Analysis of Naïve Bayes Classification for Diabetes Mellitus
S. Sankaranarayanan1 , T. Pramananda Perumal2
Section:Review Paper, Product Type: Journal Paper
Volume-6 ,
Issue-12 , Page no. 520-524, Dec-2018
CrossRef-DOI: https://doi.org/10.26438/ijcse/v6i12.520524
Online published on Dec 31, 2018
Copyright © S. Sankaranarayanan, T. Pramananda Perumal . This is an open access article distributed under the Creative Commons Attribution License, which permits unrestricted use, distribution, and reproduction in any medium, provided the original work is properly cited.
View this paper at Google Scholar | DPI Digital Library
How to Cite this Paper
- IEEE Citation
- MLA Citation
- APA Citation
- BibTex Citation
- RIS Citation
IEEE Style Citation: S. Sankaranarayanan, T. Pramananda Perumal, “Analysis of Naïve Bayes Classification for Diabetes Mellitus,” International Journal of Computer Sciences and Engineering, Vol.6, Issue.12, pp.520-524, 2018.
MLA Style Citation: S. Sankaranarayanan, T. Pramananda Perumal "Analysis of Naïve Bayes Classification for Diabetes Mellitus." International Journal of Computer Sciences and Engineering 6.12 (2018): 520-524.
APA Style Citation: S. Sankaranarayanan, T. Pramananda Perumal, (2018). Analysis of Naïve Bayes Classification for Diabetes Mellitus. International Journal of Computer Sciences and Engineering, 6(12), 520-524.
BibTex Style Citation:
@article{Sankaranarayanan_2018,
author = {S. Sankaranarayanan, T. Pramananda Perumal},
title = {Analysis of Naïve Bayes Classification for Diabetes Mellitus},
journal = {International Journal of Computer Sciences and Engineering},
issue_date = {12 2018},
volume = {6},
Issue = {12},
month = {12},
year = {2018},
issn = {2347-2693},
pages = {520-524},
url = {https://www.ijcseonline.org/full_paper_view.php?paper_id=3372},
doi = {https://doi.org/10.26438/ijcse/v6i12.520524}
publisher = {IJCSE, Indore, INDIA},
}
RIS Style Citation:
TY - JOUR
DO = {https://doi.org/10.26438/ijcse/v6i12.520524}
UR - https://www.ijcseonline.org/full_paper_view.php?paper_id=3372
TI - Analysis of Naïve Bayes Classification for Diabetes Mellitus
T2 - International Journal of Computer Sciences and Engineering
AU - S. Sankaranarayanan, T. Pramananda Perumal
PY - 2018
DA - 2018/12/31
PB - IJCSE, Indore, INDIA
SP - 520-524
IS - 12
VL - 6
SN - 2347-2693
ER -
![]() |
![]() |
![]() |
314 | 191 downloads | 170 downloads |
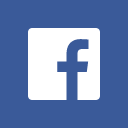
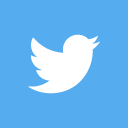
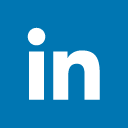
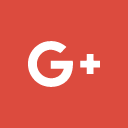
Abstract
Data Mining plays a major role in the decision making process of any application as in Health Care, Artificial Intelligence, military and weather forecasting. In particular, Classification is used to implement the real time Clinical Decision Support System (CDSS) in health care industry. Thus the CDSS can be viewed as if it predicts the decisions through the supervised learning instances from the training dataset. Here a discrete set of algorithms and techniques are in vogue in the backdrop of classification through supervised learning and hence termed as classification algorithms. Among these classification algorithms, Naïve Bayes is the most familiar which uses the historical data as supervised learning instances. This paper surveys the application of Naïve Bayes classification in health care with specific pertinence to analyzing Diabetic Mellitus disease. It also focuses on the implementation of this specific algorithm in the Diabetic domain to expertise an application.
Key-Words / Index Term
Classification, Text Classification, Naïve Bayes, Semantic Analysis, Health Care
References
[1] D. Lewis, ―Naive Bayes at Forty: The Independence Assumption in Information Retrieval‖, Proceedings of the 10th European Conference on Machine Learning(ECML-98), 1998.
[2] Christopher D. Manning, Prabhakar Raghavan and Hinrich Schütze, ―An Introduction to Information Retrieval‖, Cambridge University Press, page 181, 2009.
[3] A. Basu, C. Waters and M.Shepherd, ―Support Vector Machines for Text Categorization‖, Proceedings of the 36th Annual Hawaii International Conference on System Sciences, 2003. For Conference
[4] Gongde Guo, Hui Wang, David Bell, Yaxin Bi and Kieran Greer, ―KNN Model-Based Approach in Classification‖, Proceedings of the ODBASE, pp- 986 – 996, 2003.
[5] Kamal Nigam, John Lafferty and Andrew McCulllum, ―Using Maximum Entropy for Text Classification‖, IJCAI-99, Workshop on Machine learning for Information Filtering, pp. 61-67, 1999.
[6] Stuart, A.; Ord, K. (1994), Kendall`s Advanced Theory of Statistics: Volume I—Distribution Theory, Edward Arnold, §8.7.
[7] Priyanka, Sana Khan, Tulsi Kaur—Investigation on Smart Health Care using Data Mining Methods‖, International Journal of Scientific Research in Computer Sciences and Engineering (IJSRCSE), Vol 4, Issue 2, pp 31-36, 2320–088X, 2016
[8] Ankita R. Borkar and Dr. Prashant R. Deshmukh , ―Naïve Bayes Classifier for Prediction of Swine Flu Disease‖, International Journal of Advanced Research in Computer Science and Software Engineering, Volume 5, Issue 4, pp. 120-123, 2277 128X, 2015.
[9] Ms.Rupali R.Patil, ―Heart Disease Prediction System using Naive Bayes and Jelinek-Mercer smoothing‖, International Journal of Advanced Research in Computer and Communication Engineering, Vol. 3, Issue 5, pp. 6787-6792, : 2278-1021 2014.
[10] Shweta Kharya, Shika Agrawal and Sunita Soni, ―Naive Bayes Classifiers: A Probabilistic Detection Model for Breast Cancer‖, International Journal of Computer Applications (0975 – 8887) Volume 92 – No.10, pp.26-31, 2014 [10] UCI Machine Learning Repository, http://ics.uci.edu/ mlearn/MLRepository.html. 0975 – 8887.
[12] Stephanie J. Hickey, ―Naive Bayes Classification of Public Health Data with Greedy Feature Selection‖, Communications of the IIMA, Volume 13, Issue 2 Article 7, pp. 87-98, 2013.
[13] A.Ambica, Satyanarayana Gandi and Amarendra Kothalanka, ―An Efficient Expert System For Diabetes By Naïve Bayesian Classifier‖, International Journal of Engineering Trends and Technology (IJETT) –Volume 4 Issue 10, pp.4634-4639, 2231-5381 2013
[14] Pablo Gamallo, Marcos Garcia and Santiago Fernández-Lanza, ―TASS: A Naive-Bayes strategy for sentiment analysis on Spanish tweets‖, Workshop on Sentiment Analysis at SEPLN (TASS2013), pp. 126-132, 2013.