Automatic Tumor Classification of Brain MRI Images
V. Vani1 , M.K. Geetha2
Section:Research Paper, Product Type: Journal Paper
Volume-4 ,
Issue-10 , Page no. 144-151, Oct-2016
Online published on Oct 28, 2016
Copyright © V. Vani, M.K. Geetha . This is an open access article distributed under the Creative Commons Attribution License, which permits unrestricted use, distribution, and reproduction in any medium, provided the original work is properly cited.
View this paper at Google Scholar | DPI Digital Library
How to Cite this Paper
- IEEE Citation
- MLA Citation
- APA Citation
- BibTex Citation
- RIS Citation
IEEE Style Citation: V. Vani, M.K. Geetha , “Automatic Tumor Classification of Brain MRI Images,” International Journal of Computer Sciences and Engineering, Vol.4, Issue.10, pp.144-151, 2016.
MLA Style Citation: V. Vani, M.K. Geetha "Automatic Tumor Classification of Brain MRI Images." International Journal of Computer Sciences and Engineering 4.10 (2016): 144-151.
APA Style Citation: V. Vani, M.K. Geetha , (2016). Automatic Tumor Classification of Brain MRI Images. International Journal of Computer Sciences and Engineering, 4(10), 144-151.
BibTex Style Citation:
@article{Vani_2016,
author = {V. Vani, M.K. Geetha },
title = {Automatic Tumor Classification of Brain MRI Images},
journal = {International Journal of Computer Sciences and Engineering},
issue_date = {10 2016},
volume = {4},
Issue = {10},
month = {10},
year = {2016},
issn = {2347-2693},
pages = {144-151},
url = {https://www.ijcseonline.org/full_paper_view.php?paper_id=1093},
publisher = {IJCSE, Indore, INDIA},
}
RIS Style Citation:
TY - JOUR
UR - https://www.ijcseonline.org/full_paper_view.php?paper_id=1093
TI - Automatic Tumor Classification of Brain MRI Images
T2 - International Journal of Computer Sciences and Engineering
AU - V. Vani, M.K. Geetha
PY - 2016
DA - 2016/10/28
PB - IJCSE, Indore, INDIA
SP - 144-151
IS - 10
VL - 4
SN - 2347-2693
ER -
![]() |
![]() |
![]() |
1598 | 1366 downloads | 1311 downloads |
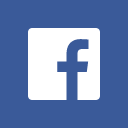
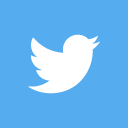
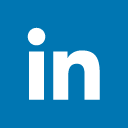
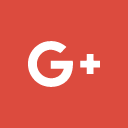
Abstract
Brain tumor classification is an active research area in medical image processing and pattern recognition. Brain tumor is an abnormal mass of tissue in which some cells grow and multiply uncontrollably, apparently unregulated by the mechanisms that control normal cells. The growth of a tumor takes up space within the skull and interferes with normal brain activity. The detection of the tumor is very important in earlier stages. Automating this process is a challenging task because of the high diversity in the appearance of tumor tissues among different patients and in many cases similarity with the normal tissues. This paper depicts a novel framework for brain tumor classification based on Gray Level Co-occurrence matrix (GLCM) statistical features are extracted from the brain MRI images, which signify the important texture features of tumor tissue. The experiments are carried out using BRATS dataset, considering two classes viz (normal and abnormal) and the extracted features are modeled by Support Vector Machines (SVM), k-Nearest Neighbor (k-NN) and Decision Tree(DT)for classifying tumor types. In the experimental results, Decision Tree exhibit effectiveness of the proposed method with an overall accuracy rate of 98.68%, this outperforms the SVM and k-NN classifiers.
Key-Words / Index Term
MRI, GLCM, SVM, K-NN, DT, Brain Tumor, Tumor detection, BRATS
References
[1] Ahmed kharrat, Karim Gasmi, et.al, �A Hybrid Approach for Automatic Classification of Brain MRI Using Genetic Algorithm and Support Vector Machine,� Leonardo Journal of Sciences, pp.71-82, 2010.
[2] Ahmed Kharrat, Mohamed Ben Messaoud, et.al, �Detection of Brain Tumor in Medical Images,� International Conference on Signals, Circuits and Systems IEEE, pp.1-6, 2009.
[3] Priyanka, Balwinder Singh. "A review on brain tumor detection using segmentation." International Journal of Computer Science and Mobile Computing (IJCSMC) 2.7 (2013): 48-54.
[4] Ramteke, R. J., and Y. Khachane Monali. "Automatic medical image classification and abnormality detection using K-Nearest Neighbour." International Journal of Advanced Computer Research 2.4 (2012): 190-196.
[5] Vishnumurthy T D, Mohana H S Vaibhav A Meshram and Pramod Kammar, "Suppression of Herringbone Artifact in MR Images of Brain Using Combined Wavelet and FFT Based Filtering Technique", International Journal of Computer Sciences and Engineering, Volume-04, Issue-02, Page No (66-71), Feb -2016
[6] Azzeddine Riahi, "Image Segmentation Techniques Based on Fuzzy C-Means and Otsu, Applied to the Brain MRI in Tumor Detection", International Journal of Computer Sciences and Engineering, Volume-03, Issue-12, Page No (89-101), Dec -2015
[7] Qurat-Ul-Ain, Ghazanfar Latif, �Classification and Segmentation of Brain Tumor using Texture Analysis,� Recent Advances In Artificial Intelligence, Knowledge Engineering And Data Bases, pp 147-155, 2010.
[8] Vipin Y. Borole, Seema S. Kawathekar, "Study of various DIP Techniques used for Brain Tumor detection and tumor area calculation using MRI images", International Journal of Computer Sciences and Engineering, Volume-04, Issue-07, Page No (39-43), Jul -2016,
[9] G Vijay Kumar and G V Raju, "A Real-Time Approach to Brain Tumor Detection Implementing Wavelets and ANN", International Journal of Computer Sciences and Engineering, Volume-03, Issue-11, Page No (89-93), Nov -2015
[10] Parag P. Bharne and Deepak Kapgate, "A Review of Classification Techniques in Brain Computer Interface", International Journal of Computer Sciences and Engineering, Volume-02, Issue-12, Page No (68-72), Dec -2014.
[11] Menze, Bjoern H., et al. "The multimodal brain tumor image segmentation benchmark (BRATS)." IEEE Transactions on Medical Imaging 34.10 (2015): 1993-2024.
[12] F. Albregtsen, �Statistical texture measures computed from GLCM�, Image processing Laboratory, Dept of Informatics, University of Oslo, 2008.
[13] Nello Cristianini and John Shawe-Taylor, An introduction to support vector machines and other kernel-based learning methods, Cambridge university press, 2000.
[14] Vladimir Naumovich Vapnik and Vlamimir Vapnik, Statistical learning theory, vol. 1, Wiley New York, 1998.
[15] Keller, James M., Michael R. Gray, and James A. Givens. "A fuzzy k-nearest neighbor algorithm." IEEE transactions on systems, man, and cybernetics 4 (1985): 580-585.
[16] N. Bhatia et al, Survey of Nearest Neighbor Techniques. International Journal of Computer Science and Information Security, Vol. 8, No. 2, 2010.
[17] Leo Breiman, Jerome Friedman, Charles J Stone, and Richard A Olshen, Classification and regression trees, CRC press, 1984.
[18] J. Ross Quinlan, �Induction of decision trees,� Machine learning, vol. 1, no. 1, pp. 81�106, 1986.
[19] Geetika Gupta, RupinderKaur, ArunBansal, MunishBans al, �Analysis and Comparison of Brain Tumor Detection and Extraction Techniques from MRI Images.� International Journal of Advanced Research in Electrical,Electronics and Instrumentation Engineering. Vol. 03(11), pp. 13272-13284, Novembe r 2014.
[20] ManojKKowarandSourabhYadav,�BrainTumor Detction and Segmentation Using Histogram Thresholding.�International Journal of Engineering and Advanced Technology. Vol. 01(04), pp. 16-20, April 2012.
[21] D.Selvaraj et.al / Indian Journal of Computer Science and Engineering (IJCSE)� Mri Brain Image Segmentation Techniques - A Review -ISSN : 0976-5166Vol. 4 No.5 Oct-Nov 2013