Gray Level Cooccurrence Matrix Feature Extraction and Fuzzy Based Discriminative Binary Descriptor for Medical Image Retrieval
N.T. Renukadevi1 , S. Karunakaran2 , K. Saraswathi3
Section:Research Paper, Product Type: Journal Paper
Volume-7 ,
Issue-6 , Page no. 62-70, Jun-2019
CrossRef-DOI: https://doi.org/10.26438/ijcse/v7i6.6270
Online published on Jun 30, 2019
Copyright © N.T. Renukadevi, S. Karunakaran, K. Saraswathi . This is an open access article distributed under the Creative Commons Attribution License, which permits unrestricted use, distribution, and reproduction in any medium, provided the original work is properly cited.
View this paper at Google Scholar | DPI Digital Library
How to Cite this Paper
- IEEE Citation
- MLA Citation
- APA Citation
- BibTex Citation
- RIS Citation
IEEE Style Citation: N.T. Renukadevi, S. Karunakaran, K. Saraswathi, “Gray Level Cooccurrence Matrix Feature Extraction and Fuzzy Based Discriminative Binary Descriptor for Medical Image Retrieval,” International Journal of Computer Sciences and Engineering, Vol.7, Issue.6, pp.62-70, 2019.
MLA Style Citation: N.T. Renukadevi, S. Karunakaran, K. Saraswathi "Gray Level Cooccurrence Matrix Feature Extraction and Fuzzy Based Discriminative Binary Descriptor for Medical Image Retrieval." International Journal of Computer Sciences and Engineering 7.6 (2019): 62-70.
APA Style Citation: N.T. Renukadevi, S. Karunakaran, K. Saraswathi, (2019). Gray Level Cooccurrence Matrix Feature Extraction and Fuzzy Based Discriminative Binary Descriptor for Medical Image Retrieval. International Journal of Computer Sciences and Engineering, 7(6), 62-70.
BibTex Style Citation:
@article{Renukadevi_2019,
author = {N.T. Renukadevi, S. Karunakaran, K. Saraswathi},
title = {Gray Level Cooccurrence Matrix Feature Extraction and Fuzzy Based Discriminative Binary Descriptor for Medical Image Retrieval},
journal = {International Journal of Computer Sciences and Engineering},
issue_date = {6 2019},
volume = {7},
Issue = {6},
month = {6},
year = {2019},
issn = {2347-2693},
pages = {62-70},
url = {https://www.ijcseonline.org/full_paper_view.php?paper_id=4509},
doi = {https://doi.org/10.26438/ijcse/v7i6.6270}
publisher = {IJCSE, Indore, INDIA},
}
RIS Style Citation:
TY - JOUR
DO = {https://doi.org/10.26438/ijcse/v7i6.6270}
UR - https://www.ijcseonline.org/full_paper_view.php?paper_id=4509
TI - Gray Level Cooccurrence Matrix Feature Extraction and Fuzzy Based Discriminative Binary Descriptor for Medical Image Retrieval
T2 - International Journal of Computer Sciences and Engineering
AU - N.T. Renukadevi, S. Karunakaran, K. Saraswathi
PY - 2019
DA - 2019/06/30
PB - IJCSE, Indore, INDIA
SP - 62-70
IS - 6
VL - 7
SN - 2347-2693
ER -
![]() |
![]() |
![]() |
511 | 460 downloads | 179 downloads |
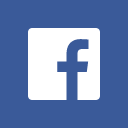
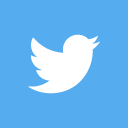
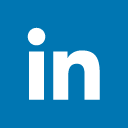
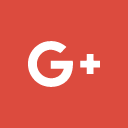
Abstract
Medical image retrieval plays an more important role in the medical research environment which needs to done fastly and accurately for improved performance. In our previous research method it is done by introducing coiflets wavelet based feature extraction and SVM based classification. However this research method cannot perform well with the presence of increased noise level and the minuter feature information. This is resolved in this research method by introducing method namely Gray Level Co occurrence Matrix Feature Extraction and Fuzzy Based Discriminative Binary Descriptor (GLCMFE-FBDBD). It contains five major steps such as deblurring, preprocessing, feature extraction, detection of most discriminative bin and subspace clustering. In this research method, the image deblurring is accomplished by utilizing Artificial Bee Colony (ABC) algorithm. Preprocessing is done by using min-max normalization; feature extraction is done by using gray level concurrence matrix Then FSK Function is used to discover the most discriminative bin selection. SC is presented for quick image retrieval. The MRI brain tumor images are used for evaluation. Finally, the results show that the proposed work gives greater performance compared to the previous work.
Key-Words / Index Term
Image retrieval, Edge Scale-Invariant Feature Transform (ESIFT), Image deblurring, Artificial Bee Colony (ABC), Subspace Clustering (SC) algorithm, Fuzzy Sigmoid Kernel (FSK)
References
[1] Rothganger F, Lazebnik S, Schmid, C, and Ponce, J. “3D object modeling and recognition using local affine-invariant image descriptors and multi-view spatial constraints”, International Journal of Computer Vision, vol. 3, Issue :66, pp: 231-259,2006.
[2] Trzcinski T, Christoudias M, Fua P, and Lepetit V, “Boosting binary keypoint descriptors”, In Proceedings of the IEEE conference on computer vision and pattern recognition. pp.2874-2881,2013.
[3] Zhou W, Yang M, Li H, Wang X, Lin Y, and Tian Q, “Towards Codebook-Free: Scalable Cascaded Hashing for Mobile Image Search”, IEEE Trans. Multimedia, vol:16, issue:3, pp. 601-611,2014.
[4] Fang B, Leung CH, Tang YY, Kwok PC and Wong YK, “Off-line signature verification by the tracking of feature and stroke positions”, Pattern recognition, vol.36, issue:1, pp: 91-101,2003.
[5] Yang Y, and Newsam S, “Bag-of-visual-words and spatial extensions for land-use classification”, In Proceedings of the 18th SIGSPATIAL international conference on advances in geographic information systems, pp. 270-279, 2010.
[6] Bhowmik N, González, VR, Gouet-Brunet V, Pedrini H, and Bloch G, “Efficient fusion of multidimensional descriptors for image retrieval”, In Proceedings of the IEEE International Conference on Image Processing, pp. 5766-5770,2014.
[7] Zhang S, Tian Q, Lu K, Huang Q, and Gao W, “Edge-SIFT: Discriminative binary descriptor for scalable partial-duplicate mobile search”, IEEE Trans. on Image Processing, vol.22, issue.7,pp. 2889-2902, 2010.
[8] Zhou W, Lu Y, Li H, and Tian Q, “Scalar quantization for large scale image search”, In Proceedings of the 20th ACM international conference on Multimedia, pp. 169-178, 2012.
[9] Liu Z, and Stasko J., “Mental models, visual reasoning and interaction in information visualization: A top-down perspective”, IEEE Trans. on Visualization & Computer Graphics, vol.6, pp. 999-1008, 2010.
[10] Grauman K and Darrell T, “The pyramid match kernel: Discriminative classification with sets of image features”, In Proceedings of the Tenth IEEE International Conference on Computer Vision,vol.2, pp.1458-1465,2005.
[11] Jegou H, Harzallah, H, and Schmid, “A contextual dissimilarity measure for accurate and efficient image search”, In Proceedings of the IEEE Conference on Computer Vision and Pattern Recognition, pp. 1-8,2007.
[12] Hong R Li L, Cai J, Tao D, Wang M, and Tian Q, “Coherent semantic-visual indexing for large-scale image retrieval in the cloud”, IEEE Trans. on Image Processing, vol.26,issue.9,pp: 4128-4138,2017
[13] Xu X Liu Z Wang Z Sheng Q Z Yu J and Wang X, "S-ABC: a paradigm of service domain-oriented artificial bee colony algorithms for service selection and composition”, Future Generation Computer Systems, vol. 68, pp. 304-319,2017.
[14] Rosten E Porter R and Drummond T, “Faster and better: A machine learning approach to corner detection”, IEEE Trans. on pattern analysis and machine intelligence, vol.32,issue.1,pp.105-119, 2012.
[15] Shrestha S and Kazama, F, “Assessment of surface water quality using multivariate statistical techniques: A case study of the Fuji river basin, Japan”, Environmental Modeling & Software, vol.22, issue.4, pp: 464-475,2007.