Optimization in Feature Extraction schemes on Static Images to Improve the Performance of Automatic Facial Expression Recognition Systems
aveen Kumar H N1 , Jagadeesha S2 , Amith K Jain3
Section:Research Paper, Product Type: Journal Paper
Volume-7 ,
Issue-6 , Page no. 1104-1109, Jun-2019
CrossRef-DOI: https://doi.org/10.26438/ijcse/v7i6.11041109
Online published on Jun 30, 2019
Copyright © Naveen Kumar H N, Jagadeesha S, Amith K Jain . This is an open access article distributed under the Creative Commons Attribution License, which permits unrestricted use, distribution, and reproduction in any medium, provided the original work is properly cited.
View this paper at Google Scholar | DPI Digital Library
How to Cite this Paper
- IEEE Citation
- MLA Citation
- APA Citation
- BibTex Citation
- RIS Citation
IEEE Citation
IEEE Style Citation: Naveen Kumar H N, Jagadeesha S, Amith K Jain, “Optimization in Feature Extraction schemes on Static Images to Improve the Performance of Automatic Facial Expression Recognition Systems,” International Journal of Computer Sciences and Engineering, Vol.7, Issue.6, pp.1104-1109, 2019.
MLA Citation
MLA Style Citation: Naveen Kumar H N, Jagadeesha S, Amith K Jain "Optimization in Feature Extraction schemes on Static Images to Improve the Performance of Automatic Facial Expression Recognition Systems." International Journal of Computer Sciences and Engineering 7.6 (2019): 1104-1109.
APA Citation
APA Style Citation: Naveen Kumar H N, Jagadeesha S, Amith K Jain, (2019). Optimization in Feature Extraction schemes on Static Images to Improve the Performance of Automatic Facial Expression Recognition Systems. International Journal of Computer Sciences and Engineering, 7(6), 1104-1109.
BibTex Citation
BibTex Style Citation:
@article{N_2019,
author = {Naveen Kumar H N, Jagadeesha S, Amith K Jain},
title = {Optimization in Feature Extraction schemes on Static Images to Improve the Performance of Automatic Facial Expression Recognition Systems},
journal = {International Journal of Computer Sciences and Engineering},
issue_date = {6 2019},
volume = {7},
Issue = {6},
month = {6},
year = {2019},
issn = {2347-2693},
pages = {1104-1109},
url = {https://www.ijcseonline.org/full_paper_view.php?paper_id=4689},
doi = {https://doi.org/10.26438/ijcse/v7i6.11041109}
publisher = {IJCSE, Indore, INDIA},
}
RIS Citation
RIS Style Citation:
TY - JOUR
DO = {https://doi.org/10.26438/ijcse/v7i6.11041109}
UR - https://www.ijcseonline.org/full_paper_view.php?paper_id=4689
TI - Optimization in Feature Extraction schemes on Static Images to Improve the Performance of Automatic Facial Expression Recognition Systems
T2 - International Journal of Computer Sciences and Engineering
AU - Naveen Kumar H N, Jagadeesha S, Amith K Jain
PY - 2019
DA - 2019/06/30
PB - IJCSE, Indore, INDIA
SP - 1104-1109
IS - 6
VL - 7
SN - 2347-2693
ER -
![]() |
![]() |
![]() |
429 | 639 downloads | 212 downloads |
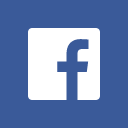
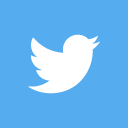
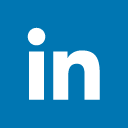
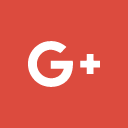
Abstract
Automatic Facial Expression Recognition (AFER) systems are gaining importance in various emerging Human Computer Interaction (HCI) applications and affective computing applications. The abstract and robust features to interpret facial expressions and encode them as an emotion, still, remain as a challenge in the field of AFER. The objective of the proposed work is to analyze the performance of still image based AFER system with respect to various feature extraction schemes, and to optimize and thereby improving the recognition accuracy of AFER systems. Features such as; Histograms of Oriented Gradients (HOG), Local Binary Pattern (LBP) and a combination of HOG-LBP are used for the analysis of AFER system performance on feature extraction schemes. Various Parameters corresponding to features of interest are involved during the experimentation to understand the impact of a particular feature parameter on the recognition rate of AFER system. It’s not a simple task to optimize parameters of a feature to achieve better recognition rates. The proposed work is implemented on Extended Cohn-Kanade (CK+) dataset for six expressions. Cell size parameter of the features experimented has shown improvement in performance. Experimental results demonstrate the effectiveness of the proposed work on still image based facial expression recognition by providing significant performance improvement over other methods under comparison.
Key-Words / Index Term
Facial Expression Recognition, Feature Extraction, Feature combination, Image Classification, Texture Descriptor, Human Computer Interaction Component
References
[1] P. Ekman and W. Friesen, “Constants across cultures in the face and emotion”, Journal of personality and social psychology, vol.17, no.2, p-124, 1971.
[2] Z.Zeng, M.Pantic, G.I.Roisman and T. S. Huang,”A survey of affect recognition methods: Audio, visual and spontaneous expressions”, IEEE IEEE Transactions on Pattern Analysis and Machine Intelligence, vol.31 no. 1, pp. 39-58, 2009.
[3] Xiaoming Zhao and Shiqing Zhang, “A Review on Facial Expression Recognition: Feature Extraction and Classification”, IETE Technical Review, Vol. 33 Issue- 5, 2016.
[4] I.Kotsia and I. Pitas, “Facial expression recognition in image sequences using geometric deformation features and support vector machines”, IEEE Transactions on Image Processing, vol.16, no.1, pp. 172-187, Jan 2007.
[5] P. Ekman and W. Friesen, “The Facial Action Coding System (FACS): A Technique for the Measurement of Facial Movement”, Consulting Psychologists Press, San Francisco, 1978.
[6] Ziheng Wang, Shangfei Wang,Qiang Ji, “Capturing Complex Spatio-Temporal Relations among Facial Muscles for Facial Expression Recognition”, IEEE Conference on Computer Vision and Pattern Recognition.
[7] K. Sikka, T. Wu, J. Susskind, and M. Bartlett, “Exploring bag of words architectures in the facial expression domain”, in Proc. European Conf. Computer Vision Workshops, pp. 250–259, 2012.
[8] S L Happy and Aurobinda Routray, “Automatic facial expression recognition using features of salient facial patches”, IEEE Transactions on Affective Computing.
[9] Navneet Dalal and Bill Triggs, “Histograms of Oriented Gradients for Human Detection”, in the Proceedings of Conference on Computer Vision and Pattern Recognition 2005.
[10] Shan, Sh. Gong, P. W. McOwan, “Facial expression recognition based on local binary patterns: a comprehensive study”, Image and Vision Computing, vol. 27, no. 6, pp. 803-816, 2009.
[11] T. Ahonen, A, Hadid, and M. Pietikainen, “Face description with local binary patterns: Application to face recognition”, IEEE Transactions on Pattern Analysis and Machine Intelligence, vol.28, pp. 2037-2041, 2006.
[12] W. Hsu and C.-J. Lin, "A comparison of methods for multiclass support vector machines", IEEE Transactions on Neural Networks, vol. 13, no. 2, pp. 415-425, 2002.
[13] M.Regina, M.S. Josephine, V. Jeyabalraja,”Multi-featured extraction and convolution neural network based SVM for automatic facial emotion recognition”, International Journal of Computer Science and Engineering, Vol.7, Issue 5, 2019.