K-modes and Fuzzy C-means with modified Particle Swam Optimization Clustering Algorithm for Epilepsy Seizure Data
C.V. Banupriya1 , D. Deviaruna2
Section:Research Paper, Product Type: Journal Paper
Volume-7 ,
Issue-1 , Page no. 73-77, Jan-2019
CrossRef-DOI: https://doi.org/10.26438/ijcse/v7i1.7377
Online published on Jan 31, 2019
Copyright © C.V. Banupriya, D. Deviaruna . This is an open access article distributed under the Creative Commons Attribution License, which permits unrestricted use, distribution, and reproduction in any medium, provided the original work is properly cited.
View this paper at Google Scholar | DPI Digital Library
How to Cite this Paper
- IEEE Citation
- MLA Citation
- APA Citation
- BibTex Citation
- RIS Citation
IEEE Style Citation: C.V. Banupriya, D. Deviaruna, “K-modes and Fuzzy C-means with modified Particle Swam Optimization Clustering Algorithm for Epilepsy Seizure Data,” International Journal of Computer Sciences and Engineering, Vol.7, Issue.1, pp.73-77, 2019.
MLA Style Citation: C.V. Banupriya, D. Deviaruna "K-modes and Fuzzy C-means with modified Particle Swam Optimization Clustering Algorithm for Epilepsy Seizure Data." International Journal of Computer Sciences and Engineering 7.1 (2019): 73-77.
APA Style Citation: C.V. Banupriya, D. Deviaruna, (2019). K-modes and Fuzzy C-means with modified Particle Swam Optimization Clustering Algorithm for Epilepsy Seizure Data. International Journal of Computer Sciences and Engineering, 7(1), 73-77.
BibTex Style Citation:
@article{Banupriya_2019,
author = {C.V. Banupriya, D. Deviaruna},
title = {K-modes and Fuzzy C-means with modified Particle Swam Optimization Clustering Algorithm for Epilepsy Seizure Data},
journal = {International Journal of Computer Sciences and Engineering},
issue_date = {1 2019},
volume = {7},
Issue = {1},
month = {1},
year = {2019},
issn = {2347-2693},
pages = {73-77},
url = {https://www.ijcseonline.org/full_paper_view.php?paper_id=3464},
doi = {https://doi.org/10.26438/ijcse/v7i1.7377}
publisher = {IJCSE, Indore, INDIA},
}
RIS Style Citation:
TY - JOUR
DO = {https://doi.org/10.26438/ijcse/v7i1.7377}
UR - https://www.ijcseonline.org/full_paper_view.php?paper_id=3464
TI - K-modes and Fuzzy C-means with modified Particle Swam Optimization Clustering Algorithm for Epilepsy Seizure Data
T2 - International Journal of Computer Sciences and Engineering
AU - C.V. Banupriya, D. Deviaruna
PY - 2019
DA - 2019/01/31
PB - IJCSE, Indore, INDIA
SP - 73-77
IS - 1
VL - 7
SN - 2347-2693
ER -
![]() |
![]() |
![]() |
395 | 252 downloads | 152 downloads |
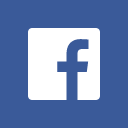
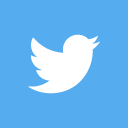
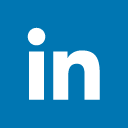
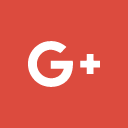
Abstract
Epilepsy is a stable neurological disorder of the brain, described by regular seizures, i.e., irregular activities. Seizure is the most imperative signal of epilepsy, which is solitary of the most expected neurological disorders. An electroencephalogram (EEG) is a test out used to weigh up the electrical activity in the brain, and is widely used in the recognition and study of epileptic seizures. Hence, it is decisive to develop a quantitative technique to automatically clustering the normal and epileptic brain activities. Several techniques have been developed for unbending out the important features of seizures present in EEGs. The proposed approach is evaluated an extracting the features of EEG signals using wavelet transform coefficients and unsupervised learning technique like clustering the data using Fuzzy C- Means with Modified Particle Swarm Optimization (PSO) and K- Mode Clustering. The recital of the Clusters are analyzed and examined that Fuzzy C-Means with PSO less error rate and out performs than K-Mode Clustering in accuracy.
Key-Words / Index Term
Fuzzy C-means, K-mode, EEG, Seizures, Wavelet, PSO, Clustering
References
[1] World Health Organization, 2011.Available: http://www.who.int/mediacentre/?factsheets/fs999/en/.
[2] J. Gotman, “Automatic detection of seizures and spikes,” J. Clin. Neurophysiol. 16: 130-140, 1999.
[3] James, C. J. “Detection of epileptic form activity in the electroencephalogram using the electroencephalogram using artificial neural networks”, University of Canterbury, Christchurch, 1997.
[4] J.D. Bronzing, “Biomedical Engineering Handbook”, New York: CRC Press LLC, Vol. I, 2nd edition 2000.
[5] Hojjat Adeli, Samanwoy Ghosh and Dastidat, “Automated EEG Based Diagnosis of Neurological Disorders”, CRC Press; 1 edition, 2010.
[6] Aarabi, R. Fazel-Rezai and Y. Aghakhani, “A fuzzy rule-based system for epileptic seizure detection in intracranial EEG,” Clin. Neurophysiology, 120: 1648-1657, 2009.
[7] H. Adeli, S. Ghosh-Dastidar and N. Dadmehr, “A Wavelet-Chaos methodology for analysis of EEGs and EEG sub bands to detect seizure and epilepsy,” IEEE Trans. Biomed. Eng., 54: 205-211, 2007.
[8] S. Tong and N. V. Thakor (Ed.), “Quantitative EEG Analysis Methods and Clinical Applications”, Norwood: Artech House, 2009.
[9] Varsavsky, I., Mareels and M. Cook, “Epileptic seizures and the EEG”, Boca Raton: CRC Press, 2011.
[10] Zhexue Huang, A Fast Clustering Algorithm to Cluster Very Large Categorical Data Sets in Data Mining.
[11] Guo Tao, Ding Xingu, Li Yefeng, Parallel k-modes Algorithm based on MapReduce.
[12] S. Agrawal, R. Panda, L. Dora, "A study on fuzzy clustering for magnetic resonance brain image segmentation using soft computing approaches", Applied Soft Computing, 2014.
[13] D. L. Pham, "Fuzzy Clustering With Spatial Constraints", Image Processing. 2002. Proceedings. 2002 International Conference, 2002.