9781118159804.
[7] G. Saxena, “Data Warehouse Designing:
Dimensional Modelling and E-R Modelling”, IJEI, Vol.3, Issue.9, pp.28-34, 2014.
[8] K.E. Kendall, J.E. Kendall, “SYSTEM ANALYSIS
AND DESIGN”, Prentice Hall, 2005, ISBN no 0130415715.
[9] A. Ambarwati, R. Rusady. “Analisis
Implementasi Teknologi Informasi pada Domain Deliver and Support di PT. RDPI”,
INFORM Journal, Vol.2, Issue.2, 2017.
[1]
W.
Rathmann, G. Giani, Global prevalence of diabetes: estimates for the year 2000
and projections for 2030, Diabetes Care 27 (10) (2004) 2568–2569
[2]
The
National Health and Nutrition Examination Survey (NHANES), 〈http:// www.cdc.gov/nchs/nhanes.htm〉, Last
accessed December 2012.
[3]
Pappada,
Scott M., Brent D. Cameron, and Paul M. Rosman. "Development of a neural
network for prediction of glucose concentration in type 1 diabetes
patients." Journal of diabetes science and technology 2.5 (2008): 792-801.
[4]
Cobelli,
C., Dalla Man, C., Sparacino, G., Magni, L., De Nicolao, G., & Kovatchev,
B. P. (2009). Diabetes: models, signals, and control. IEEE reviews in
biomedical engineering, 2, 54-96.
[5]
Pérez-Gandía,
C., Facchinetti, A., Sparacino, G., Cobelli, C., Gómez, E. J., Rigla, M., ...
& Hernando, M. E. (2010). Artificial neural network algorithm for online
glucose prediction from continuous glucose monitoring. Diabetes technology
& therapeutics, 12(1), 81-88.
[6]
Ash C,
Farrow JAE, Wallbanks S, Collins MD. Phylogenetic heterogeneity of the genus
bacillus revealed by comparativeanalysis of small subunit ribosomal RNA
sequences. Lett Appl Microbiol. 1991;13:202–6.
[7]
Audic
S, Claverie JM. The significance of digital gene expression profiles. Genome
Res. 1997;7:986–95.
[8]
Quinlan
JR, Rivest RL. Inferring decision trees using the minimum description lenght
principle. Inform Comput.1989;80(3):227–48.
[9]
Agrawal
R, Ghosh S, Imielinski T, Iyer B, Swami A. An interval classifier for database
mining applications. 1992. pp.560–73.
[10] Breiman L, Friedman JH, Olshen RA, Stone CJ.
Classification and regression trees. Belmont: Wadsworth International Group;
1984.
[11] Cortes, C., Vapnik, V., “Support-vector
networks”, Machine Learning, 20(2),pp. 273-297, 1995.
[12] V. Vapnik, “The Nature of Statistical
Learning Theory.” NY: Springer- Verlag. 1995.
[13] Rodríguez-Rodríguez, I., Rodríguez, J. V.,
Chatzigiannakis, I., & Zamora Izquierdo, M. Á. (2019). On the Possibility
of Predicting Glycaemia ‘On the Fly’with Constrained IoT Devices in Type 1
Diabetes Mellitus Patients. Sensors, 19(20), 4538.
[14] Zou, Q., Qu, K., Ju, Y., Tang, H., Luo, Y.,
& Yin, D. (2018). Predicting diabetes mellitus with machine learning
techniques. Frontiers in genetics, 9, 515.
[15] Mendez CE, Umpierrez GE. Management of type
1 diabetes in the hospital setting. Curr Diab Rep 2017;17:98
[16] Yogi-Morren D, Lansang MC. Management of
patients with type 1 diabetes in the hospital. Curr Diab Rep 2014;14:458
[17] Murad MH, Coburn JA, Coto-Yglesias F, et al.
Glycemic control in non-critically ill hospitalized patients:a systematic
reviewandmeta-analysis. J Clin Endocrinol Metab 2012; 97:49–58.
[18] Moghissi ES, Korytkowski MT, DiNardo M, et
al.; American Association of Clinical Endocrinologists; American Diabetes
Association. American Association of Clinical Endocrinologists and American
Diabetes Association consensus statement on inpatient glycemic control.
Diabetes Care 2009; 32:1119–1131
[19] Umpierrez GE, Hellman R, Korytkowski MT, et
al.; Endocrine Society. Management of hyperglycemia in hospitalized patients in
non-critical care setting: an Endocrine Society clinical practice guideline. J
Clin Endocrinol Metab 2012; 97: 16–38.
[20] Noschese ML, DiNardo MM, Donihi AC, et al.
Patient outcomes after implementation of a protocol for inpatient insulin pump
therapy. Endocr Pract 2009;15:415–424
[21] Adamson TL, Eusebio FA, Cook CB, LaBelle JT.
The promise of electrochemical impedance spectroscopy as novel technology for
the management of patients with diabetes mellitus. Analyst (Lond)
2012;137:4179–4187