Recent Advances in Deep Learning Techniques
M.Sornam 1 , E. Panneer Selvam2
Section:Survey Paper, Product Type: Journal Paper
Volume-06 ,
Issue-04 , Page no. 131-135, May-2018
Online published on May 31, 2018
Copyright © M.Sornam, E. Panneer Selvam . This is an open access article distributed under the Creative Commons Attribution License, which permits unrestricted use, distribution, and reproduction in any medium, provided the original work is properly cited.
View this paper at Google Scholar | DPI Digital Library
How to Cite this Paper
- IEEE Citation
- MLA Citation
- APA Citation
- BibTex Citation
- RIS Citation
IEEE Style Citation: M.Sornam, E. Panneer Selvam, “Recent Advances in Deep Learning Techniques,” International Journal of Computer Sciences and Engineering, Vol.06, Issue.04, pp.131-135, 2018.
MLA Style Citation: M.Sornam, E. Panneer Selvam "Recent Advances in Deep Learning Techniques." International Journal of Computer Sciences and Engineering 06.04 (2018): 131-135.
APA Style Citation: M.Sornam, E. Panneer Selvam, (2018). Recent Advances in Deep Learning Techniques. International Journal of Computer Sciences and Engineering, 06(04), 131-135.
BibTex Style Citation:
@article{Selvam_2018,
author = {M.Sornam, E. Panneer Selvam},
title = {Recent Advances in Deep Learning Techniques},
journal = {International Journal of Computer Sciences and Engineering},
issue_date = {5 2018},
volume = {06},
Issue = {04},
month = {5},
year = {2018},
issn = {2347-2693},
pages = {131-135},
url = {https://www.ijcseonline.org/full_spl_paper_view.php?paper_id=368},
publisher = {IJCSE, Indore, INDIA},
}
RIS Style Citation:
TY - JOUR
UR - https://www.ijcseonline.org/full_spl_paper_view.php?paper_id=368
TI - Recent Advances in Deep Learning Techniques
T2 - International Journal of Computer Sciences and Engineering
AU - M.Sornam, E. Panneer Selvam
PY - 2018
DA - 2018/05/31
PB - IJCSE, Indore, INDIA
SP - 131-135
IS - 04
VL - 06
SN - 2347-2693
ER -
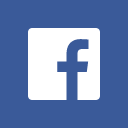
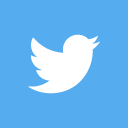
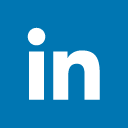
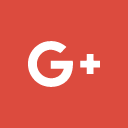
Abstract
Deep Learning is currently being used for a variety of different applications. It has drawn increasing research interest because of the capability of overcoming the drawback of traditional algorithms. Some of the important applications are pattern recognition, computer vision, speech recognition, natural language processing, handwriting recognition, face recognition, IoT and medical. There are several researches has been done in the area of deep learning from the last decade of the nineteenth century and still many more to come. This paper gives survey on deep learning and some of the recent research that has been done in the area of deep learning.
Key-Words / Index Term
Machine Learning, Deep learning, Fog Computing, Genetic Algorithm, Pattern Recognition.
References
[1] Weibo Liu, Zidong Wang, Xiaohui Liu, Nianyin Zeng, Yurong Liu, Fuad E. Alsaadi, “A survey of deep neural network architecture and their applications,” Neurocomputing, vol. 234, pp. 11-26, 2017.
[2] Vrizlynn L.L. Thing, “IEEE 802.11 Network Anomaly Detection: A Deep Learning Approach,” IEEE Wireless Communication and Networking Conference, pp. 1-6, 2017.
[3] AsanthaThilina, Shakthi Atanayake, SacithSamarakoon, DahamiNawodya, LakmalRupasinghe, NadithPathirage, TharinduEdirisinghe, KesavanKrishnadeva, “Intruder Detection using Deep Learning and Association Rule Mining,” IEEE Conference on Computer and Information Technology, pp. 615-620, 2016.
[4] Chuanlong Yin, Yuefei Zhu, Jinlong Fei, Xinzheng He, “A Deep Learning Approach for Intrusion Detection using Recurrent Neural Networks,” IEEE Access, vol.5, pp. 21954-21961, 2017.
[5] Nency Patel, Narendra Sekokar, “Implementation of pattern matching algorithm to defend SQLIA,” Procedia Computer Science, vol.45, pp. 453-459, 2015
[6] Youbiao He, Gihan J, Mendis, Jin Wei, “Real-Time Detection of False Data Injection Attacks in Smart Grid: A Deep Learning-Based Intelligent Mechanism,” IEEE Transaction on Smart Grid, vol. 8, no. 5, pp. 2505-2516, 2017.
[7] Hongmei He, Tim Watson, Carsten Maple, JornMehnen, Ashutosh Tiwari, “A New Semantic Deep Learning with a Linguistic Attribute Hierarchy for Spam Detection,” International Joint Conference on Neural Networks, pp. 3862-3869, 2017.
[8] A.ADiro, N. Chilamkurti, “Distributed Attack Detection Scheme using Deep Learning Approach for Internet of Things,” Future Generation Computer Systems DOI=http”//dx.doi.org/10.1016/j.future.2017.08.043
[9] Dayieng Liu, JianchengLv, Xiaofeng Qi and Jiangshhu Wei, “A Neural Words Encoding Model,” International Joint Conference on Neural Network, pp. 532-536, 2016.
[10] Xiaoguang Chen, Xuan Yang, Maosen Wang, Jiaancheng Zou, “Convolutional Neural Network for Automatic Facial Expression Recognition,” International Conference on Applied System Innovation, pp. 814-817, 2017.
[11] Zijing Mao, Wan Xiang Yao, Yufei Huang, “EEG-based biometric identification with deep learning,” International IEEE/EMBS Conference on Neural Engineering, pp. 609-612, 2017.
[12] Daniele Ravi, Charence Wong, Benny Lo, Guang-Zhong Yang, “A Deep Learning Approach to on-Node Sensor Data Analytics for Mobile or Wearable Devices,” IEEE Journal of Biomedical and Health Informatics, vol. 21, no.1, pp. 56-64, 2017.
[13] Na Lu, Tengei Li, Xiaodong Ren, Hongyu Miao, “A Deep Learning Scheme or Motor Imagery Classification based on Restricted Boltzman Machines,” Transaction on Neural Systems and Rehabilitation Engineering, vol. 25, no. 6, pp. 566-576, 2017.
[14] M. Sornam, C. Vishnu Priya, “Deep Convolutional Neural Network for Handwritten Tamil Character Recognition using Principal Component Analysis,” International Conference on Next Generation Computing Technologies, University of Petroleum and Energy Studies, Dehradun, Oct 30th and 31st 2017, pp. 102-112.
[15] Mohammed Amine JanatiIdrissi, Hassan Ramchoun, Youssef Ghanou, “Genetic Algorithm for Neural Network Architecture Optimization,” International Conference on Logistics Operation Management, pp.1-4, 2017.
[16] YausiKanada, “Optimizing Neural Network Learning Rate by Using a Genetic Algorithm with Per-epoch Mutations,” International Joint Conference on Neural Networks, pp. 1472-1479, 2016.