Robust and Realistic Classification of Massive Gray Level Thresholding in Remote Sensing Images
T. Balaji1
Section:Research Paper, Product Type: Journal Paper
Volume-2 ,
Issue-11 , Page no. 31-38, Nov-2014
Online published on Nov 30, 2014
Copyright © T. Balaji . This is an open access article distributed under the Creative Commons Attribution License, which permits unrestricted use, distribution, and reproduction in any medium, provided the original work is properly cited.
View this paper at Google Scholar | DPI Digital Library
How to Cite this Paper
- IEEE Citation
- MLA Citation
- APA Citation
- BibTex Citation
- RIS Citation
IEEE Style Citation: T. Balaji , “Robust and Realistic Classification of Massive Gray Level Thresholding in Remote Sensing Images,” International Journal of Computer Sciences and Engineering, Vol.2, Issue.11, pp.31-38, 2014.
MLA Style Citation: T. Balaji "Robust and Realistic Classification of Massive Gray Level Thresholding in Remote Sensing Images." International Journal of Computer Sciences and Engineering 2.11 (2014): 31-38.
APA Style Citation: T. Balaji , (2014). Robust and Realistic Classification of Massive Gray Level Thresholding in Remote Sensing Images. International Journal of Computer Sciences and Engineering, 2(11), 31-38.
BibTex Style Citation:
@article{Balaji_2014,
author = {T. Balaji },
title = {Robust and Realistic Classification of Massive Gray Level Thresholding in Remote Sensing Images},
journal = {International Journal of Computer Sciences and Engineering},
issue_date = {11 2014},
volume = {2},
Issue = {11},
month = {11},
year = {2014},
issn = {2347-2693},
pages = {31-38},
url = {https://www.ijcseonline.org/full_paper_view.php?paper_id=297},
publisher = {IJCSE, Indore, INDIA},
}
RIS Style Citation:
TY - JOUR
UR - https://www.ijcseonline.org/full_paper_view.php?paper_id=297
TI - Robust and Realistic Classification of Massive Gray Level Thresholding in Remote Sensing Images
T2 - International Journal of Computer Sciences and Engineering
AU - T. Balaji
PY - 2014
DA - 2014/11/30
PB - IJCSE, Indore, INDIA
SP - 31-38
IS - 11
VL - 2
SN - 2347-2693
ER -
![]() |
![]() |
![]() |
3658 | 3498 downloads | 3617 downloads |
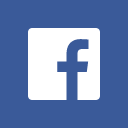
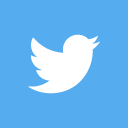
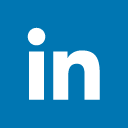
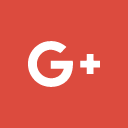
Abstract
Thresholding is an important technique for remote sensing image classification that tries to identify and extract a target from its background on the basis of the distribution of gray levels. Most thresholding techniques are based on the statistics of the one-dimensional histogram of gray levels and the two-dimensional co-occurrence matrix of an image. The remote sensing image classification refers to the process of separating an image into the multi gray level classes or features. The main goal of classification is to simply change the representation of an image into something that is more meaningful and easier to analyze. We present a new algorithm based on robust and realistic classification of massive gray level thresholding representation of remote sensing image. This algorithm allows the distribute the number of gray levels for an image in a fine to grainy fashion, starting with the original gray levels present in the image and all the way down to two gray levels that simply create a binary based version of the original image. This algorithm can also be used to find different gray level threshold image in a natural way without forcing a specific number ahead of time. The accuracy of this algorithm can be most demanding part of a computer vision application. In this work, gray level histogram thresholding is proposed in order to help the classification step to found to be robust way regardless of the classification approach. A proposed method over a remote sensing images have shown that offers very good classification results with a low computation time. Our experimental results show that the thresholding method based on gray level optimization is more efficient than the other classical thresholding methods.
Key-Words / Index Term
RSI, LCS, EBC, RBC, TBT, LTT, GTT and SMGT
References
[1] Balaji T., amd Sumathi M., “Effective Features of Remote Sensing Image Classification Using Interactive Adaptive Thresholding Method”, International Conference Proceedings, ICICA, Coimbatore, pp. 149-153, March 2014.
[2] Balaji T., amd Sumathi M., “A Simple and Efficient Feature Based Image Classification of Remote Sensing Images using Local Threshold Method”, International Conference Proceedings, ENIAC, Kovilpatti, pp. 49-55, January 2014.
[3] Balaji T., amd Sumathi M., “Relational Color Features of Remote Sensing Image Classification Using Dynamic Global Threshold Method”, International Conference Proceedings, ICRSI, Gujarat, pp. 29-34, December 2013.
[4] Balaji T., and Sumathi M., “Remote Sensing Image Classification – A Perspective Analysis”, International Journal of Third Concept, pp. 37-41, September 2013.
[5] D. M. Tsai and Y. H. Chen, “A Fast Histogram Clustering Approach for Multilevel Thresholding”, Pattern Recognition, Vol. 53, No. 24, 2012, pp. 245-252.
[6] N. Otsu, “A Threshold Selection Method from Gray Level Histogram”, IEEE Transactions on System Man Cybernetics, Vol. 19, No. 3, 2012, pp. 62-66.
[7] J. Gong, L. Li, and W. Chen, “Fast Recursive Algorithms for Two-dimensional Thresholding”, Pattern Recognition, Vol. 61, No. 14, 2011, pp. 295-300.
[8] N. Ahuja and A. Rosenfeld, “A Note on the Use of Second-order Gray Level Statistics for Threshold Selection”, IEEE Trans. Vol. 98, 2010, pp.383–388.
[9] B. Chanda and D. D. Majumder, “A Note on the Use of Gray Level Co-occurrence Matrix in Threshold Selection”, Signal Processing, Vol. 55, 2010, pp. 149–167.
[10] A. S. Abutaleb, “Automatic Thresholding of Gray Level Pictures Using Two-dimensional Entropy”, Computer Vision Graphics, Vol. 77, 2010, pp. 122–132.
[11] A. D. Brink, “Gray Level Thresholding of Images Using a Correlation Criterion”, Pattern Recogn. Vol. 99, 2010, pp. 335–341.
[12] Y. J. Zhang, ‘‘A Survey on Evaluation Methods for Image Classification’’, Pattern Recogn. Vol. 129, 2009, pp. 1335–1346.
[13] Otsu. N, “A Thresholding Selection Method from Gray Level Histogram”, IEEE Trans.,Vol. 48, 2009, pp. 62–66.
[14] Kapur, J.N., Sahoo, P.K., and Wong, “A New Method for Gray Level Picture Thresholding Using the Entropy of the Histogram”, Computer Vision Graphics, Vol. 109, 2009, pp. 273–285.