A Reliable Solution for Sparsity Problem in Collaborative Filtering Using Demographic Approach
Kaira Nithin Goud1
Section:Research Paper, Product Type: Journal Paper
Volume-7 ,
Issue-9 , Page no. 54-59, Sep-2019
CrossRef-DOI: https://doi.org/10.26438/ijcse/v7i9.5459
Online published on Sep 30, 2019
Copyright © Kaira Nithin Goud . This is an open access article distributed under the Creative Commons Attribution License, which permits unrestricted use, distribution, and reproduction in any medium, provided the original work is properly cited.
View this paper at Google Scholar | DPI Digital Library
How to Cite this Paper
- IEEE Citation
- MLA Citation
- APA Citation
- BibTex Citation
- RIS Citation
IEEE Style Citation: Kaira Nithin Goud, “A Reliable Solution for Sparsity Problem in Collaborative Filtering Using Demographic Approach,” International Journal of Computer Sciences and Engineering, Vol.7, Issue.9, pp.54-59, 2019.
MLA Style Citation: Kaira Nithin Goud "A Reliable Solution for Sparsity Problem in Collaborative Filtering Using Demographic Approach." International Journal of Computer Sciences and Engineering 7.9 (2019): 54-59.
APA Style Citation: Kaira Nithin Goud, (2019). A Reliable Solution for Sparsity Problem in Collaborative Filtering Using Demographic Approach. International Journal of Computer Sciences and Engineering, 7(9), 54-59.
BibTex Style Citation:
@article{Goud_2019,
author = {Kaira Nithin Goud},
title = {A Reliable Solution for Sparsity Problem in Collaborative Filtering Using Demographic Approach},
journal = {International Journal of Computer Sciences and Engineering},
issue_date = {9 2019},
volume = {7},
Issue = {9},
month = {9},
year = {2019},
issn = {2347-2693},
pages = {54-59},
url = {https://www.ijcseonline.org/full_paper_view.php?paper_id=4849},
doi = {https://doi.org/10.26438/ijcse/v7i9.5459}
publisher = {IJCSE, Indore, INDIA},
}
RIS Style Citation:
TY - JOUR
DO = {https://doi.org/10.26438/ijcse/v7i9.5459}
UR - https://www.ijcseonline.org/full_paper_view.php?paper_id=4849
TI - A Reliable Solution for Sparsity Problem in Collaborative Filtering Using Demographic Approach
T2 - International Journal of Computer Sciences and Engineering
AU - Kaira Nithin Goud
PY - 2019
DA - 2019/09/30
PB - IJCSE, Indore, INDIA
SP - 54-59
IS - 9
VL - 7
SN - 2347-2693
ER -
![]() |
![]() |
![]() |
342 | 337 downloads | 143 downloads |
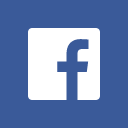
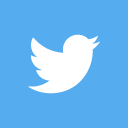
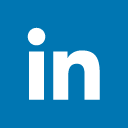
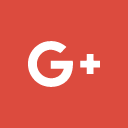
Abstract
Now a day’s online resources are increasing very rapidly like amazon and flipchart, eBay etc. The main role of recommendation systems is to provide recommendations based upon the ratings given by the users.it suffers from the sparsity to reduce that we are going to introduce a reliable solution that motives to perform better results using a demographic approach. Each prediction consorts with a reliability measure. Reliability is a measure of how liable a prediction is. So each recommendation for a user is associated with a pair of values those are Prediction and reliability. Quality of reliability is also discussed. Experimental results show that our proposed reliable solution using demographic approach has increased the overall recommendation and reduced the sparsity.
Key-Words / Index Term
Recommender systems, Collaborative filtering, prediction, reliability, location
References
[1] G. Adomavicius, A. Tuzhilin, Toward the next generation of recommender systems: a survey of the state-of-the-art and possible extensions, IEEE.
[2] S. Alonso, E. Herrera-Viedma, F. Chiclana, F. Herrera, A web based consensus support system for group decision making problems and incomplete preferences, Information Sciences 180 (23) (2010) 4477–4495.
[3] N. Antonopoulus, J. Salter, Cinema screen recommender agent: combining collaborative and content-based filtering, IEEE Intelligent Systems (2006) 35–41.
[4] A. Barragáns-Martínez, E. Costa-Montenegro, J. Burguillo, M. Rey-López, F. Mikic-Fonte, A. Peleteiro, A hybrid content-based and item-based collaborative filtering approach to recommend tv programs enhanced with singular value decomposition, Inform. Sci. 180 (22) (2010) 4290–4311.
[5]S. Zhang, W. Wang, J. Ford, F. Makedon, Learning from incomplete ratings using non-negative matrix factorization, in: SDM, SIAM, 2006, pp.549–553.
[6] G. Beliakov, G. Li, Improving the speed and stability of the k-nearest neighbors method, Pattern Recognition Letters 33 (2012) 1296–1301.
[7] J. Bobadilla, F. Ortega, A. Hernando, A. Gutiérrez, Recommender systems survey, Knowledge-Based Systems 46 (2013) 109–132.
[8] P. Resnick, H.R. Varian, Recommender systems, Communications of the ACM 40 (1997) 56–58.
[9]S.AmerYahia,S.B.Roy,A.Chawlat,G.Das,C.Yu,Group recommendation : semantics and efficiency ,in: Proceedings of the 35th International Conference on Very Large DataBases,2009,pp.754–765.
[10]B.Sarwar,G.Karypis,J.Konstan,J.Riedl,Item-based collaborative filtering recommendation algorithms, in: Proceedings of the 10th International Conference on World Wide Web, WWW`01, ACM, NewYork, NY, USA, 2001, pp. 285–295.
[11] K. Ali and W. van Stam, “TiVo: Making show recommendations using a distributed collaborative filtering architecture,” in ACM KDD ’04, pp. 394–401, ACM, 2004.
[12] X. Amatriain, J. Pujol, and N. Oliver, “I like it. . . I like it not: Evaluating user ratings noise in recommender systems,” in UMAP 2009, vol. 5535 of LectureNotes in Computer Science, pp. 247–258, Springer, 2009.
[13] X. Amatriain, J. M. Pujol, N. Tintarev, and N. Oliver, “Rate it again:Increasing recommendation accuracy by user re-rating,” in ACM RecSys ’09,pp. 173–180, ACM, 2009.
[14] C. De Rosa, J. Cantrell, A. Havens, J. Hawk, L. Jenkins, B. Gauder, R. Limes, D. Cellentani, and OCLC,Sharing, privacy and trust in our networked world: A report to the OCLC membership. OCLC, 2007.
[15] X. Zhou, Y. Xu, Y. Li, A. Josang, and C. Cox, “The state-of-the-art in personalized recommender systems.