A Survey on TAXO Finder: An Efficient Taxonomy Learning Using Graph Based Approach
Abhijeet Ashokrao Kadam1 , Shivputra Guruling Swami2
Section:Survey Paper, Product Type: Journal Paper
Volume-7 ,
Issue-12 , Page no. 132-134, Dec-2019
CrossRef-DOI: https://doi.org/10.26438/ijcse/v7i12.132134
Online published on Dec 31, 2019
Copyright © Abhijeet Ashokrao Kadam, Shivputra Guruling Swami . This is an open access article distributed under the Creative Commons Attribution License, which permits unrestricted use, distribution, and reproduction in any medium, provided the original work is properly cited.
View this paper at Google Scholar | DPI Digital Library
How to Cite this Paper
- IEEE Citation
- MLA Citation
- APA Citation
- BibTex Citation
- RIS Citation
IEEE Style Citation: Abhijeet Ashokrao Kadam, Shivputra Guruling Swami, “A Survey on TAXO Finder: An Efficient Taxonomy Learning Using Graph Based Approach,” International Journal of Computer Sciences and Engineering, Vol.7, Issue.12, pp.132-134, 2019.
MLA Style Citation: Abhijeet Ashokrao Kadam, Shivputra Guruling Swami "A Survey on TAXO Finder: An Efficient Taxonomy Learning Using Graph Based Approach." International Journal of Computer Sciences and Engineering 7.12 (2019): 132-134.
APA Style Citation: Abhijeet Ashokrao Kadam, Shivputra Guruling Swami, (2019). A Survey on TAXO Finder: An Efficient Taxonomy Learning Using Graph Based Approach. International Journal of Computer Sciences and Engineering, 7(12), 132-134.
BibTex Style Citation:
@article{Kadam_2019,
author = {Abhijeet Ashokrao Kadam, Shivputra Guruling Swami},
title = {A Survey on TAXO Finder: An Efficient Taxonomy Learning Using Graph Based Approach},
journal = {International Journal of Computer Sciences and Engineering},
issue_date = {12 2019},
volume = {7},
Issue = {12},
month = {12},
year = {2019},
issn = {2347-2693},
pages = {132-134},
url = {https://www.ijcseonline.org/full_paper_view.php?paper_id=4986},
doi = {https://doi.org/10.26438/ijcse/v7i12.132134}
publisher = {IJCSE, Indore, INDIA},
}
RIS Style Citation:
TY - JOUR
DO = {https://doi.org/10.26438/ijcse/v7i12.132134}
UR - https://www.ijcseonline.org/full_paper_view.php?paper_id=4986
TI - A Survey on TAXO Finder: An Efficient Taxonomy Learning Using Graph Based Approach
T2 - International Journal of Computer Sciences and Engineering
AU - Abhijeet Ashokrao Kadam, Shivputra Guruling Swami
PY - 2019
DA - 2019/12/31
PB - IJCSE, Indore, INDIA
SP - 132-134
IS - 12
VL - 7
SN - 2347-2693
ER -
![]() |
![]() |
![]() |
206 | 188 downloads | 131 downloads |
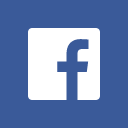
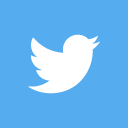
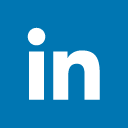
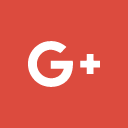
Abstract
Taxonomy learning knowledge is an essential project for knowledge acquisition, sharing, and classification in addition to utility development and usage in diverse domains. To decrease human effort to build a taxonomy from scratch and enhance the quality of discovered taxonomy, we endorse a brand new taxonomy gaining knowledge of method, named taxo finder. Taxofinder takes 3 steps to mechanically build a taxonomy. First, it identifies domain-specific standards from a website textual content corpus. 2nd, it builds a graph representing how such standards are related collectively primarily based on their co-occurrences. As the key approach in taxofinder, the taxonomy may be built manually however it`s far a complex manner when the information is so huge and it additionally produces some errors while taxonomy production. There may be diverse automated taxonomy creation techniques that are used to study taxonomy based totally on key-word terms, text corpus and from domain particular principles and so on. So it`s far required to construct taxonomy with less human effort and with much less error price. This paper affords certain records about those techniques.
Key-Words / Index Term
Taxonomy learning, knowledge searching, TaxoFinder, keyword phrases
References
[1] S. Porna Sai, S. Udhaya, R. Suganya, K.S. Sangeetha. Supporting Privacy Protection in Personalized Web Search - A Survey. Indian Journal of Innovations and Developments. 2014, 3(3), 45-49.
[2] F. Fallucchi, F. M. Zanzotto. Inductive probabilistic taxonomy learning using singular value decomposition. Natural Language Engineering. 2011, 17(01), 71-94.
[3] Z. Kozareva, E. A. Hovy. semi-supervised method to learn and construct taxonomies using the web. In Proceedings of the 2010 conference on empirical methods in natural language processing. Association for Computational Linguistics. 2010, 1110-1118.
[4] M. S. Paukkeri, A. P. García-Plaza, S. Pessala, T. Honkela. Learning taxonomic relations from a set of text documents. In Computer Science and Information Technology (IMCSIT), Proceedings of the 2010 International Multiconference. 2010, 105-112.
[5] W. Wang, P. M.Barnaghi, A. Bargiela. Probabilistic topic models for learning terminological ontologies. IEEE Transactions on Knowledge and Data Engineering. 2010, 22(7), 1028-1040.
[6] I. Novalija, D. Mladenić, L. Bradeško. OntoPlus: Text-driven ontology extension using ontology content, structure, and co-occurrence information. Knowledge-Based Systems. 2011, 24(8), 1261-1276
[7] S. P. Ponzetto, M. Strube. Taxonomy induction based on a collaboratively built knowledge repository. Artificial Intelligence. 2011, 175(9-10), 1737-1756.
[8] E. A. Dietz, D. Vandic, F. Frasincar. Taxolearn: A semantic approach to domain taxonomy learning. In Web Intelligence and Intelligent Agent Technology (WI-IAT). 2012; 1, 58-65.
[9] T. Fountain, M. Lapata. Taxonomy induction using hierarchical random graphs. In Proceedings of the 2012 Conference of the North American Chapter of the Association for Computational Linguistics: Human Language Technologies.2012, 466-476.
[10] X. Liu, Y. Song, S. Liu, H. Wang. Automatic taxonomy construction from keywords. In Proceedings of the 18th ACM SIGKDD international conference on Knowledge discovery and data mining. 2012, 1433-1441.
[11] H. Yang. Constructing task-specific taxonomies for document collection browsing. In Proceedings of the 2012 Joint Conference on Empirical Methods in Natural Language Processing and Computational Natural Language Learning. 2012; 1278-1289.
[12] J. De Knijff, F. Frasincar, F. Hogenboom. Domain taxonomy learning from text: The subsumption method versus hierarchical clustering. Data & Knowledge Engineering 2013; 83, 54-69.