Soft computing to determine a Hemoglobin level of an early stage Multiple Myeloma patient by using Rectified Linear Units (ReLu) activation function
Akshansh Mishra1 , Mitali Diwan2
Section:Research Paper, Product Type: Journal Paper
Volume-7 ,
Issue-9 , Page no. 26-30, Sep-2019
CrossRef-DOI: https://doi.org/10.26438/ijcse/v7i9.2630
Online published on Sep 30, 2019
Copyright © Akshansh Mishra, Mitali Diwan . This is an open access article distributed under the Creative Commons Attribution License, which permits unrestricted use, distribution, and reproduction in any medium, provided the original work is properly cited.
View this paper at Google Scholar | DPI Digital Library
How to Cite this Paper
- IEEE Citation
- MLA Citation
- APA Citation
- BibTex Citation
- RIS Citation
IEEE Style Citation: Akshansh Mishra, Mitali Diwan, “Soft computing to determine a Hemoglobin level of an early stage Multiple Myeloma patient by using Rectified Linear Units (ReLu) activation function,” International Journal of Computer Sciences and Engineering, Vol.7, Issue.9, pp.26-30, 2019.
MLA Style Citation: Akshansh Mishra, Mitali Diwan "Soft computing to determine a Hemoglobin level of an early stage Multiple Myeloma patient by using Rectified Linear Units (ReLu) activation function." International Journal of Computer Sciences and Engineering 7.9 (2019): 26-30.
APA Style Citation: Akshansh Mishra, Mitali Diwan, (2019). Soft computing to determine a Hemoglobin level of an early stage Multiple Myeloma patient by using Rectified Linear Units (ReLu) activation function. International Journal of Computer Sciences and Engineering, 7(9), 26-30.
BibTex Style Citation:
@article{Mishra_2019,
author = {Akshansh Mishra, Mitali Diwan},
title = {Soft computing to determine a Hemoglobin level of an early stage Multiple Myeloma patient by using Rectified Linear Units (ReLu) activation function},
journal = {International Journal of Computer Sciences and Engineering},
issue_date = {9 2019},
volume = {7},
Issue = {9},
month = {9},
year = {2019},
issn = {2347-2693},
pages = {26-30},
url = {https://www.ijcseonline.org/full_paper_view.php?paper_id=4843},
doi = {https://doi.org/10.26438/ijcse/v7i9.2630}
publisher = {IJCSE, Indore, INDIA},
}
RIS Style Citation:
TY - JOUR
DO = {https://doi.org/10.26438/ijcse/v7i9.2630}
UR - https://www.ijcseonline.org/full_paper_view.php?paper_id=4843
TI - Soft computing to determine a Hemoglobin level of an early stage Multiple Myeloma patient by using Rectified Linear Units (ReLu) activation function
T2 - International Journal of Computer Sciences and Engineering
AU - Akshansh Mishra, Mitali Diwan
PY - 2019
DA - 2019/09/30
PB - IJCSE, Indore, INDIA
SP - 26-30
IS - 9
VL - 7
SN - 2347-2693
ER -
![]() |
![]() |
![]() |
1180 | 348 downloads | 188 downloads |
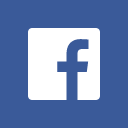
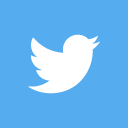
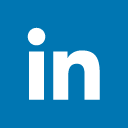
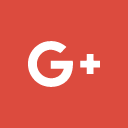
Abstract
Artificial Intelligence (AI) has found various applications in many industries, from development of new alloys to cyber security and healthcare domain. By 2025 it is expected that the market for healthcare artificial intelligence tools will surpass 34 billion dollars. There is no doubt that the application of AI is going to lead to a real digital shift in traditional medical imaging, requiring AI and people to work together to meet the challenges of the medical industry. In our present work, we have tried to determine the hemoglobin level corresponding to Packed Cell Volume (PCV) and Red Blood Cells (RBC) count. In the Artificial Neural Network (ANN) architecture, PCV (%) and RBC count (mill/cumm) are the inputs while hemoglobin (g/dL) is the output. The result obtained is quite promising. Artificial Neural Network (ANN) trained on Rectified Linear Unit (ReLu) activation function showed 97.15% accuracy.
Key-Words / Index Term
Multiple Myeloma, Artificial Intelligence, Artificial Neural Network, Hemoglobin level
References
[1] Patel, V.L., Shortliffe, E.H., Stefanelli, M., Szolovits, P., Berthold, M.R., Bellazzi, R. and Abu-Hanna, A., 2009. The coming of age of artificial intelligence in medicine. Artificial intelligence in medicine, 46(1), pp.5-17.
[2] Jaremko, Jacob L. et al. Canadian Association of Radiologists Journal, Volume 70, Issue 2, 107 – 118
[3] Soni, J., Ansari, U., Sharma, D. and Soni, S., 2011. Predictive data mining for medical diagnosis: An overview of heart disease prediction. International Journal of Computer Applications, 17(8), pp.43-48.
[4] Lo, S.C., Lou, S.L., Lin, J.S., Freedman, M.T., Chien, M.V. and Mun, S.K., 1995. Artificial convolution neural network techniques and applications for lung nodule detection. IEEE Transactions on Medical Imaging, 14(4), pp.711-718.